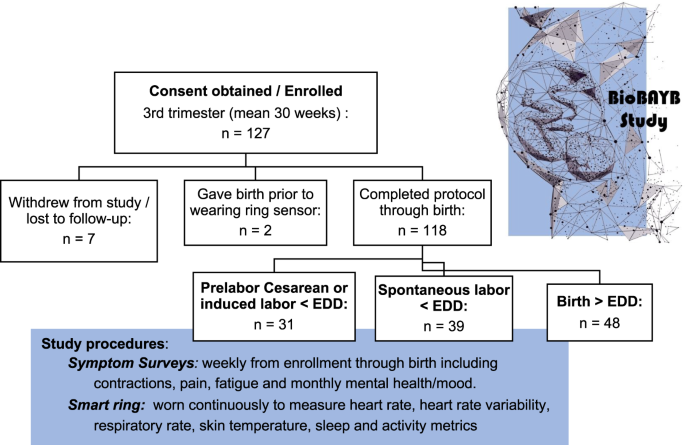
- Select a language for the TTS:
- UK English Female
- UK English Male
- US English Female
- US English Male
- Australian Female
- Australian Male
- Language selected: (auto detect) - EN
Play all audios:
ABSTRACT The transition from pregnancy into parturition is physiologically directed by maternal, fetal and placental tissues. We hypothesize that these processes may be reflected in maternal
physiological metrics. We enrolled pregnant participants in the third-trimester (_n_ = 118) to study continuously worn smart ring devices monitoring heart rate, heart rate variability, skin
temperature, sleep and physical activity from negative temperature coefficient, 3-D accelerometer and infrared photoplethysmography sensors. Weekly surveys assessed labor symptoms, pain,
fatigue and mood. We estimated the association between each metric, gestational age, and the likelihood of a participant’s labor beginning prior to (versus after) the clinical estimated
delivery date (EDD) of 40.0 weeks with mixed effects regression. A boosted random forest was trained on the physiological metrics to predict pregnancies that naturally passed the EDD versus
undergoing onset of labor prior to the EDD. Here we report that many raw sleep, activity, pain, fatigue and labor symptom metrics are correlated with gestational age. As gestational age
advances, pregnant individuals have lower resting heart rate 0.357 beats/minute/week, 0.84 higher heart rate variability (milliseconds) and shorter durations of physical activity and sleep.
Further, random forest predictions determine pregnancies that would pass the EDD with accuracy of 0.71 (area under the receiver operating curve). Self-reported symptoms of labor correlate
with increased gestational age and not with the timing of labor (relative to EDD) or onset of spontaneous labor. The use of maternal smart ring-derived physiological data in the
third-trimester may improve prediction of the natural duration of pregnancy relative to the EDD. SIMILAR CONTENT BEING VIEWED BY OTHERS THE IMPACT OF HEALTHY PREGNANCY ON FEATURES OF HEART
RATE VARIABILITY AND PULSE WAVE MORPHOLOGY DERIVED FROM WRIST-WORN PHOTOPLETHYSMOGRAPHY Article Open access 30 November 2023 PREDICTION OF FETAL RR INTERVALS FROM MATERNAL FACTORS USING
MACHINE LEARNING MODELS Article Open access 13 November 2023 PRETERM BIRTH RISK STRATIFICATION THROUGH LONGITUDINAL HEART RATE AND HRV MONITORING IN DAILY LIFE Article Open access 27 August
2024 INTRODUCTION Only 5% of the nearly 4 million births occurring in the United States each year occur on the Estimated Date of Delivery (EDD), defined as 40 completed weeks from the first
day of the last menstrual period1,2. Term pregnancy, where term labor is expected to begin, spans from 37–42 weeks of gestation and is typically associated with better outcomes than labors
starting prior to the 37th week. The latter stages of pregnancy can be complicated by preeclampsia, problems with inadequate or excessive fetal growth (macrosomia) as well as concerns for
fetal demise3,4. Obstetric care providers and families in their care, therefore, must balance these known risks of ongoing pregnancy with uncertainty and anxiety of when and where labor will
start5,6,7,8. This may be particularly worrisome for those living in rural areas or in expanding ‘maternal care deserts’9 where residents may need to drive hours to appropriate hospital
facilities10. Symptoms signaling labor onset are difficult to precisely define as the experience of labor is highly variable between people11. As clinicians have no reliable way to predict
when labor will begin, labor induction is routinely used in situations where uncertainty with waiting for labor could be risky, for example, living far from a hospital, developing an
obstetric problem, or in cases where there is higher potential for a problem like hypertension or fetal demise occurring. For all of these reasons, nearly one-third (31.4%)12 of labors are
induced; a rate that has more than tripled since 1990 (9.6%)13. However, it has been hypothesized that peripheral measures of autonomic activity, reflecting the neuroendocrine state with
shifts in inflammation14 and steroidogenesis15 in the time preceding labor, may therefore be useful in understanding the shift between pregnancy to labor16. Few studies have examined these
kinds of metrics in humans, though animal literature notes that changes in parasympathetic activity17 and body temperature fluctuate in advance of giving birth to offspring18,19,20,21,22,23.
