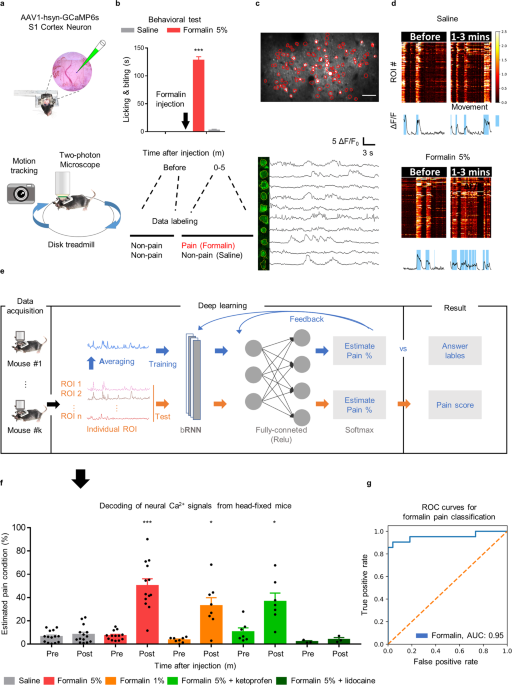
- Select a language for the TTS:
- UK English Female
- UK English Male
- US English Female
- US English Male
- Australian Female
- Australian Male
- Language selected: (auto detect) - EN
Play all audios:
ABSTRACT Chronic pain remains an intractable condition in millions of patients worldwide. Spontaneous ongoing pain is a major clinical problem of chronic pain and is extremely challenging to
diagnose and treat compared to stimulus-evoked pain. Although extensive efforts have been made in preclinical studies, there still exists a mismatch in pain type between the animal model
and humans (i.e., evoked vs. spontaneous), which obstructs the translation of knowledge from preclinical animal models into objective diagnosis and effective new treatments. Here, we
developed a deep learning algorithm, designated AI-bRNN (Average training, Individual test-bidirectional Recurrent Neural Network), to detect spontaneous pain information from brain cellular
Ca2+ activity recorded by two-photon microscopy imaging in awake, head-fixed mice. AI-bRNN robustly determines the intensity and time points of spontaneous pain even in chronic pain models
and evaluates the efficacy of analgesics in real time. Furthermore, AI-bRNN can be applied to various cell types (neurons and glia), brain areas (cerebral cortex and cerebellum) and forms of
somatosensory input (itch and pain), proving its versatile performance. These results suggest that our approach offers a clinically relevant, quantitative, real-time preclinical evaluation
platform for pain medicine, thereby accelerating the development of new methods for diagnosing and treating human patients with chronic pain. SIMILAR CONTENT BEING VIEWED BY OTHERS A
SYSTEMATIC REVIEW OF NEUROPHYSIOLOGICAL SENSING FOR THE ASSESSMENT OF ACUTE PAIN Article Open access 26 April 2023 NEOCORTICAL CIRCUITS IN PAIN AND PAIN RELIEF Article 14 June 2021
FIRST-IN-HUMAN PREDICTION OF CHRONIC PAIN STATE USING INTRACRANIAL NEURAL BIOMARKERS Article 22 May 2023 INTRODUCTION Spontaneous ongoing pain is a primary complaint in patients with chronic
intractable pain1. It leads to a heavy financial burden on society, affects the daily life of patients, and increases their suicide rates2. Conventional analgesics for chronic pain are
frequently associated with severe side effects3. For example, opioids, a widely prescribed class of drugs for chronic pain, are most commonly used in the United States, where they have
caused health crises such as addiction, overdose, and even death4,5. Recently, opioids have been an inevitable component of pain treatment in COVID-19 patients, raising concerns about side
effects6. Numerous non-opioid analgesics and diagnostic methods have been suggested based on a growing understanding of the mechanisms of pain in animal models. However, few have been
successfully translated into clinical interventions7,8,9. A mismatch in pain type between humans and animals (i.e., spontaneous versus stimulus-evoked pain) has been claimed to be a major
reason for these failures10. Many challenges exist in the objective assessment of spontaneous pain in animal models as well as human patients, and researchers have typically measured
stimulus-evoked pain instead of spontaneous pain due to this restriction11. The lack of quantitative measurement techniques for spontaneous pain has impeded the translation of a vast amount
of preclinical knowledge into clinical diagnosis and treatment. Although several methods have been developed in preclinical contexts to overcome this issue, they have inevitable limitations.
The grimace scale (GS) identified spontaneous pain in rodents based on their facial expressions12. However, the GS could not be applied to animal models of subchronic or chronic pain,
including persistent inflammatory or neuropathic pain. Another method, the conditioned place preference (CPP) paradigm, can assess the presence of spontaneous neuropathic pain and analgesic
effects by associating pain relief with a place that has a specific environmental cue13. However, this method is time consuming and requires the pharmacokinetics and administration route of
the drug to be considered. In addition, drugs that have an impact on learning and memory or reward systems are limited with CPP14. Thus, there is an unmet need for a new methodology to
objectively quantify spontaneous pain and the effects of painkillers. The neuronal processing of pain information involves multiple brain areas. Among these, the primary somatosensory cortex
(S1) plays a key role in the perception and discrimination of pain sensation by encoding its intensity, location, and temporal course15,16,17. This led us to hypothesize that neuronal
activity patterns in the mouse S1 differ between spontaneous pain and non-pain conditions and that spontaneous pain can be measured quantitatively based on this discrepancy. Neurons in the
S1 receive and discriminate various forms of somatosensory input, including touch, itch and pain17,18,19,20. This complexity makes it difficult to extract pain-specific information from
neuronal activity patterns. Hence, to detect spontaneous pain information from noise-polluted signals in S1, we employed a bidirectional recurrent neural network (bRNN), a deep learning
method specialized in time-series data analysis21. Furthermore, we optimized the preprocessing steps (Average training and Individual test, AI) for supervised learning from biology-driven
data. The consequent deep learning model, designated AI-bRNN, successfully classified spontaneous pain in various pain models with different prognoses and evaluated the effects of various
analgesics. Additionally, we demonstrated that AI-bRNN is versatile, performing well for other brain areas and cell types as well as other types of somatosensation. MATERIALS AND METHODS
EXPERIMENTAL ANIMALS All mice were C57BL/6 males aged 5–6 weeks old at the start of the experiments. The mice were housed in groups of two to minimize stress. The vivarium was controlled
with a 12/12-h light/dark cycle, and all experiments were performed during daylight hours. All experimental procedures were approved by the Seoul National University Institutional Animal
Care and Use Committee and performed in accordance with the guidelines of the National Institutes of Health. BEHAVIOR TEST The formalin test was performed in mice that had been individually
exposed to the observation chamber. Before the test, the mice were acclimated to the chamber for 1 h on 3 consecutive days. Ten microliters of 5% or 1% formalin solution was injected into
the right hind paw; the mouse was then immediately put back into the chamber. We recorded the time spent in nociceptive behavior (licking and biting of the injected paw) every 5 min. In the
experiments involving analgesic drugs, ketoprofen (100 mg/kg, 50 μl, i.p.) or 2% lidocaine (10 μl, subplantar, s.c.) was administered 20 min before the formalin injection. To quantify the
relieving effect of gabapentin/venlafaxine (GB/VX) on partial sciatic nerve ligation (PSL)-induced neuropathic mechanical allodynia, the mice were placed in a 12 (d) × 8 (w) × 6 (h) cm clear
plastic cage on a metal mesh. Behavioral tests were performed 30, 60, and 120 min after GB (100 mg/kg) and VX (50 mg/kg) administration. Mechanical allodynia was assessed using the von Frey
filament (Linton Instrumentation, Norfolk, UK). Specifically, von Frey filaments delivering different bending forces (2.36, 2.44, 2.83, 3.22, 3.61, 3.84, 4.08, and 4.31 expressed as the log
of the bending force in mg) were applied to the right hind paw using the up-down method, and the threshold force corresponding to 50% withdrawal was determined22. The experimenters were
blinded to the treatments that the animals had received. SURGICAL PREPARATION FOR IMAGING IN AWAKE MICE All surgical procedures were performed under isoflurane anesthesia (1–1.5%). To
minimize edema and related inflammation, dexamethasone (0.2 mg/kg) and meloxicam (20 mg/kg) were administered by subcutaneous injection. A cranial window was made above the area of the left
S1 corresponding to the hind paw (size: 2 × 2 mm; center relative to bregma: lateral, 1.5 mm, posterior, 0.5 mm)23,24. A small craniotomy was carefully performed using a #11 surgical blade.