In this study, we report on data derived from a multi-modal smart ring, worn by pregnant participants on a finger. We describe the change in physiological metrics across advancing
gestation, and present results from a boosted random forest that was trained to predict whether pregnancies will extend beyond the EDD compared to those with a naturally shorter length of
gestation. We present the derived physiological metrics that were most useful in improving prediction accuracy for the random forest. We also describe labor symptom frequencies across
gestation and relative to timing of onset of labor. RESULTS PARTICIPANT CHARACTERISTICS From July 2021 through April 2022, 127 pregnant participants living in the United States enrolled in
the Biological Rhythms Before and After Your Birth (BioBAYB) Study. Six participants withdrew after consenting and one was lost to follow-up. Two participants enrolled and then underwent
onset of labor prior to obtaining or wearing the device. For the remaining 118, the mean (standard deviation) maternal age on enrollment was 32.6 (4.1) years and the gestational age at
enrollment was 30.3 (2.9) weeks (Fig. 1). Nulliparous individuals (never given birth previously) comprised 57.1% (_n_ = 68) of the sample. The mean pre-pregnancy body mass index was 24.0
kg/m2 (SD 4.1). The majority had employer-based health insurance (_n_ = 102) and had at least an undergraduate degree of education (_n_ = 112, 94.6%). All participants reported being
partnered or married. Six participants (4.8%) had a history of prior preterm birth and were enrolled slightly earlier in pregnancy, at 27.0 weeks. Timing of enrollment for the majority of
the sample was intended to limit to those without gestational diabetes, which was tested around 26–28 weeks of gestation. Other baseline health and demographic characteristics are reported
in Table 1. Among participants who completed the after-birth survey, 8 (6.7%) reported developing gestational hypertension or preeclampsia after enrolling in the study. Spontaneous onset of
labor occurred for 70 (58.8%) participants at a mean (standard deviation) gestational age of 39.8 (1.3) weeks and a range of 34.1–41.9 weeks. Only one preterm birth was reported (a twin
gestation) when labor began spontaneously at 34 weeks of gestation. Among individuals who underwent labor induction, seven were performed for pre-labor spontaneous rupture of membranes
(SROM) without a clear indication of labor symptoms or cervical dilation at the time of induction; labor induction occurred prior to the EDD for five of these seven participants. For the
additional 41 labor inductions, nine were attributed to passing the due date (range of 40.1–41.1 weeks). Only four (3.3%) pregnancies ended with a Cesarean prior to labor onset due to
obstetric indications (e.g., breech presentation). Figure 2 denotes the distribution and characterization of the gestational age at delivery and timing as well as the mode of labor onset
relative to the EDD. PHYSIOLOGICAL METRICS Thirty metrics were available for analysis from the smart ring. For activity metrics, these data were available as averages across a 24-hour
period. Sleep metrics represent an average during the sleep period. Waveform data was not available for analysis; thus, the metrics are based on the manufacturer’s algorithms for
transforming or interpreting the data (e.g., sleep state or heart rate variability). The mixed effects regression revealed that a number of the daily physiological metrics acquired from the
ring were significantly related with advancing days of gestation. Results from the regression analysis for each physical activity and sleep metric are shown (Table 2). We then compared the
smart ring derived metrics between the analytic groups: those experiencing labor before the EDD to those whose pregnancies passed the EDD. Figure 3 demonstrates the median and interquartile
range values for a subset of the physiological metrics from 30 weeks to 40 weeks of gestation separated by the primary analytic comparison groups. Table 3 shows the differences between these
groups’ metrics in mixed effects linear regression models, adjusted for gestational age at the time of analysis. The individuals who had labor induction or pre-labor Cesarean birth prior to
the EDD were excluded as the primary research question was focused on the timing of spontaneous labor onset prior to vs. after the EDD and these individuals had a shortened pregnancy due to
medical recommendation. After Bonferroni correction for multiple comparisons, only the medium MET metric appeared to differ across the study period (_p_ < 0.002). That is, those
experiencing labor after the EDD had higher average medium intensity physical activity across the measurement period compared to those who had labor start before the EDD. We examined smart
ring metrics (resting heart rate, temperature change, total sleep, medium activity, RMSSD, average breaths, total caloric expenditure) relative to participant characteristics of
pre-pregnancy BMI, fetal sex, maternal age and parity using mixed effects regression, controlling for gestational age day. We did not find any statistically significant findings using a _p_
< 0.002. PREDICTING DELIVERY AFTER EDD USING PHYSIOLOGICAL METRICS We designed a boosted random forest to predict whether participants in the study would have a longer gestation compared
to those who would labor and deliver prior to the EDD. We included all of the features available from the smart ring (Table 2) and we also included the following clinical and demographic
features: age, BMI, sex of baby (if known during gestation), and nulliparity (participant’s first birth). In addition, we included the gestational age on the day of the physiological metric
as a feature in the model. Participants who delivered via induced labor or pre-labor Cesarean prior to the EDD were excluded. The data set was divided into those participants who gave birth
on or before their EDD following spontaneous labor (_n_ = 39) and those who had a pregnancy pass the EDD (_n_ = 48). The longer pregnancies eventually ended via spontaneous labor (_n_ = 31)
or after labor induction (_n_ = 17). To train the random forest, we used only data collected prior to 40 weeks of gestation. Specifically, we included gestational days 234–275 (data from
33.4–39.3 weeks of gestation) to do the prediction. We further limited the training dataset by excluding metrics in the 4 days prior to the onset of labor or labor induction. For example,
for a person starting labor at 39.0 weeks of gestation (273 days) we included any available data from gestational day 234 to gestational day 269. For those laboring >40 weeks data were
included through day 280 only. Our rationale for this approach was to use only data from several days before the due date excluding data whereby labor symptoms in the 4 days just prior to
labor were present. We performed a greedy search24 to determine the combinations of features achieving the highest prediction accuracy as defined by AUC of the ROC curve. To do the greedy
search, we started with a single-feature predictor and determined which feature yielded the largest AUC. We then sequentially added features and determined which 2-feature combination
yielded the largest AUC. We proceeded with this for 3-feature, 4-feature and up to 33-feature combinations. During the search, we used cross validation to train and evaluate the algorithm
whereby we used 80% of the participants for the training and evaluated the accuracy of the predictor on the 20% of the held-out participants. This was repeated 10 times with a different 20%
of held-out participants each time. The accuracy for each of the 10 folds was stored. Cross validation of the features was repeated five times. Figure 4 shows how the features ranked across
the five runs with the highest ranked features as determined by greedy search are listed from left (best) to right (worst). Each feature’s rank was averaged across the five folds and the
standard deviation of the rank is also shown for each feature across the five folds. Supplementary Fig. 1 shows the individual ranking of features across each of the five runs. While
gestational age was the highest- ranking feature on average, it was not found to be the highest-ranking features on any of the individual folds (Supplementary Fig. 1). When the random forest
was trained only on the gestational age, the performance was significantly worse than when additional physiologic features were included, with a mean sensitivity across 10-fold validation
of 0.55 (specificity = 0.56, AUC = 0.58) (Supplementary Fig. 2). Figure 5 shows the ROC curve for the best feature set of each of the 5 runs using cross-validation data sets. Hyperparameter
tuning was done by cross validation and the final number of trees was found to be 100 with a learning rate of 1.0 and a maximum number of splits of 10. We repeated the greedy search 5 times
to determine whether the ranked features would change order based on the training/testing sets randomly selected. We found that there was some variability in the ranking of the optimal
features, which was most likely due to the fact that many features were highly correlated with each other because they were derived from a small set of sensors on the ring. Regardless,
certain features consistently ranked higher than others and yielded a higher prediction accuracy. Overall, the performance of the model was demonstrated by an AUC of 0.71 and optimal
sensitivity (0.66) and specificity (0.64) based on the harmonic mean (and IQR) across the 5 runs. The best ranked features for predicting if a pregnancy will pass the EDD were the
gestational age at the time the prediction was made, temperature trend values, MET (medium, high activity) and sleep measures (REM-stage sleep, total, and rest). The next best-ranked
features were BMI, sleep onset/midpoint, MET: high, and resting heart rate, temperature change and respiratory rate. Among the least predictive features were sleep efficiency, target
kilometers, average heart rate, daily movement, low activity, active calories, participant age, inactivity, average/low metabolic equivalents, total steps, parity, deep sleep duration, end
of bedtime, total calories, RMSSD, fetal sex and duration restless sleep. MATERNAL SELF-REPORTED LABOR SYMPTOMS ASSOCIATED WITH ADVANCING GESTATIONAL AGE BUT NOT WITH MODE OF LABOR ONSET OR
EVENTUAL DURATION OF PREGNANCY Participants completed weekly labor symptom surveys administered through automated REDCap25 invitations after enrollment. We used mixed effects logistic
regression models, with the participant as the random effect, to estimate the likelihood of labor symptoms being reported with advancing gestation. We found that reports of uterine activity,
vaginal discharge, menstrual-like cramping and low back pain increased as gestation advanced as a whole (main effects) (Table 4). With two exceptions, interaction models did not demonstrate
any significant differences between the group of individuals who experienced spontaneous onset of labor compared to those who did not have spontaneous labor (labor induction or Cesarean
birth without labor). Those who eventually had labor begin spontaneously were 25% more likely to report contractions occurring while at rest and 16% more likely to report vaginal discharge
compared to those who ended up with labor induction or delivery without labor. Both odds ratios were significant using a _p_ < 0.05, which may be spurious findings given the number of
comparisons performed in Table 4. No symptom differences were noted between those with pregnancies passing the EDD versus labor starting before 40 weeks. Clinical and demographic data
including parity, body mass, ethnicity, age, educational attainment, employment, insurance method, or family income did not differ between those who labored prior to their EDD and those with
longer pregnancies (Table 1). Finally, no differences were observed between groups in symptom burden (Fig. 6). In sum, these data demonstrate that self-reported symptoms of labor were
well-correlated with advancing gestational age, but the likelihood of reporting the symptom was not related to labor occurring spontaneously, nor related to longer vs. shorter gestation.
DISCUSSION The purpose of this study was to (1) evaluate how physiological data acquired from a smart ring device during pregnancy are associated with gestational age and (2) evaluate
whether these physiological data can be used to predict the natural length of gestation relative to the clinical due date. Our key findings were first that daily physiological metrics (e.g.,
heart rate, temperature) and sleep and activity measures from the smart ring were statistically related to gestational age, and second that these metrics were useful when used in a random
forest model for predicting which participants were likely to pass their EDD compared to those who would give birth earlier in gestation. We also found that increased self-reported labor
symptoms correlated to advancing gestational age, but were not helpful predicting which participants were likely to pass their EDD compared to those who would give birth earlier in
gestation. Together, these findings indicate that physiological metrics derived from a smart ring worn during pregnancy are most closely mapped to the advancement of the gestation,
particularly given adjustment for multiple comparisons. Comparisons between before/after EDD analytic groups using single metrics were less robust to correction for multiple comparison than
the relationship with gestational age, with only medium MET demonstrating significance. In these data, other clinical and demographic characteristics were not robustly associated with the
physiological data and participants’ symptoms of labor were also unrelated to timing of labor onset. However, the use of multiple physiological metrics in the boosted random forest model
provided for modest prediction of labor beginning before/after the clinical EDD, which was a significant improvement over gestational age alone. Physiological metrics collected by the smart
ring were found to be predictive of timing of birth before or after the EDD. An algorithm that can accurately predict the approximate gestational age of delivery could help to inform
clinical decision-making and personal planning around expectations of labor onset. The prediction accuracy of the algorithm may be improved in the future by utilizing data sampled at a
higher frequency to capture ultradian patterns26,27. A high accuracy algorithm may be useful in planning delivery around term. In cases where balancing uncertainty of risks with ongoing
pregnancy are present (e.g., high blood pressure), which may indicate the need for labor induction, knowledge of the approximate time to the natural start of labor would be valuable for
providers and patients. Some pregnancies will end naturally weeks before others (Fig. 2)—and knowing the time to labor onset would enable opportunities for personalized recommendations.
Forecast of labor timing would also be useful in instances where labor is contraindicated, for example, with placenta previa or certain fetal conditions or if a patient is living a long
distance from the hospital. Our sample was not able to specifically study the prediction of labor starting before term (37 weeks) as we had only one case of spontaneous preterm labor onset
at 34 weeks. Future work in larger samples or more high-risk populations are needed to develop models that predict preterm labor. Studies using a WHOOPTM device and HRV data reported there
was a prominent rise in HRV around the 33rd week of pregnancy28. A preprint using similarly-derived data from WHOOPTM indicated that this inflection was notable around 7 weeks prior to the
onset of labor for term and preterm deliveries29. Our findings are consistent with this observation from the perspective of increased RMSSD with advancing gestational age. While we did not
find RMSSD (heart rate variability) to be a top ranked feature in the boosted random forest model; we did see that RMSSD was related to gestational age in the mixed-effects model. More work
is needed on understanding intergroup as well as inter-individual changes across pregnancy and in relation to delivery across the span of gestation. On average, the most useful physiological
metrics in predicting delivery past the EDD included the temperature trend, the metabolic expenditure in the medium range, the high activity metric, and the time spent in REM and total
sleep. The most predictive physiological metrics in the random forest varied somewhat based on which validation data sets were used (Figs. 4, 5), which was likely due to the fact that many
of the metrics were correlated with each other thus there was not a substantial difference in how these metrics impacted the prediction accuracy. Demographic features including age, fetal
sex, and parity ranked poorly in improving model accuracy. The gestational age when a forecast was being done was one of the more predictive variables on average when assessed alongside the
smart-ring data, however, adding the physiological metrics increased the model’s predictive ability as a whole. More specifically, the prediction based on gestational age alone was little
better than a flip-of-a-coin with and AUC of 0.58. Adding the physiological metrics improved the AUC to 0.71 across the runs. Further, while temperature trends alone were not significantly
related to delivering past the EDD, this metric consistently ranked near the top of features in the random forest. Existing mammalian literature indicate that body temperature changes
precede the onset of labor reliably enough to be used as a metric in animal husbandry or to discern the proximity to giving birth in a variety of species (e.g., cow, dog, horse, moose,
monkey, orca, rabbit, sheep, squirrel, wolverine)18,19,20,21,22,23,30,31,32,33,34,35,36,37,38,39. To our knowledge, this change in temperature as a function of gestational age has not been
thoroughly examined in the human literature40 and would therefore be useful to study in future research utilizing high temporal resolution. Heart rate variability also has been used to study
and predict parturition in pregnant cows23. It is hypothesized that mechanisms governing spontaneous labor in humans could be visible as trends in peripheral physiological output data
because physiological metrics (e.g. temperature, heart rate variability) are correlated with changes in inflammation, neuroendocrine or autonomic activity16. The transition from pregnancy
into labor results from alterations in numerous hormones, gene/protein expression and reproductive tissue modifications14,15,41,42. Briefly, placental progesterone production (which
maintains uterine quiescence) is progressively subdued by greater placental/fetal corticotropin releasing hormone (CRH) production as well as estriol dominance43. The rise of estriol is
partly dependent upon the fetus’ nervous system and adrenal gland maturation, which leads to greater dehydroepiandrosterone sulfate (DHEA-S) production44. As such, the fetal production of
CRH not only contributes to lung maturation through surfactant production, but also impacts the timing of labor onset45. Estriol and CRH, as well as inflammatory changes, feed-forward
transforming maternal uterine and cervical tissues in preparation for labor14,46. Progesterone is a respiratory47 and body temperature stimulant48,49,50, and higher progesterone is
associated with higher heart rate and lower heart rate variability28,51,52,53,54. Therefore, the functional progesterone withdrawal55 in the lead-up to labor onset could potentially be
apparent by tracking peripheral metrics, such as respiration, temperature and heart rate. In addition to these mechanisms, studies with non-human mammalian models also show that the
autonomic nervous system (ANS) contributes to labor onset and progress and can therefore be inhibited or stalled by experiences that trigger sympathetic dominance17,56. Heart rate
variability is a commonly utilized measure of the parasympathetic/sympathetic balance of the ANS56,57, which could be monitored non-invasively with wearable sensors23,28,58. Current clinical
methods for counseling pregnant individuals on how and when to prepare for labor onset involve considering the current date relative to the EDD, monitoring symptoms, cervical examination
(digital or via ultrasound) and general advice based on population-based data from non-specific demographic characteristics (e.g., first pregnancies tend to be a little longer than
subsequent pregnancies). Conventional and clinical advice centers on monitoring symptom patterns in anticipation of when labor might be beginning. However, symptoms are typically most useful