The exposed cortex was perfused with artificial cerebrospinal fluid, and an adeno-associated virus expressing GCaMP6s (AV-1-PV2824; produced by the University of Pennsylvania Gene Therapy
Program Vector Core) was injected into S1 (30–50 nl per site; 200–300 μm from the surface) using a broken glass electrode (20–40 μm tip diameter). After virus injection, the exposed cortex
was covered with a thin cover glass (Matsunami, Japan), and the margin between the skull and the cover glass was tightly sealed using Vetbond (3 M) and dental cement. For imaging of the
ipsilateral cerebellar Bergmann glia, a small craniotomy was performed over lobule IV/V of the cerebellar vermis. AAV5.GfaABC1d.Lck-GCaMP6f was delivered to the cerebellum (100–300 μm
beneath the surface) as described above. TWO-PHOTON CA2+ IMAGING IN AWAKE MICE For awake imaging, mice were habituated to the treadmill under head-fixed conditions for 40 min per day over 2
weeks. Ca2+ imaging was performed using a two-photon microscope (Zeiss LSM 7 MP, Carl Zeiss, Jena, Germany) equipped with a water immersion objective lens (Apochromat 20, NA = 1.0, Carl
Zeiss). Two-photon excitation at 900 nm for GCaMP6s imaging was carried out using a mode-locked Ti:sapphire laser system (Chameleon, Coherent, USA). Data were acquired using ZEN software
(Zeiss Efficient Navigation, Carl Zeiss) at 4.4 Hz for imaging of S1 and 32 Hz for imaging of the cerebellar Bergmann glia. MOTION TRACKING DURING CA2+ IMAGING Mouse locomotion was recorded
using a video camera. Motion tracking was performed by a custom program written in LabVIEW (National Instruments, USA) and was synchronized with two-photon imaging by a trigger generated in
the program. Mouse locomotion was recorded at a rate of 64 Hz using a high-speed CCD camera (IPX-VGA210, IMPERX, USA) with infrared illumination (DR4-56R-IR85, LVS, S. Korea). The recorded
video had 64 frames per second, with a frame size of 720 × 480 pixels. To assess the level of locomotion, the difference in intensity of each pixel between frames was calculated and
summarized across all the pixels. If the summarized value of a frame exceeded an arbitrary threshold determined by an experimenter blinded to the treatment information, the frame was scored
as a locomotion-positive frame. EXPERIMENTAL MODELS OF SPONTANEOUS PAIN For the formalin-induced pain model, 10 μl of formalin (5% or 1%) or vehicle solution was injected into the right hind
paw. For the capsaicin model, 10 μl of capsaicin (0.01%) was delivered to the right hind paw. For the Complete freund’s adjuvant (CFA) model, 10 μl of CFA was injected subcutaneously into
the plantar surface of the right hind paw. For the chemotherapy-induced peripheral neuropathy model, oxaliplatin (6 mg/kg) or 5% glucose was administered intraperitoneally. For the
neuropathic pain model, PSL surgery was performed on the right hind paw under isoflurane anesthesia. The right sciatic nerve was exposed at the upper thigh of the mouse, and the nerve was
ligated to a diameter of one-third to one-half of the original value with a 9–0 suture. In the assessment of analgesic effects, GB (100 mg/kg) and VX (50 mg/kg) were coadministered to the
PSL animals 3 and 10 d after surgery. Imaging and behavioral tests were performed 30 min after the intraperitoneal injection of the drugs. For the itch model, chloroquine (100 μg/10 μl) was
delivered to the right hind paw. Imaging was performed 1–5 min after chloroquine administration. EXTRACTING CA2+ TRACES AND EVENT DETECTION The imaging data were motion corrected using the
Turboreg algorithm (Biomedical Imaging Group, Swiss Federal Institute of Technology, Lausanne, Switzerland). Regions of interest (ROIs) were detected using the CNMF-E algorithm25 and then
manually reviewed. Spatial information on the ROIs was imported into ImageJ (https://imagej.nih.gov/ij/), and the average fluorescence in each ROI was calculated along with the frame. The
events were detected from the extracted Ca2+ traces using MLSpike26, an open-source algorithm. The hyperparameters for MLSpike were set as follows: _a_ = 0.3, tau = 1, saturation = 0.1,
finetune.sigma = 0.02, and drift.parameter = 0.1. The drift signal from MLSpike was used to calculate the amplitude and frequency of the events. CA2+ ACTIVITY NORMALIZATION A Gaussian
window27 was applied to reduce the noise of the extracted Ca2+ traces. The average value of the activity below the 70th percentile in each ROI was used as the baseline fluorescence activity
(F0). All activity signals were transformed to dF/F0 in each ROI to normalize the scale range. The length of the data sequence was fixed at 497 frames. When the length of the sequence
exceeded 497 frames, the data were divided using the window slicing method (window size of 497 frames, step size of 10 frames). PREPROCESSING FOR DEEP LEARNING AND DEEP LEARNING ARCHITECTURE
The Ca2+ imaging data obtained in one imaging session had a size of n × m, where n was the number of ROIs and m was the number of frames (typically 497 frames for 2 min). The deep learning
model was trained to have an output of [1, 0] for the non-pain condition or [0, 1] for the pain condition. The neural network was implemented using Keras28. The input data had dimensions of
(k, 497, 1), where k is the number of input data sequences in the training set, 497 is the length of each data sequence, and 1 is the number of features. This input was forwarded to
bidirectional long short-term memory recurrent neural networks29 activated by a hyperbolic tangent function. Then, the data were fed to two dense layers that had dropout rates of 0.2 and
0.1. These two dense layers were activated according to rectified linear unit (ReLU)30 and sigmoid activation functions, respectively. Finally, the data were fed to a dense layer that was
activated by the softmax function, which is defined as follows: $$S\left( {y_i} \right) = \frac{{e^{y_i}}}{{\mathop {\sum}\limits_i^n {e^{y_i}} }}$$ (1) where y is the activated value, i is
each node (i.e., class), and n is the total number of nodes or classes. The loss function was defined as follows: $$- \frac{1}{N}\mathop {\sum}\limits_i^N {\left[ {y_i\log \hat y_i + \left(
{1 - y_i} \right)\log \left( {1 - \hat y_i} \right)} \right]}$$ (2) where y-hat is the estimated value that was activated by the softmax function, y is the label [1,0] for non-pain or [0, 1]
for pain, and N is the total number of samples. The Adam optimizer31 was used, and the hyperparameters were set to the default values (lr = 0.01, decay = 1e-8, β1 = 0.9, β2 = 0.999). The L2
regularizer was applied to each dense layer to prevent overfitting. Thus, the loss function was redefined as follows: $$- \frac{1}{N}\mathop {\sum}\limits_i^N {\left[ {y_i\log \hat y_i +
\left( {1 - y_i} \right)\log \left( {1 - \hat y_i} \right)} \right]} + \lambda \mathop {\sum}\limits_i^N {W^{2_i}}$$ (3) where W is the summation of the weight values of dense layers and λ
is the arbitrary weight of the L2 regularizer. The initial weights of all layers were set based on the He uniform variance scaling initializer with a fixed random seed to ensure
reproducibility. PAIN CLASSIFICATION DEEP LEARNING MODEL BASED ON S1 ACTIVITY Ca2+ activity of the S1 in formalin-induced pain states was used as ground-truth data for pain. All baseline
data were used as ground-truth data for non-pain. The quantity of pain data for deep learning training was less than the quantity of non-pain data. To balance the class sizes, the pain data
were duplicated. To validate the deep learning model, we used the leave-one-subject-out (LOSO) cross-validation (CV) method. All data obtained from one mouse (subject) were isolated and
assigned to the test session. Non-formalin data (i.e., capsaicin-, CFA-, PSL-, and oxaliplatin-induced pain data) were used in the test session directly because these data were never used in
the training session. PAIN CLASSIFICATION DEEP LEARNING MODEL BASED ON CEREBELLAR GLIAL CELL ACTIVITY The Ca2+ activity of the cerebellar Bergmann glia in the capsaicin-induced pain state
was used as ground truth data for pain. All baseline data were used as ground-truth data for non-pain. The Ca2+ imaging data of the cerebellum Bergmann glia (19200 frames per subject) were
downsampled to 1/40 (479 frames). The locomotor information was used as a second feature, such that the input to the neural network had the shape of (k, 479, 2). For the test, the LOSO-CV
method was applied. ITCH CLASSIFICATION DEEP LEARNING MODEL BASED ON S1 ACTIVITY The Ca2+ activity of S1 in the chloroquine-induced itch state was used as ground truth data for itch. All
baseline data were used as ground truth data for non-itch. The validation of the itch classification model was performed with the CV method. To compare itch and pain signals in S1, we used
itch data as a test set for the pain classification deep learning model trained with formalin-induced pain signals. STATISTICS Statistical analyses were performed using GraphPad Prism 7
(GraphPad Software, Inc.) or Python (SciPy library32). Two-factor repeated-measures ANOVA and Student–Newman–Keuls post hoc tests were used in Supplementary Fig. 6a, b. Pearson correlation
analysis was used in Supplementary Fig. 3b and Supplementary Fig. 4c. The Wilcoxon test was used in Fig. 1f. The Mann–Whitney _U_ test was used in other analyses. All values are represented
as the means ± SEM. RESULTS AI-BRNN DETECTS INFORMATION ON FORMALIN-INDUCED SPONTANEOUS PAIN AND THE EFFICACY OF PAINKILLERS FROM S1 NEURONAL CA2+ To identify a prototype of neuronal
activity patterns in spontaneous pain, we recorded S1 neuronal Ca2+ activity during formalin-induced spontaneous pain in awake, head-fixed mice (see Methods for details). Using in vivo
two-photon microscopy and the genetically encoded Ca2+ indicator GCaMP6s, we imaged the Ca2+ activity of layer II/III neurons in the left S1 of head-fixed mice while tracking their motion
using an infrared camera (Fig. 1a). Consistent with previous reports33, pain behaviors (i.e., licking and biting) were most prominent at 0–5 min after a formalin injection (5%, 10 μl, s.c.)