to diagnose labor in the moment, despite being highly variable from person to person11,59. In our study, weekly self-reported symptoms did not vary by timing of labor relative to the EDD or
whether labor was spontaneous vs. induced. This finding underscores the difficulty (or flawed assumptions) in using symptoms to help guide prediction of future labor onset in personal or
clinical decision making. This observation may be limited by our use of a weekly sampling method. Daily questionnaires may improve observations of subtle changes in labor-related symptoms,
though individual burden and attrition may increase as well. Interestingly, in our sample, few demographic or clinical features were associated with a pregnancy passing the EDD compared to
laboring spontaneously before the due date. Our enrollment criteria for the study (generally healthy, low-risk for complications in pregnancy) likely played a role limiting our ability to
detect differences in pregnancy complications through the smart ring metrics. In addition, the homogeneity in the sample in terms of educational background and income also likely influenced
these null findings. Of the self-reported ethnicity/ancestry, the majority of individuals who identified as Hispanic/Latina were among those giving birth spontaneously prior to the EDD (_p_
< 0.05) (_n_ = 7 of 10 Hispanic participants), though, overall, the sample was mostly made up of those reporting European ancestry/White identity. Repeating this study in a larger, more
diverse sample or among those with higher-risk obstetric histories would be valuable. Several studies have utilized a variety of clinical60,61 or ultrasound derived measures62,63,64 to help
predict the future spontaneous onset of labor, while others have used multi-omics data gathered from blood samples across gestation65. Clinical use of the fetal fibronectin test is currently
used to predict preterm birth (prior to 37 weeks) in high-risk patients, typically presenting with risk factors or labor symptoms. This vaginal swab test used for screening has a
sensitivity of 43–92% and specificity of 52–93% in high-risk patients66. However, fetal fibronectin has no proven utility in predicting term labor onset. Each of these approaches/tools has a
similar objective, to forecast the natural expected length of the pregnancy; however, each method cited above performs with varying degrees of accuracy and requires patients to undergo
specific procedures or tests performed at the clinic. A method using maternal remote physiological monitoring would offer the opportunity to evaluate the likelihood of labor starting using
non-invasive tools in the person’s home environment. Other approaches for wearable non-invasive labor prediction have included use of electrohysterography (measuring electrical activity from
uterine muscle) with or without maternal heart rate data67,68, which appear to characterize the early stages of labor itself and may have particular utility in signaling preterm labor when
overt symptoms are not perceived. One of the limitations of the study is that it assessed proprietary metrics acquired from a commercial smart ring. The accuracy of the metrics could not be
independently validated by our team; however, there have been prior publications demonstrating the accuracy of its calculation of heart rate variability69 and skin temperature70. Many of the
metrics are derived from a small set of sensors, which means that they are correlated with each other. Another potential limitation for interpreting the sensor data is related to
differences in physical activity between groups in which the individuals’ work/home life patterns or exercise routines differ, as these activity habits potentially mediate patterns in other
metrics (i.e., heart rate or respiratory rate). Future work can elucidate these differences using experimental or statistical methods. Replication of this approach in another independent
physiological dataset gathered in pregnancy is needed to validate these findings. Given our enrollment period was largely limited to the third trimester, future studies should aim for
monitoring for a greater proportion of the entire pregnancy. These data will help researchers discern a larger baseline, examine trends across each trimester, and determine the minimum
number of days/weeks of data necessary to obtain reliable predictions on the timing for labor onset. We also note the limitations in generalizability, given the sample was largely
self-identified as White and a majority had at least a college-level of education. Despite the limitations, the strengths of the investigation include use of a device that was relatively
easy/comfortable for the participants to wear; only two participants withdrew from the study because of device discomfort issues. This resulted in high wear time and limited missing data.
Our findings are augmented by the use of weekly survey data on labor symptoms to compare to the physiological metric utility. The use of a predictive training/testing methodology allows the
data to forecast clinical utility of this kind of physiological data driven approach to labor prediction. Another strength is the use of data gathered prior to the EDD (and several days in
advance of labor) which, if replicable or improved upon, could offer a window of time for a person to make important decisions and for their care providers to offer more personalized
recommendations or consider alternative testing/treatment if labor was unlikely to begin prior to the EDD. We demonstrate how multi-modal data derived from a commercially available wearable
smart ring device is associated with the maternal physiological state across gestation and how these data can be used to help predict whether birth may occur before or after the clinical
Estimated Delivery Date. METHODS STUDY OVERSIGHT The institutional review board for Oregon Health and Science University (Study #20059) reviewed and approved the protocol for this study.
This research was carried out in accordance with the Declaration of Helsinki. PARTICIPANTS Participant recruitment strategies included social media advertising across the United States as
well as posted paper and digital announcements in the metro Portland, Oregon region. Inclusion criteria included: adults (at least 18 years of age), able to provide written (e-consent model)
informed consent71 in English who were having a generally healthy pregnancy, at least 26 weeks of gestation (after gestational diabetes testing), had no contraindication for vaginal birth
and pre-pregnancy body mass index of less than 40 kg/m2. Exclusion for enrollment included: current gestational diabetes, hypertension or uncontrolled thyroid disorders, plans to undergo
unlabored Cesarean or to induce labor prior to 41 weeks of gestation, history of ovulatory dysfunction or use of assisted reproductive technology (e.g., in vitro fertilization) for current
pregnancy, working rotating or night shifts. A smaller group of participants were enrolled prior to 26 weeks’ gestation if they had a history of prior preterm birth or current twin gestation
and the participant otherwise met inclusion criteria. STUDY DESIGN AND PROCEDURES This study used a prospective observational design. After enrollment, participants completed an online
survey including demographic data, health/pregnancy history, social determinants of health and an array of psychometric and symptomatology surveys. Surveys included questions on stress
(Perceived Stress Scale), sleep (PROMIS® Sleep Related Impairment—SF), sleep patterns (Munich Chronotype Questionnaire), occupational strains, depression and anxiety scores (Edinburgh
Postnatal Depression and Generalized Anxiety Disorder-7), Antenatal Attachment Scale, adverse childhood experiences (ACES), social support (MOS), fatigue (PROMIS®-SF), physical symptoms/pain
(PHQ-15), emotional support (PROMIS®-SF) and self-efficacy (PROMIS®-SF). A weekly survey of labor symptoms was sent to participants and asked the respondent to indicate (Yes/No) if they had
experienced any of the following across the last seven days: different patterns of contractions (irregular, regular, contractions while at rest, painful, waking at night with contractions,
and/or with movement), low back pain, vaginal discharge or menstrual-like cramping. We also repeated the PROMIS®-SF scales on emotional support, self-efficacy, sleep and fatigue weekly.