into the right hind paw in freely moving mice. Only the Ca2+ activity signals recorded 1–3 min after the formalin injection were used as ‘pain’ condition signals (Fig. 1b). We extracted raw
Ca2+ traces from each region of interest (ROI) (Fig. 1c) and matched the heatmap-visualized Ca2+ traces (Fig. 1d, top) and the averaged trace (Fig. 1d bottom) with the motion tracking data
(blue background, Fig. 1d, bottom). However, using conventional Ca2+ analyses26, we could not collect pain-specific information from S1 neurons. The amplitude and frequency of Ca2+ events
showed no differences between the pain and non-pain conditions (Supplementary Fig. 1a, b), and the receiver operating characteristic (ROC) curve showed poor AUC scores of 0.61 and 0.55,
respectively (Supplementary Fig. 1c). These negative results may not be surprising, considering that S1 neurons process touch and proprioception as well as pain16,19. Hence, we developed a
deep learning algorithm to detect distinct features of Ca2+ activity that represent spontaneous pain. Given the complexity of biology-driven data, adequate preprocessing steps are critical
for the performance of deep learning model algorithms34. Indeed, when data were fed to the deep learning model without configuration of data preparation, the results showed poor performance
(i.e., training and testing with individual ROI activity signals) (Supplementary Fig. 2a–c). Thus, we developed optimized preprocessing steps through trial and error and decided to use
simplified data (sequential averaged activity signals across the ROIs) for the training session and individual data (sequential activity signals from each ROI) for the test session (Fig. 1e
and Supplementary Fig. 2a–c). Since the formalin pain model exhibits clear, strong, and measurable pain behaviors35, we utilized S1 neuronal Ca2+ activity signals recorded at 1-3 min
following formalin injection as supervisory signals for AI-bRNN. After training the AI-bRNN, we tested its classification performance with the LOSO-CV method. AI-bRNN predicted spontaneous
pain conditions depending on the formalin concentration (5%, 1% and saline; Fig. 1f, g). Mild (ketoprofen, 100 mg/kg, i.p.) and strong (2% lidocaine, s.c.) painkillers reduced the estimated
pain values in the 5% formalin group to the levels of the 1% formalin group and a saline-treated non-pain group (Fig. 1f). All of these pain values estimated by AI-bRNN in head-fixed mice
were similar to the pain behaviors measured in freely moving mice (Supplementary Fig. 3a), with a highly positive correlation between the two (_r_ = 0.97; Supplementary Fig. 3b). Along with
the early phase of pain after formalin injection, we examined the late phase of pain. The results showed a late phase of pain that was distinct from the preceding period, and the estimated
pain level was reduced to zero by injection of ketoprofen (Supplementary Fig. 4a, b). To compare the analgesic effects of ketoprofen between the early and late phases, we normalized the pain
level to 1 for each phase. Ketoprofen showed a significantly stronger analgesic effect in the late phase than in the early phase (Supplementary Fig. 4c). The early phase of formalin pain is
the result of a direct effect on nociceptors, and the late phase is an inflammatory response36. Therefore, the observation that ketoprofen, an anti-inflammatory drug, had a stronger
analgesic effect in the late phase than in the early phase is consistent with a previous report. Together, these results indicate that our deep learning model can quantify the intensity of
spontaneous pain and evaluate the efficacy of analgesics. AI-BRNN CAN BE APPLIED TO OTHER CLINICALLY RELEVANT PAIN MODELS, INCLUDING CHRONIC NEUROPATHIC PAIN Next, we tested whether AI-bRNN
trained on S1 neuronal Ca2+ during formalin-induced pain is broadly applicable to various pain models with different chronicities. Our deep learning algorithm discriminated acute spontaneous
pain induced by capsaicin (0.1%, s.c.) from the non-pain condition (Fig. 2a). AI-bRNN also distinguished subchronic spontaneous pain on Days 1 and 3 after injection of complete Freund’s
adjuvant (CFA; 10 μl, s.c.) (Fig. 2b). We note that this could not be accomplished by the measurement of facial expression using GS37 or by analyzing locomotor behavior (Supplementary Fig.
5a, d) or the mean calcium activity of S1 (Supplementary Fig. 5b, e). Additionally, the pain index estimated by AI-bRNN was not correlated with S1 calcium activity or movement (Supplementary
Fig. 5c), indicating that the deep learning model detects a feature distinct from body movement and the simple intensity of S1 calcium activity. In addition to CFA-induced pain, AI-bRNN
detected subchronic pain conditions in a mouse model of chemotherapy-induced peripheral neuropathy induced by oxaliplatin treatment (6 mg/kg, i.p.). The pain value estimated by AI-bRNN was
significant at 3 d but returned to the control level at 10 d after oxaliplatin injection (Fig. 2c), consistent with the previously known symptomatic time course of this model38. These
results indicate that AI-bRNN can discriminate capsaicin-, CFA- or oxaliplatin-induced pain from the non-pain condition, although the model was trained exclusively with data from mice with
formalin-induced pain (Fig. 2d). The diagnosis and treatment of spontaneous pain in neuropathic pain conditions have been a major challenge for pain researchers for decades. The previous
approach using the GS could not detect pain in a chronic neuropathic pain model. Another method, CPP, merely detected the existence of an aversive state suggestive of spontaneous pain in
model animals using pharmacological intervention that involves the reward system. Our approach using AI-bRNN could identify the condition of spontaneous pain in neuropathic pain mice at the
early (3 d) and late (10 d) phases following PSL injury (Fig. 2e–g) without the use of pharmacological intervention. We also applied a sliding window method (segmentation of estimated pain
values by 2-min time windows) and tracked the sequential change in the estimated pain values. Using this technique, we revealed when, how long, and at what degree of severity PSL group mice
felt spontaneous pain (Fig. 2f, Supplementary Fig. 6a–c). We then asked whether our method could be used to measure the efficacy of analgesic drugs against spontaneous neuropathic pain.
Combined treatment using gabapentin (GB; 100 mg/kg, i.p.) and venlafaxine (VX; 50 mg/kg, i.p.)39,40, which are clinically utilized analgesics, attenuated the estimated pain value at 3 d
following PSL (Fig. 2e, f). Interestingly, however, the estimated pain value was not reduced when the same drugs were injected 10 d after PSL injury. These results resemble clinical studies
that showed that the effects of these conventional analgesics diminish with the duration of neuropathic pain symptoms in patients41,42. We confirmed using a conventional von Frey test that
the treatment could relieve stimulus-evoked pain (mechanical allodynia) in both phases (Supplementary Fig. 7a, b). Taken together, our findings indicate that AI-bRNN has broad applicability
to various animal models of spontaneous pain and emphasize that painkillers should be assessed separately for different pain types (i.e., evoked vs. spontaneous). VERSATILITY OF AI-BRNN We
then tested the versatility of AI-bRNN by applying it to a different brain region (cerebellum) and cell type (glia), as well as to a different form of somatosensation (itch).
Capsaicin-induced spontaneous pain information is transmitted to the cerebellum43,44, leading to increased neuronal activity and subsequent Ca2+ elevation in the resident Bergmann glia
(unpublished data). We expressed GCaMP6f in the Bergmann glia of cerebellar cortex lobule IV/V to image Ca2+ activity during capsaicin-induced pain (0–10 min after injection) or non-pain
conditions (Fig. 3a, see Methods for details). Since the boundaries of individual Bergmann glia are difficult to discern, we modified our deep learning model to use average Ca2+ activity
from the whole imaging field, rather than individual Ca2+ traces from ROIs, in the test session as well as in the training session. Nevertheless, the algorithm robustly estimated the
capsaicin pain condition (Fig. 3b, c). We also applied AI-bRNN to classify another somatosensation, itch, which shares common neuroanatomical pathways with pain while being a clearly
distinct sensation18. We acquired S1 neuronal Ca2+ activity signals during chloroquine (100 μl, s.c.)-induced spontaneous itch (1–5 min after injection) or non-itch conditions (Fig. 4a) and
used these signals as labeling data for the itch classification model. The results showed that the AI-bRNN approach could distinguish itch signals from non-itch signals (Fig. 4b, d). We also
developed a deep learning model that differentiates itch from pain (Fig. 4c). Chloroquine-induced itch signals were used as labeled itch data (0 at the _y_-axis), and formalin-induced pain
signals were reused as labeled pain data (100 at the _y_-axis); the established AI-bRNN clearly classified itch and pain (Fig. 4d). These results demonstrate the versatility of AI-bRNN.
DISCUSSION Pain researchers and clinicians have long been eager to develop a real-time, scalable pain indicator. Since spontaneous ongoing pain is a major clinical problem and extremely
difficult to treat, researchers have tried to develop intervention methods using various approaches in animal models. However, the successful translation of these methods into the clinic has
yet to be achieved. In this regard, the lack of objective assessment of spontaneous pain in preclinical models has been regarded as a critical obstacle to filling the gap between animal
experiments and clinical practice8,10. Here, we developed AI-bRNN, a deep learning algorithm for spontaneous pain assessment from brain activity signals obtained by two-photon microscopy in
awake mice. We demonstrated that AI-bRNN robustly detects detailed information about spontaneous pain, such as its intensity, time point and duration, regardless of the phase (from acute to
chronic) or the initiating cause of pain (inflammatory or neuropathic), and evaluates the analgesic efficacy of drugs. Thus, our unprecedented approach provides a powerful evaluation
platform for spontaneous pain that will facilitate the successful translation of pain experiments into clinical practice. The performance of our method is vastly superior to that of previous
pain assessment tools that are based on observation of behaviors such as facial expressions12, place preference13, daily activities, food intake45 and ultrasound vocalizations46. First,
AI-bRNN does not depend on behavioral responses; thus, there is no need to consider the motor function, learning ability, or the reward system of the animal. This would be an incomparable
advantage in the study of pain symptoms in models of neurodegenerative diseases, such as Alzheimer’s disease47 or Parkinson’s disease48. Another advantage of AI-bRNN is that once the model
is trained with pain datasets, testing new data requires minimal computing power and time. Therefore, real-time pain detection is entirely feasible (see Supplementary Movie 1) by applying
the established model to the data acquisition step. This real-time analysis provides an advantage in studying the pharmacokinetics of drugs, enabling a better understanding of the intensity
and duration of action of painkillers. Finally, AI-bRNN can be applied not only to neurons in the S1 but also to glia in other brain regions, such as the cerebellum (Fig. 3b, c). This
multiregional applicability provides an additional benefit: when S1 is unavailable due to damage, signals from alternative brain regions could be used as pain indicators. These benefits that
are obtainable through AI-bRNN cannot be achieved using existing methods, such as GS and CPP, which indicates that spontaneous pain detection based on neural activity has more sensitivity
than behavioral responses. Using AI-bRNN, the analgesic efficacy of drugs could be measured as well. The amelioration of pain by each drug treatment could be assessed in different types of
pain models. Systemic administration of ketoprofen, a nonsteroidal anti-inflammatory drug, moderately reduced the pain value estimated by AI-bRNN, while subplantar injection of lidocaine
strongly reduced the pain value in formalin-treated mice. All of these pain values determined by AI-bRNN in head-fixed mice were highly correlated with the pain behaviors measured in freely
moving mice (49,50; see also Fig. 1f and Supplementary Fig. 3a, b). These results indicate that the values estimated by AI-bRNN can be used for quantification of pain and analgesic efficacy.
This is also applicable to further experiments conducted in a mouse model of neuropathic pain. When GB/VX was administered 3 d after nerve injury, AI-bRNN detected clear analgesic effects
of the treatment. On the other hand, AI-bRNN assessed that GB/VX exerted no significant analgesic effects when administered 10 d after nerve injury (Fig. 2e). This result contrasts with
previous reports39,40 and our behavior experiments, which measured the analgesic efficacy of the drugs using a stimulus-evoked response test and observed a reduction in responsive behavior
in animals (Supplementary Fig. 7a, b). Rather, the results from AI-bRNN resemble clinical studies reporting that the analgesic effects of the drugs on neuropathic pain are not
reliable51,52,53, presumably because the drugs could not exert sufficient analgesic effects when administered to patients with chronic pain41,42. Based on these findings, we interpreted our
method with AI-bRNN to represent the clinical situation more relevantly than the conventional stimulus-evoked method, and we propose that painkillers should be assessed separately for
different pain types (i.e., evoked vs. spontaneous). In the optimization process to establish pain detection with deep learning techniques, we considered various approaches in the
configuration of data preparation. Data labeling is a critical part of the supervised learning process34, and inaccurately labeled data cause the deep learning model to perform poorly, as
shown in Supplementary Fig. 2a–c. In this study, we injected formalin into the hind paw of mice to generate severe ongoing pain with a clear time window. The deep learning model showed high
classification performance on various pain states when average ROI activity signals were used to train the model. In contrast, the performance was poor when the Ca2+ activity signals from
individual ROIs were used as the pain label. These results might stem from the complexity in the S1 neuronal processing of sensory signals54,55. Considering that S1 neurons process multiple
sensory signals, non-pain signals would be mixed into all the datasets we obtained. The individual non-pain signals included in the pain-label datasets would act as false pain data and
interfere with the supervised learning. Averaging the Ca2+ activity of the ROIs would increase the signal-to-noise ratio, as information representing pain would be reflected by averaged
activity in proportion to the pain signals included56. Using the averaged Ca2+ activity for training of the deep learning model would reduce the chance of training the model with irrelevant
signals (false pain data). This explains the superior classification performance of the model trained with averaged Ca2+ activity compared to the model trained with individual Ca2+ activity.
In contrast to the training session, we found that the data configuration in the testing session was not crucial for the classification performance of the established model. Although the
use of the AI setting (using the average signal for training and individual signals for testing) showed the highest performance among those that we tested (Supplementary Fig. 2a–c),
sufficient classification performance was also obtained when the averaged signal from ROIs was used in the testing session (AA setting) (Supplementary Fig. 2a, b). Even using the average of
the signals from the entire imaging field without ROI detection could achieve satisfactory classification performance (Fig. 3c). These results of testing with various settings provide
additional flexibility to our deep learning approach. We believe that our approach provides a good example of a suitable optimization process for biology-driven data in supervised learning.
We adapted the bidirectional long short-term memory (LSTM) RNN, which is specialized for time-series data analysis, to the classification model29. bRNN has been reported to outperform other
deep learning models that analyze time-series data57,58. The Ca2+ imaging data are also time series, and we expect that Ca2+ imaging data contain both forward and backward information. This
deep learning architecture requires minimal field-specific knowledge because the feature extraction step is automated. For example, we adopted only the dF/F0 transformation, which is the
procedure commonly applied to Ca2+ imaging. This minimized manual processing step promotes the versatility of AI-bRNN because it should be easily applicable to any type of Ca2+ data
regardless of properties (e.g., event intensity, frequency and decay). We validated this versatility by pain estimation using glial Ca2+ activity recorded from the cerebellum (Fig. 3b, c).