After baseline, mood and anxiety scores were assessed monthly throughout the study period (GAD-7 and EPDS). Participants were sent a ring-fitting kit made by the manufacturer (Oura, Finland)
which contains eight different ring sizes and were instructed to wear the best-fitting ring for 24 h to ensure comfortable fit and ability to remove it after sleep. Each participant was
provided a dummy-coded email address for signing up and syncing their ring with the smartphone application. Data would sync and upload to a cloud-based platform upon opening the Oura App. In
the event the app was not opened, the ring would store several days’ worth of data. Rings were charged as needed, typically lasting several days before the application prompted the
participant to charge the ring. Follow-up surveys were sent either weekly or monthly depending on the questionnaire until birth occurred. Upon giving birth, participants notified the study
staff via email. A birth experience survey was sent and any clarifying questions were asked by staff via email, when needed. Self-reported outcomes included any pregnancy associated
conditions (gestational hypertension, preeclampsia, abnormally low amniotic fluid, or growth restriction etc.). Participants also reported if they experienced labor prior to delivery (versus
Cesarean birth before labor began), the date of delivery, mode of birth (vaginal, Cesarean or instrument-assisted vaginal birth), and their newborn(s)’ weight(s). For those who experienced
labor, participants reported if the labor began spontaneously versus a labor induction via pharmaceutical (e.g., oxytocin, prostaglandin) or mechanical methods (e.g., artificial rupture of
membranes). SMART RING DATA The Oura Ring is a commercial health tracking device worn on the finger. The Gen2 Oura Ring is equipped with temperature (negative temperature coefficient (NTC)),
3-D accelerometer, and infrared photoplethysmography (PPG) sensors and measures physiological signals, such as heart rate (HR), heart rate variability (HRV), temperature trends,
respiration, and movement. The sensors are located in the inner part of the ring on the palm side of the finger and the ring is water resistant up to 100 m. Data is transmitted from the ring
to the user’s phone via Bluetooth, and from the phone it is uploaded to the cloud. Users can view their physiological measurements and insights in the Oura App. Participants wore an Oura
Ring throughout the study on whichever finger that they could achieve the best fit on the non-dominant hand. The continuous data collection enables the establishment of personalized
biometric baselines for each user. Thirty features from the wearable-derived data across gestational age were available from the manufacturer of the smart ring including the following:
activity (inactive, rest, low, medium, high, steps, total daily movement), metabolic equivalents (MET) (average, low, medium, high, inactive), calories (active, total), heart rate (average,
resting), HRV (root mean square of successive differences (RMSSD)), sleep (start, onset, midpoint, end, efficiency, total, restless, deep, rapid-eye-movement (REM)), average breaths per
minute, temperature deviation (weighted average across days) and delta from prior day, and non-wear time. OUTCOMES Outcomes were assessed across the sample relative to gestational age and
then between two groups. The first group was made of participants experiencing the onset of spontaneous labor at or before the EDD, and was compared to the second group, consisting of
participants whose pregnancies lasted more than 40 weeks. This dichotomy was chosen as the pregnancies passing the EDD would all have hypothetically started labor eventually if intervention
had not been undertaken for another indication. The EDD was reported by the participant and we also recorded the manner in which the EDD was determined (using the last menstrual period or
ultrasound). Labor and birth times were self-reported. First, we examine the relationship between gestational age at the time of measurement with each smart ring metric followed by a
comparison of the physiological metrics between the groups. Then we report the labor-related symptoms, symptom burden, and pain, fatigue and mood scores in relation to shorter versus longer
gestation. STATISTICAL ANALYSES Descriptive baseline data and self-reported birth outcomes were compared between groups having spontaneous labor onset prior to 40 weeks’ gestation versus a
longer pregnancy using bivariate statistics (parametric or non-parametric as appropriate). In assessing whether the physiological metrics varied with gestational age we performed a mixed
effects linear regression analysis whereby gestational age in days was the independent variable used to predict each physiological metric when controlling for body mass index (BMI), age of
the mother at the start of pregnancy, parity (number of prior births), and sex of the baby if known during pregnancy. Given that some of the metrics were correlated with each other due to
the fact that they were derived from a minimal set of sensors, we used a Bonferroni adjustment to divide _p_ = 0.05 by the 30 features evaluated with gestational age, to obtain a
significance level of _p_ < 0.001667 (_p_ < 0.002). We also measured differences in the physiological metrics relative to participant characteristics (BMI, parity, maternal age or
fetal sex). Using mixed effects logistic regression, we compared presence/absence of individual symptoms of labor in relationship to advancing gestational age, again using an interaction
term for spontaneous labor onset compared to labor that was induced. Next, we tested an interaction term between groups of participants with labor starting before the EDD versus those with
longer gestations. Measures of mental health (mood, depression/anxiety), fatigue were compared against gestational age with mixed effects linear regression and interactions. We also examined
a weekly symptom burden as the sum of the number of symptoms reported by each participant during each week of gestation and compared the symptom burden at each week from 32–40 weeks between
those who experienced induced birth prior to the EDD compared with those who had labor begin spontaneously or those with a longer pregnancy with a Poisson regression model. PREDICTIVE MODEL
A boosted random forest (Adaboost1) was trained using 10-fold cross validation whereby 80% of the participants were included in the training of the model and for hyperparameter tuning, and
20% were used for testing the accuracy in the test set. The objective was to determine which combination of features yielded the highest cross-validation accuracy on the test set. This was
done using a greedy search of optimal features by starting with a single feature and determining the most accurate predictor based on the area under the curve (AUC) of the receiver operative
characteristic (ROC) curve. After the first optimal feature was identified, that feature was combined with each additional feature to determine the best combination of two features that
would yield the highest AUC. This process was repeated across all of the features. REPORTING SUMMARY Further information on research design is available in the Nature Research Reporting
Summary linked to this article. DATA AVAILABILITY Data gathered in this investigation are subject to data use agreements with parties involved in the study and are therefore not freely
available. CODE AVAILABILITY Code will be made available upon request to the contact author. No custom code was generated for these analyses. REFERENCES * Vos, S. C., Anthony, K. E. &
O’Hair, H. D. Constructing the uncertainty of due dates. _Health Commun._ 29, 866–876 (2014). PubMed Google Scholar * Martin, J. A., Hamilton, B. E., Osterman, M. J., Driscoll, A. K. &
Matthews, T. J. National Vital Statistics Reports, 66, 1–70 (2017). * American College of Obstetricians and Gynecologists. Management of late-term and postterm pregnancies. _Obstet.