We also showed that AI-bRNN could be successfully applied to a distinct type of somatosensation, itch, which shares a neuroanatomical pathway with pain (Fig. 4b)18, and the model further
distinguished between spontaneous pain and itch (Fig. 4c). Based on the flexibility, broad applicability and versatility of AI-bRNN, we strongly expect that our approach will be further
extended to the detection of specific patterns not only from brain cellular Ca2+ activity but also from other forms of sequential data in the entire field of biology. Recent technical
advances have allowed us to record the activity of multiple neurons in the brain using electrocorticography59,60 or microelectrodes61 in humans. AI-bRNN can analyze the time-series data
obtained with these techniques in real time. Such clinical applications of AI-bRNN, if combined with brain stimulation62, may enable simultaneous diagnosis and treatment of chronic pain in
human patients, which is expected to reduce drug abuse, suicide rate and socioeconomic burden. In summary, AI-bRNN provides a new paradigm for the measurement of spontaneous ongoing pain and
analgesic efficacy. This clinically relevant, scalable, real-time pain indicator paves the way for the successful translation of numerous mechanisms and analgesics discovered in preclinical
animal models into pain relief in human patients. DATA AVAILABILITY Python with the TensorFlow library was used to perform the analysis. All data and code associated with this study are
presented in the paper, supplementary materials, or GitHub repository (https://github.com/KHUSKlab/paindecoding). REFERENCES * Backonja, M. M. & Stacey, B. Neuropathic pain symptoms
relative to overall pain rating. _J. Pain._ 5, 491–497 (2004). Article PubMed Google Scholar * Enright, A. & Goucke, R. The global burden of pain: The tip of the iceberg? _Anesth.
Analg._ 123, 529–530 (2016). Article PubMed Google Scholar * Jamison, R. N., Dorado, K., Mei, A., Edwards, R. R. & Martel, M. O. Influence of opioid-related side effects on
disability, mood, and opioid misuse risk among patients with chronic pain in primary care. _Pain. Rep._ 2, e589 (2017). Article PubMed PubMed Central Google Scholar * Grosser, T., Woolf,
C. J. & FitzGerald, G. A. Time for nonaddictive relief of pain. _Science_ 355, 1026–1027 (2017). Article CAS PubMed Google Scholar * Skolnick, P. & Volkow, N. D. Re-energizing
the development of pain therapeutics in light of the opioid epidemic. _Neuron_ 92, 294–297 (2016). Article CAS PubMed Google Scholar * Lovell, N. et al. Characteristics, symptom
management, and outcomes of 101 patients with COVID-19 referred for hospital palliative care. _J. Pain. Symptom Manag_ 60, e77–e81 (2020). Article Google Scholar * Borsook, D., Hargreaves,
R., Bountra, C. & Porreca, F. Lost but making progress-Where will new analgesic drugs come from? _Sci. Transl. Med._ 6, 249sr3 (2014). Article PubMed PubMed Central CAS Google
Scholar * Mao, J. Current challenges in translational pain research. _Trends Pharmacol. Sci._ 33, 568–573 (2012). Article CAS PubMed PubMed Central Google Scholar * Mogil, J. S.,
Davis, K. D. & Derbyshire, S. W. The necessity of animal models in pain research. _Pain_ 151, 12–17 (2010). Article PubMed Google Scholar * Tappe-Theodor, A. & Kuner, R. Studying
ongoing and spontaneous pain in rodents-challenges and opportunities. _Eur. J. Neurosci._ 39, 1881–1890 (2014). Article PubMed Google Scholar * Hargreaves, K., Dubner, R., Brown, F.,
Flores, C. & Joris, J. A new and sensitive method for measuring thermal nociception in cutaneous hyperalgesia. _Pain_ 32, 77–88 (1988). Article CAS PubMed Google Scholar * Langford,
D. J. et al. Coding of facial expressions of pain in the laboratory mouse. _Nat. Methods_ 7, 447–449 (2010). Article CAS PubMed Google Scholar * King, T. et al. Unmasking the
tonic-aversive state in neuropathic pain. _Nat. Neurosci._ 12, 1364–1366 (2009). Article CAS PubMed PubMed Central Google Scholar * Tappe-Theodor, A., King, T. & Morgan, M. M. Pros
and cons of clinically relevant methods to assess pain in rodents. _Neurosci. Biobehav. Rev._ 100, 335–343 (2019). Article PubMed PubMed Central Google Scholar * Rocchi, L., Casula, E.,
Tocco, P., Berardelli, A. & Rothwell, J. Somatosensory temporal discrimination threshold involves inhibitory mechanisms in the primary somatosensory area. _J. Neurosci._ 36, 325–335
(2016). Article CAS PubMed PubMed Central Google Scholar * Kim, Y. R., Kim, C. E., Yoon, H., Kim, S. K. & Kim, S. J. S1 employs feature-dependent differential selectivity of single
cells and distributed patterns of populations to encode mechanosensations. _Front. Cell. Neurosci._ 13, 132 (2019). Article CAS PubMed PubMed Central Google Scholar * Bushnell, M. C. et
al. Pain perception: is there a role for primary somatosensory cortex? _Proc. Natl Acad. Sci. USA_ 96, 7705–7709 (1999). Article CAS PubMed PubMed Central Google Scholar * Dhand, A.
& Aminoff, M. J. The neurology of itch. _Brain_ 137, 313–322 (2014). Article PubMed Google Scholar * Kim, S. S., Gomez-Ramirez, M., Thakur, P. H. & Hsiao, S. S. Multimodal
interactions between proprioceptive and cutaneous signals in primary somatosensory cortex. _Neuron_ 86, 555–566 (2015). Article CAS PubMed PubMed Central Google Scholar * Khasabov, S.
G. et al. Responses of neurons in the primary somatosensory cortex to itch- and pain-producing stimuli in rats. _J. Neurophysiol._ 123, 1944–1954 (2020). Article CAS PubMed PubMed Central
Google Scholar * Schuster, M. & Paliwal, K. K. Bidirectional recurrent neural networks. _IEEE Trans. Signal Process_ 45, 2673–2681 (1997). Article Google Scholar * Chaplan, S. R.,
Bach, F. W., Pogrel, J. W., Chung, J. M. & Yaksh, T. L. Quantitative assessment of tactile allodynia in the rat paw. _J. Neurosci. Methods_ 53, 55–63 (1994). Article CAS PubMed Google
Scholar * Eto, K. et al. Inter-regional contribution of enhanced activity of the primary somatosensory cortex to the anterior cingulate cortex accelerates chronic pain behavior. _J.