Gynecol._ 124, 390–396 (2014). Google Scholar * Kortekaas, J. C. et al. Risk of adverse pregnancy outcomes of late- and postterm pregnancies in advanced maternal age: a national cohort
study. _Acta Obstetricia et Gynecologica Scandinavica_ 1022–1030 https://doi.org/10.1111/aogs.13828 (2020). * Eri, T. S., Blystad, A., Gjengedal, E. & Blaaka, G. ‘The waiting mode’:
first-time mothers’ experiences of waiting for labour onset. _Sex. Reprod. Healthc._ 1, 169–173 (2010). PubMed Google Scholar * Henderson, J. & Redshaw, M. Worries about labor and
birth: a population-based study of outcomes for young primiparous women. _Birth_ 43, 151–158 (2016). PubMed Google Scholar * Borrelli, S. E., Walsh, D. & Spiby, H. First-time mothers’
expectations of the unknown territory of childbirth: uncertainties, coping strategies and ‘going with the flow’. _Midwifery_ 63, 39–45 (2018). PubMed Google Scholar * Wessberg A, Lundgren
I, Elden H. Being in limbo: Women’s lived experiences of pregnancy at 41 weeks of gestation and beyond - A phenomenological study. BMC Pregnancy and Childbirth. 17,
https://doi.org/10.1186/s12884-017-1342-4 (2017) * Sonenberg, A. & Mason, D. J. Maternity care deserts in the US. _JAMA Health Forum_ 4, e225541 (2023). PubMed Google Scholar *
Pearson, J., Siebert, K., Carlson, S. & Ratner, N. Patient perspectives on loss of local obstetrical services in rural northern Minnesota. _Birth_ 45, 286–294 (2018). PubMed Google
Scholar * Hanley, G. E. et al. Diagnosing onset of labor: a systematic review of definitions in the research literature. _BMC Pregnancy Childbirth_ 16, 71 (2016). PubMed PubMed Central
Google Scholar * Osterman, M., Hamilton, B., Martin, J. A., Driscoll, A. K. & Valenzuela, C. P. Births: final data for 2020. _Natl Vital-. Stat. Rep._ 70, 1–50 (2021). PubMed Google
Scholar * Osterman MJK, Martin JA. Recent Declines in Induction of Labor by Gestational Age Data from the Natality Data File, National Vital Statistics System. (2014). * Menon, R., Bonney,
E. A., Condon, J., Mesiano, S. & Taylor, R. N. Novel concepts on pregnancy clocks and alarms: redundancy and synergy in human parturition. _Hum. Reprod. Update_ 22, 535–560 (2016). CAS
PubMed PubMed Central Google Scholar * Vannuccini, S., Bocchi, C., Severi, F. M., Challis, J. R. & Petraglia, F. Endocrinology of human parturition. _Ann. d’Endocrinologie_ 77,
105–113 (2016). Google Scholar * Grant, A. D. & Erickson, E. N. Birth, love, and fear: physiological networks from pregnancy to parenthood. _Compr. Psychoneuroendocrinol._ 11, 100138
(2022). PubMed PubMed Central Google Scholar * Nagel, C., Aurich, C. & Aurich, J. Stress effects on the regulation of parturition in different domestic animal species. _Anim. Reprod.
Sci._ 207, 153–161 (2019). PubMed Google Scholar * Græsli, A. R. et al. Body temperature patterns during pregnancy and parturition in moose. _J. Therm. Biol._ 109, 103334 (2022). PubMed
Google Scholar * Nabenishi, H. & Yamazaki, A. Decrease in body surface temperature before parturition in ewes. _J. Reprod. Dev._ 63, 185–190 (2017). PubMed PubMed Central Google
Scholar * Katsumata, E. et al. Body temperature and circulating progesterone levels before and after parturition in killer whales (Orcinus orca). _J. Reprod. Dev._ 52, 65–71 (2006). CAS
PubMed Google Scholar * Ricci, A. et al. Assessment of the temperature cut-off point by a commercial intravaginal device to predict parturition in Piedmontese beef cows. _Theriogenology_
113, 27–33 (2018). CAS PubMed Google Scholar * McFarland, R. et al. The thermal consequences of primate birth hour and its evolutionary implications. _Biol. Lett._ 18, 20210574 (2022).
PubMed PubMed Central Google Scholar * Kovács, L. et al. Heart rate and heart rate variability in multiparous dairy cows with unassisted calvings in the periparturient period. _Physiol.
Behav._ 139, 281–289 (2015). PubMed Google Scholar * Whitney, A. W. A direct method of nonparametric measurement selection. _IEEE Trans. Comput. C.–_ 20, 1100–1103 (1971). Google Scholar
* Harris, P. A. et al. Research electronic data capture (REDCap)—a metadata-driven methodology and workflow process for providing translational research informatics support. _J. Biomed.
Inform._ 42, 377–381 (2009). PubMed Google Scholar * Grant, A. D., Newman, M. & Kriegsfeld, L. J. Ultradian rhythms in heart rate variability and distal body temperature anticipate the
luteinizing hormone surge onset. _bioRxiv_ 2020.07.15.205450-2020.07.15.205450. https://doi.org/10.1101/2020.07.15.205450 (2020). * Shannahoff-Khalsa, D. S., Kennedy, B., Yates, F. E. &
Ziegler, M. G. Ultradian rhythms of autonomic, cardiovascular, and neuroendocrine systems are related in humans. _Am. J. Physiol._ 270, R873–887 (1996). CAS PubMed Google Scholar *
Rowan, S. P., Lilly, C. L., Claydon, E. A., Wallace, J. & Merryman, K. Monitoring one heart to help two: heart rate variability and resting heart rate using wearable technology in active
women across the perinatal period. _BMC Pregnancy Childbirth_ 22, 887 (2022). PubMed PubMed Central Google Scholar * Jasinski, S. R., Rowan, S., Presby, D. M., Claydon, E. A. &
Capodilupo, E. R. Wearable-derived maternal heart rate variability as a novel digital biomarker of preterm birth. 2022.11.04.22281959. Preprint at https://doi.org/10.1101/2022.11.04.22281959
(2022). * Cooper-Prado, M. J., Long, N. M., Wright, E. C., Goad, C. L. & Wettemann, R. P. Relationship of ruminal temperature with parturition and estrus of beef cows1. _J. Anim. Sci._
89, 1020–1027 (2011). CAS PubMed Google Scholar * Crociati, M., Sylla, L., De Vincenzi, A., Stradaioli, G. & Monaci, M. How to predict parturition in cattle? A literature review of
automatic devices and technologies for remote monitoring and calving prediction. _Anim. (Basel)_ 12, 405 (2022). Google Scholar * Friebe, A. et al. Factors affecting date of implantation,
parturition, and den entry estimated from activity and body temperature in free-ranging brown bears. _PLoS ONE_ 9, e101410–e101410 (2014). PubMed PubMed Central Google Scholar * Geiser,
B., Burfeind, O., Heuwieser, W. & Arlt, S. Prediction of parturition in bitches utilizing continuous vaginal temperature measurement. _Reprod. Domest. Anim._ 49, 109–114 (2014). CAS
PubMed Google Scholar * Higaki, S. et al. Prediction of 24-h and 6-h periods before calving using a multimodal tail-attached device equipped with a thermistor and 3-axis accelerometer
through supervised machine learning. _Anim. (Basel)_ 12, 2095 (2022). Google Scholar * Korosue, K. et al. Assessment for predicting parturition in mares based on prepartum temperature
changes using a digital rectal thermometer and microchip transponder thermometry device. _J. Vet. Med. Sci._ 74, 845–50 (2012). PubMed Google Scholar * Ruppenthal, G. C. & Goodlin, B.
L. Monitoring temperature of pigtailed macaques (Macaca nemestrina) during pregnancy and parturition. _Am. J. Obstet. Gynecol._ 143, 971–3 (1982). CAS PubMed Google Scholar * Smarr, B.
L., Zucker, I. & Kriegsfeld, L. J. Detection of successful and unsuccessful pregnancies in mice within hours of pairing through frequency analysis of high temporal resolution core body
temperature data. _PLoS ONE_ 11, e0160127 (2016). PubMed PubMed Central Google Scholar * Suarez-Trujillo, A. et al. Effect of circadian system disruption on the concentration and daily
oscillations of cortisol, progesterone, melatonin, serotonin, growth hormone, and core body temperature in periparturient dairy cattle. _J. Dairy Sci._ 105, 2651–2668 (2022). CAS PubMed
Google Scholar * Wright, E. C., Boehmer, B. H., Cooper-Prado, M. J., Bailey, C. L. & Wettemann, R. P. Effect of elevated ambient temperature at parturition on duration of gestation,
ruminal temperature, and endocrine function of fall-calving beef cows1. _J. Anim. Sci._ 92, 4449–4456 (2014). CAS PubMed Google Scholar * Yewlett, H. M. Temperature change with impending
labour. _Lancet (Lond., Engl.)_ 2, 106–106 (1979). CAS Google Scholar * Aguilar, H. N. & Mitchell, B. F. Physiological pathways and molecular mechanisms regulating uterine
contractility. _Hum. Reprod. Update_ 16, 725–744 (2010). CAS PubMed Google Scholar * Ghaemi, M. S. et al. Multiomics modeling of the immunome, transcriptome, microbiome, proteome and
metabolome adaptations during human pregnancy. _Bioinformatics_ 35, 95–103 (2019). CAS PubMed Google Scholar * Lopez Bernal, A., Rivera, J., Europe-Finner, G., Phaneuf, S. & Asboth,
G. Parturition: activation of stimulatory pathways or loss of uterine quiescence? _Adv. Exp. Med. Biol._ 395, 435–451 (1995). CAS PubMed Google Scholar * Özdemir, B. G., Özdemir, H. &
Atalay, C. R. The importance of fetal adrenal gland volume measurement in successful labor induction with oxytocin. _J. Obstet. Gynaecol. Res._ https://doi.org/10.1111/jog.15361 (2022). *
Reinl, E. L. & England, S. K. Fetal-to-maternal signaling to initiate parturition. _J. Clin. Invest._ 125, 2569–2571 (2015). PubMed PubMed Central Google Scholar * Snegovskikh, V.,
Park, J. S. & Norwitz, E. R. Endocrinology of parturition. _Endocrinol. Metab. Clin. North Am._ 35, 173–191 (2006). CAS PubMed Google Scholar * LoMauro, A. & Aliverti, A.
Respiratory physiology of pregnancy. _Breathe (Sheff.)_ 11, 297–301 (2015). PubMed Google Scholar * Charkoudian, N. & Stachenfeld, N. Sex hormone effects on autonomic mechanisms of
thermoregulation in humans. _Auton. Neurosci._ 196, 75–80 (2016). CAS PubMed Google Scholar * Buxton, C. L. & Atkinson, W. B. Hormonal factors involved in the regulation of basal body
temperature during the menstrual cycle and pregnancy. _J. Clin. Endocrinol. Metab._ 8, 544–549 (1948). CAS PubMed Google Scholar * Burt, C. C. Peripheral skin temperature in normal
pregnancy. _Lancet_ 254, 787–790 (1949). Google Scholar * Charkoudian, N., Hart, E. C. J., Barnes, J. N. & Joyner, M. J. Autonomic control of body temperature and blood pressure:
influences of female sex hormones. _Clin. Auton. Res._ 27, 149–155 (2017). PubMed Google Scholar * Carpenter, R. E. et al. Changes in heart rate variability and QT variability during the
first trimester of pregnancy. _Physiol. Meas._ 36, 531–545 (2015). CAS PubMed Google Scholar * Al-Shafei, A. I. et al. Heart rate variability and hematological parameters in pregnant
women. _J. Clin. Lab Anal._ 34, e23250 (2020). CAS PubMed PubMed Central Google Scholar * Sarhaddi, F. et al. Trends in heart rate and heart rate variability during pregnancy and the
3-month postpartum period: continuous monitoring in a free-living context. _JMIR Mhealth Uhealth_ 10, e33458 (2022). PubMed PubMed Central Google Scholar * Nadeem, L. et al. Molecular
evidence of functional progesterone withdrawal in human myometrium. _Nat. Commun._ 7, 11565–11565 (2016). CAS PubMed PubMed Central Google Scholar * Nagel, C. et al. Parturition in
horses is dominated by parasympathetic activity of the autonomous nervous system. _Theriogenology_ 82, 160–168 (2014). CAS PubMed Google Scholar * Washio, H., Takeshita, D. & Sakata,
S. Parasympathetic nervous activity is associated with oxytocin in multiparous, but not primiparous, women during the perinatal period. _Clin. Exp. Pharm. Physiol._ 47, 955–965 (2020). CAS
Google Scholar * Grant, A. D., Newman, M. & Kriegsfeld, L. J. Ultradian rhythms in heart rate variability and distal body temperature anticipate onset of the luteinizing hormone surge.