Neurosci._ 31, 7631–7636 (2011). Article CAS PubMed PubMed Central Google Scholar * Kim, S. K. & Nabekura, J. Rapid synaptic remodeling in the adult somatosensory cortex following
peripheral nerve injury and its association with neuropathic pain. _J. Neurosci._ 31, 5477–5482 (2011). Article CAS PubMed PubMed Central Google Scholar * Zhou, P. et al. Efficient and
accurate extraction of in vivo calcium signals from microendoscopic video data. _eLife_ 7, e28728 (2018). Article PubMed PubMed Central Google Scholar * Deneux, T. et al. Accurate spike
estimation from noisy calcium signals for ultrafast three-dimensional imaging of large neuronal populations in vivo. _Nat. Commun._ 7, 12190 (2016). Article CAS PubMed PubMed Central
Google Scholar * Oppenheim, S. Schafer, Ronald W. _Discrete-Time Signal Processing_ 2nd edn, Vol. 1 (Prentice-Hall, Inc., USA, 1999). * Chollet, F. Keras. https://github.com/fchollet/keras
(2015). * Hochreiter, S. & Schmidhuber, J. Long short-term memory. _Neural Comput._ 9, 1735–1780 (1997). Article CAS PubMed Google Scholar * Glorot, X., Bordes, A. & Bengio, Y.
_In_ _Proc. Volume 15: Proceedings of the Fourteenth International Conference on Artificial Intelligence and Statistics_ (eds Gordon Geoffrey, Dunson David, & Dudík Miroslav) 315–323
(Fort Lauderdale, Florida, 2011). * Kingma, D. P., & Ba, J. Adam: A method for stochastic optimization. preprint at https://arxiv.org/abs/1412.6980 (2014). * Jones, E., Oliphant, T.
& Peterson, P. SciPy: Open source scientific tools for Python. https://github.com/scipy/scipy (2001). * Dubuisson, D. & Dennis, S. G. The formalin test: a quantitative study of the
analgesic effects of morphine, meperidine, and brain stem stimulation in rats and cats. _Pain_ 4, 161–174 (1977). Article CAS PubMed Google Scholar * Xu, C. & Jackson, S. A. Machine
learning and complex biological data. _Genome Biol._ 20, 76 (2019). Article PubMed PubMed Central Google Scholar * Hunskaar, S., Fasmer, O. B. & Hole, K. Formalin test in mice, a
useful technique for evaluating mild analgesics. _J. Neurosci. Methods_ 14, 69–76 (1985). Article CAS PubMed Google Scholar * Hunskaar, S. & Hole, K. The formalin test in mice:
dissociation between inflammatory and non-inflammatory pain. _Pain_ 30, 103–114 (1987). Article CAS PubMed Google Scholar * Sotocinal, S. G. et al. The Rat Grimace Scale: a partially
automated method for quantifying pain in the laboratory rat via facial expressions. _Mol. Pain._ 7, 55 (2011). PubMed PubMed Central Google Scholar * Kim, W. et al. Combined effects of
bee venom acupuncture and morphine on oxaliplatin-induced neuropathic pain in mice. _Toxins (Basel)_ 8, 33 (2016). Article CAS Google Scholar * Rode, F., Brolos, T., Blackburn-Munro, G.
& Bjerrum, O. J. Venlafaxine compromises the antinociceptive actions of gabapentin in rat models of neuropathic and persistent pain. _Psychopharmacol. (Berl.)_ 187, 364–375 (2006).
Article CAS Google Scholar * Folkesson, A., Honore, P. H. & Bjerrum, O. J. Co-administered gabapentin and venlafaxine in nerve injured rats: Effect on mechanical hypersensitivity,
motor function and pharmacokinetics. _Scand. J. Pain._ 1, 91–97 (2010). Article PubMed Google Scholar * Ahn, S. H. et al. Gabapentin effect on neuropathic pain compared among patients
with spinal cord injury and different durations of symptoms. _Spine (Philos. Pa 1976)_ 28, 341–346 (2003). Google Scholar * Amr, Y. M. & Yousef, A. A. Evaluation of efficacy of the
perioperative administration of Venlafaxine or gabapentin on acute and chronic postmastectomy pain. _Clin. J. Pain._ 26, 381–385 (2010). Article PubMed Google Scholar * Iadarola, M. J. et
al. Neural activation during acute capsaicin-evoked pain and allodynia assessed with PET. _Brain_ 121, 931–947 (1998). Article PubMed Google Scholar * Moulton, E. A., Schmahmann, J. D.,
Becerra, L. & Borsook, D. The cerebellum and pain: passive integrator or active participator? _Brain Res. Rev._ 65, 14–27 (2010). Article PubMed PubMed Central Google Scholar *
Urban, R., Scherrer, G., Goulding, E. H., Tecott, L. H. & Basbaum, A. I. Behavioral indices of ongoing pain are largely unchanged in male mice with tissue or nerve injury-induced
mechanical hypersensitivity. _Pain_ 152, 990–1000 (2011). Article PubMed PubMed Central Google Scholar * Kurejova, M. et al. An improved behavioural assay demonstrates that ultrasound
vocalizations constitute a reliable indicator of chronic cancer pain and neuropathic pain. _Mol. Pain._ 6, 18 (2010). Article PubMed PubMed Central CAS Google Scholar * Monroe, T. B.,
Gore, J. C., Chen, L. M., Mion, L. C. & Cowan, R. L. Pain in people with Alzheimer disease: potential applications for psychophysical and neurophysiological research. _J. Geriatr.
Psychiatry Neurol._ 25, 240–255 (2012). Article PubMed PubMed Central Google Scholar * Ha, A. D. & Jankovic, J. Pain in Parkinson’s disease. _Mov. Disord._ 27, 485–491 (2012).
Article PubMed Google Scholar * Miranda, H. F., Puig, M. M., Dursteler, C., Prieto, J. C. & Pinardi, G. Dexketoprofen-induced antinociception in animal models of acute pain: synergy
with morphine and paracetamol. _Neuropharmacology_ 52, 291–296 (2007). Article CAS PubMed Google Scholar * Joshi, S. K. & Gebhart, G. F. Nonopioid actions of U50,488 enantiomers
contribute to their peripheral cutaneous antinociceptive effects. _J. Pharmacol. Exp. Ther._ 305, 919–924 (2003). Article CAS PubMed Google Scholar * Wiffen, P. J. et al. Gabapentin for
chronic neuropathic pain in adults. _Cochrane Database Syst. Rev._ 6, CD007938 (2017). PubMed Google Scholar * Rauck, R. et al. Intrathecal gabapentin to treat chronic intractable
noncancer pain. _Anesthesiology_ 119, 675–686 (2013). Article PubMed Google Scholar * Gallagher, H. C., Gallagher, R. M., Butler, M., Buggy, D. J. & Henman, M. C. Venlafaxine for
neuropathic pain in adults. _Cochrane Database Syst. Rev._ 18, CD011091 (2015). Google Scholar * Kim, W., Kim, S. K. & Nabekura, J. Functional and structural plasticity in the primary
somatosensory cortex associated with chronic pain. _J. Neurochem._ 141, 499–506 (2017). Article CAS PubMed Google Scholar * Prescott, S. A. & Ratté, S. in _Conn’s Translational
Neuroscience_ 1st edn, Vol. 1 (ed P. Michael Conn) Ch. 23 (Elsevier Academic Press, Cambridge, 2017). * Hassan, U. & Anwar, M. S. Reducing noise by repetition: introduction to signal
averaging. _Eur. J. Phys._ 31, 453–465 (2010). Article Google Scholar * Apaydin, H. et al. Comparative analysis of recurrent neural network architectures for reservoir inflow forecasting.
_Water_ 12, 1500 (2020). Article Google Scholar * Arisoy, E., Sethy, A., Ramabhadran, B. & Chen, S. (ed.) In _Proc. 40th IEEE International Conference on Acoustics_, _Speech and Signal
Processing_ (Brisbane, Australia, 2015). * Matsushita, K. et al. A fully implantable wireless ECoG 128-channel recording device for human brain-machine interfaces: W-HERBS. _Front.
Neurosci._ 12, 511 (2018). Article PubMed PubMed Central Google Scholar * Hartley, C. et al. Nociceptive brain activity as a measure of analgesic efficacy in infants. _Sci. Transl. Med._
9, 388 (2017). Article Google Scholar * Musk, E. An integrated brain-machine interface platform with thousands of channels. _J. Med. Internet Res._ 21, e16194 (2019). Article PubMed
PubMed Central Google Scholar * Opri, E. et al. Chronic embedded cortico-thalamic closed-loop deep brain stimulation for the treatment of essential tremor. _Sci. Transl. Med_. 12, eaay7680
(2020). Article PubMed PubMed Central Google Scholar Download references ACKNOWLEDGEMENTS We thank Changmin Oh and Yangseok Kim for their valuable advice on deep learning algorithms;
Yoorim Kim, Yong-Seok Lee, Hyunsu Bae and Sun Wook Hwang for critical discussions on the experiments; and Junichi Nabekura and Schuichi Koizumi for providing cover glasses and encouragement.
FUNDING This work was supported by grants from the National Research Foundation of Korea funded by the Korean government (NRF-2017M3C7A1025604, NRF-2017M3A9E4057926, and
NRF-2019R1A2C2086052 to SKK; NRF-2018R1A5A2025964 and NRF-2017M3C7A1029611 to SJK). AUTHOR INFORMATION Author notes * These authors contributed equally: Heera Yoon, Myeong Seong Bak. AUTHORS
AND AFFILIATIONS * Department of Physiology, College of Korean Medicine, Kyung Hee University, Seoul, 02447, Republic of Korea Heera Yoon, Geehoon Chung & Sun Kwang Kim * Department of
Science in Korean Medicine, Graduate School, Kyung Hee University, Seoul, 02447, Republic of Korea Heera Yoon, Myeong Seong Bak, Ji Hwan Lee & Sun Kwang Kim * Department of Physiology,
Seoul National University College of Medicine, Seoul, 03080, Republic of Korea Seung Ha Kim & Sang Jeong Kim * Department of Biomedical Sciences, Seoul National University College of
Medicine, Seoul, 03080, Republic of Korea Seung Ha Kim & Sang Jeong Kim Authors * Heera Yoon View author publications You can also search for this author inPubMed Google Scholar * Myeong
Seong Bak View author publications You can also search for this author inPubMed Google Scholar * Seung Ha Kim View author publications You can also search for this author inPubMed Google
Scholar * Ji Hwan Lee View author publications You can also search for this author inPubMed Google Scholar * Geehoon Chung View author publications You can also search for this author
inPubMed Google Scholar * Sang Jeong Kim View author publications You can also search for this author inPubMed Google Scholar * Sun Kwang Kim View author publications You can also search for
this author inPubMed Google Scholar CONTRIBUTIONS SJK and SKK conceived and supervised the project. HY, MSB, GC, SJK and SKK designed the study. HY performed all the two-photon imaging
experiments examining S1 in awake head-fixed mice and the behavioral tests in freely moving mice. MSB developed the deep learning algorithm (AI-bRNN) with intellectual input from GC and SKK;
MSB also processed and analyzed all the Ca2+ imaging data. SHK conducted the two-photon imaging experiments examining Bergmann glia in the cerebellum. JHL performed and analyzed all the
behavioral tests in freely moving mice. HY, MSB, GC and SKK wrote the manuscript with comments from SJK. All authors read and approved the manuscript. CORRESPONDING AUTHORS Correspondence to
Sang Jeong Kim or Sun Kwang Kim. ETHICS DECLARATIONS COMPETING INTERESTS Neurogrin Inc., founded by the authors, holds the patent applications related to the contents of this article
(10-2019-0173382 in Korea and PCT/KR2020/006221). ADDITIONAL INFORMATION PUBLISHER’S NOTE Springer Nature remains neutral with regard to jurisdictional claims in published maps and
institutional affiliations. SUPPLEMENTARY INFORMATION SUPPLEMENTARY FIGURES 1–7 SUPPLEMENTARY MOVIE 1 RIGHTS AND PERMISSIONS OPEN ACCESS This article is licensed under a Creative Commons
Attribution 4.0 International License, which permits use, sharing, adaptation, distribution and reproduction in any medium or format, as long as you give appropriate credit to the original
author(s) and the source, provide a link to the Creative Commons license, and indicate if changes were made. The images or other third party material in this article are included in the
article’s Creative Commons license, unless indicated otherwise in a credit line to the material. If material is not included in the article’s Creative Commons license and your intended use
is not permitted by statutory regulation or exceeds the permitted use, you will need to obtain permission directly from the copyright holder. To view a copy of this license, visit
http://creativecommons.org/licenses/by/4.0/. Reprints and permissions ABOUT THIS ARTICLE CITE THIS ARTICLE Yoon, H., Bak, M.S., Kim, S.H. _et al._ Development of a spontaneous pain indicator
based on brain cellular calcium using deep learning. _Exp Mol Med_ 54, 1179–1187 (2022). https://doi.org/10.1038/s12276-022-00828-7 Download citation * Received: 29 October 2021 * Revised:
04 May 2022 * Accepted: 23 May 2022 * Published: 18 August 2022 * Issue Date: August 2022 * DOI: https://doi.org/10.1038/s12276-022-00828-7 SHARE THIS ARTICLE Anyone you share the following
link with will be able to read this content: Get shareable link Sorry, a shareable link is not currently available for this article. Copy to clipboard Provided by the Springer Nature
SharedIt content-sharing initiative