_Sci. Rep._ 10, 1–12 (2020). Google Scholar * Gross, M. M., Haunschild, T., Stoexen, T., Methner, V. & Guenter, H. H. Women’s recognition of the spontaneous onset of labor. _Birth_ 30,
267–271 (2003). PubMed Google Scholar * De Silva, D. A. et al. Timing of delivery in a high-risk obstetric population: a clinical prediction model. _BMC Pregnancy Childbirth_ 17, 202
(2017). PubMed PubMed Central Google Scholar * Panelli, D. M. et al. Using cervical dilation to predict labor onset: a tool for elective labor induction counseling. _Am. J. Perinatol._
36, 1485–1491 (2019). PubMed Google Scholar * Vankayalapati, P. et al. Ultrasound assessment of cervical length in prolonged pregnancy: prediction of spontaneous onset of labor and
successful vaginal delivery. _Ultrasound Obstet. Gynecol._ 31, 328–331 (2008). CAS PubMed Google Scholar * Tanir, H., Sener, T. & Yildiz, Z. Digital and transvaginal ultrasound
cervical assessment for prediction of successful labor induction. _Int. J. Gynecol. Obstet._ 100, 52–55 (2008). CAS Google Scholar * Peng, F. et al. Using transperineal ultrasound to
predict labor onset. _Ann. Transl. Med._ 7, 718 (2019). PubMed PubMed Central Google Scholar * Stelzer, IA. et al. Integrated trajectories of the maternal metabolome, proteome, and
immunome predict labor onset. _Science Translational Medicine_ 13, eabd9898 (2021). CAS PubMed PubMed Central Google Scholar * Daskalakis, G. J., Papantoniou, N. E., Koutsodimas, N. B.,
Papapanagiotou, A. & Antsaklis, A. J. Fetal fibronectin as a predictor of preterm birth. _J. Obstet. Gynaecol._ 20, 347–353 (2000). CAS PubMed Google Scholar * Altini, M., Rossetti,
E., Rooijakkers, M. J. & Penders, J. Towards Non-invasive Labour Detection: A Free-Living Evaluation. in _2018 40th Annual International Conference of the IEEE Engineering in Medicine
and Biology Society (EMBC)_ 2841–2844. https://doi.org/10.1109/EMBC.2018.8512964 (2018). * Altini, M. et al. Combining electrohysterography and heart rate data to detect labour. in _2017
IEEE EMBS International Conference on Biomedical & Health Informatics (BHI)_ 149–152. https://doi.org/10.1109/BHI.2017.7897227 (2017). * Cao, R. et al. Accuracy assessment of oura ring
nocturnal heart rate and heart rate variability in comparison with electrocardiography in time and frequency domains: comprehensive analysis. _J. Med. Internet Res._ 24, e27487 (2022).
PubMed PubMed Central Google Scholar * Maijala, A., Kinnunen, H., Koskimäki, H., Jämsä, T. & Kangas, M. Nocturnal finger skin temperature in menstrual cycle tracking: ambulatory pilot
study using a wearable {Oura} ring. _BMC Women’s Health_ 19, 150–150 (2019). PubMed PubMed Central Google Scholar * Chen, C. et al. Evaluation of a REDCap-based workflow for supporting
federal guidance for electronic informed consent. _AMIA Jt Summits Transl. Sci. Proc._ 2019, 163–172 (2019). PubMed PubMed Central Google Scholar Download references ACKNOWLEDGEMENTS We
are grateful for the contributions of the participants to this research. We also acknowledge research assistance from Sage Fanucchi-Funes, DNP, CNM. Dr. Erickson received an award from the
Oregon Health and Science University (OHSU)/ Oregon Clinical Translational Research Institute - Biomedical Innovations Program to conduct this investigation as well as funding from OHSU
School of Nursing Foundation Innovation Award. We also acknowledge the support of Ouraring Inc., in particular, for its contribution of devices and data access provided to the investigators
under a data use agreement with OHSU. AUTHOR INFORMATION AUTHORS AND AFFILIATIONS * College of Nursing / College of Pharmacy, The University of Arizona, Tucson, AZ, USA Elise N. Erickson *
Midwifery Division, School of Nursing, Oregon Health & Science University, Portland, OR, USA Elise N. Erickson * Ouraring Inc, San Francisco, CA, USA Neta Gotlieb * Department of
Obstetrics & Gynecology, School of Medicine, Oregon Health & Science University, Portland, OR, USA Leonardo M. Pereira & Leslie Myatt * Artificial Intelligence for Medical
Systems (AIMS) Lab, Department of Biomedical Engineering, Oregon Health & Science University, Portland, OR, USA Clara Mosquera-Lopez & Peter G. Jacobs Authors * Elise N. Erickson
View author publications You can also search for this author inPubMed Google Scholar * Neta Gotlieb View author publications You can also search for this author inPubMed Google Scholar *
Leonardo M. Pereira View author publications You can also search for this author inPubMed Google Scholar * Leslie Myatt View author publications You can also search for this author inPubMed
Google Scholar * Clara Mosquera-Lopez View author publications You can also search for this author inPubMed Google Scholar * Peter G. Jacobs View author publications You can also search for
this author inPubMed Google Scholar CONTRIBUTIONS Conceptualization: E.N.E., L.M., L.M.P. Methodology: E.N.E., N.G., C.M.L., P.G.J. Investigation: E.N.E., N.G., P.G.J. Visualization: E.N.E.,
P.G.J. Supervision: E.N.E., P.G.J. Writing—original draft: E.N.E., P.G.J. Writing—review & editing: E.N.E., P.G.J., C.M.L., N.G., L.M., L.M.P. CORRESPONDING AUTHOR Correspondence to
Elise N. Erickson. ETHICS DECLARATIONS COMPETING INTERESTS Ouraring Inc. employs one of the authors (N.G.), in both scientific and product development roles. Research support in the form of
data access and devices for the study were provided by Ouraring Inc. The remaining authors declare no competing interests. ADDITIONAL INFORMATION PUBLISHER’S NOTE Springer Nature remains
neutral with regard to jurisdictional claims in published maps and institutional affiliations. SUPPLEMENTARY INFORMATION SUPPLEMENTARY MATERIAL REPORTING SUMMARY RIGHTS AND PERMISSIONS OPEN
ACCESS This article is licensed under a Creative Commons Attribution 4.0 International License, which permits use, sharing, adaptation, distribution and reproduction in any medium or format,
as long as you give appropriate credit to the original author(s) and the source, provide a link to the Creative Commons license, and indicate if changes were made. The images or other third
party material in this article are included in the article’s Creative Commons license, unless indicated otherwise in a credit line to the material. If material is not included in the
article’s Creative Commons license and your intended use is not permitted by statutory regulation or exceeds the permitted use, you will need to obtain permission directly from the copyright
holder. To view a copy of this license, visit http://creativecommons.org/licenses/by/4.0/. Reprints and permissions ABOUT THIS ARTICLE CITE THIS ARTICLE Erickson, E.N., Gotlieb, N.,
Pereira, L.M. _et al._ Predicting labor onset relative to the estimated date of delivery using smart ring physiological data. _npj Digit. Med._ 6, 153 (2023).
https://doi.org/10.1038/s41746-023-00902-y Download citation * Received: 21 February 2023 * Accepted: 10 August 2023 * Published: 19 August 2023 * DOI:
https://doi.org/10.1038/s41746-023-00902-y SHARE THIS ARTICLE Anyone you share the following link with will be able to read this content: Get shareable link Sorry, a shareable link is not
currently available for this article. Copy to clipboard Provided by the Springer Nature SharedIt content-sharing initiative