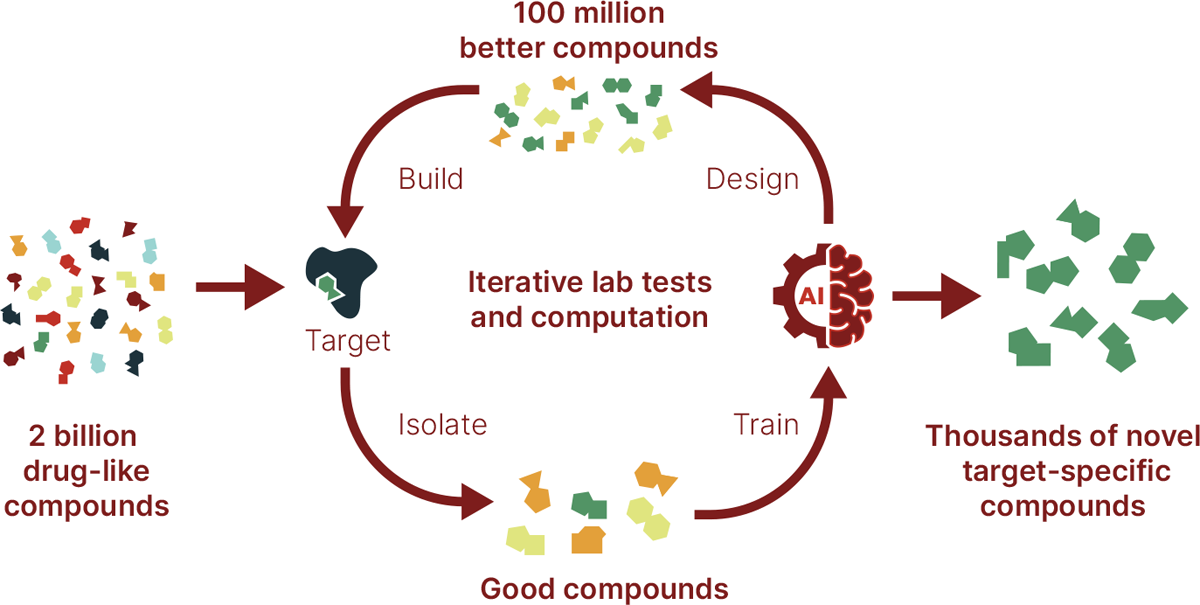
- Select a language for the TTS:
- UK English Female
- UK English Male
- US English Female
- US English Male
- Australian Female
- Australian Male
- Language selected: (auto detect) - EN
Play all audios:
Conventional drug research and development (R&D) is much too slow for most patients, often taking nearly 25 years to translate an idea into a medicine. Hundreds of targets with a strong
connection to human disease still need medicines, mainly because we lack the tools to unlock these novel target classes and challenging mechanisms. Enter Anagenex, a pre-clinical
biotechnology company rethinking small-molecule drug discovery through the lens of directed evolution. The company’s unique combination of ultra-high-throughput biochemistry and artificial
intelligence (AI)-driven experiments enables it to build and test 100 million compounds in a month to discover and optimize highly novel compounds for historically intractable targets (Fig.
1). “Eighty percent of protein targets are currently untouched because they don’t fit conventional ideas about which proteins are amenable to small-molecule medicines. For these proteins,
it’s hard to find a chemical starting point. Unfortunately, AI hasn’t solved this because drug discovery datasets are too small and there’s no way to iteratively refine the AI with large
datasets,” explained Nicolas Tilmans, founder and CEO. “We set out to build massive, high-quality datasets by running large-scale lab experiments creating the first generation of compounds
for a target. Our machine-learning models learn the essence of those datasets and use it to design the next ‘evolved’ generation of 100 million compounds.” COMBINING LAB AND AI APPROACHES
Bringing together an experienced team of experts in chemistry, biology, and machine learning, Anagenex has developed novel chemistries that enable it to rapidly build ultra-high-quality
libraries with increased diversity and test target-focused compounds at unprecedented scale. The company has synthesized dozens of AI-optimized DNA-encoded libraries totaling more than two
billion diverse and drug-like compounds that it screens using proprietary affinity experiments designed to identify the compounds most likely to bind in a biologically relevant way to a
given target. To confirm the results and remove any errors, these ‘hits’ are tested off DNA using a miniaturized affinity-selected mass-spectrometry platform enabling Anagenex to verify
thousands of datapoints in parallel. This results in a unique dataset that measures protein–compound interactions at unprecedented scale and provides its machine-learning models with
extremely clean data. These rich, high-quality data are then used to train proprietary AI models, which generatively design 100 million previously unseen ‘evolved’ target-specific molecules
to experimentally probe structure–activity relationships at extreme scale. Those compounds are then synthesized and tested—iteratively refining the AI’s predictions—in order to identify
highly selective and potent drug candidates. In a recent example, the AI-designed evolved library doubled the number of compounds identified in four initial hit series and found an
additional six completely novel series. In total, Anagenex’s evolved process found 40 times more hits than were identified in the initial library, dramatically expanding options for solving
absorption, distribution, metabolism, and excretion (ADME)/pharmacokinetic (PK) and other medicinal chemistry problems for this target. “Our iterative system creates a virtuous circle
between real lab experiments and computational tools giving us incredible insights into what chemistry works for the target, ultimately saving us from building hundreds of compounds that
will never work while focusing on those that will,” said Tilmans, “and we continue to develop new capabilities.” By controlling its process from start to finish, Anagenex’s expert team can
rapidly develop new lab methodologies and achieve game-changing results with unrivalled speed and accuracy. Anagenex recently developed two new selection approaches enabling it to identify
compounds that bind covalently to targets, and to test compounds for membrane permeability directly within its massive compound libraries. Those experimental results can then be integrated
into tailormade neural networks with a holistic view of the entire experimental process, boosting accuracy. PROGRAMS AND PARTNERING This novel integrated lab and computational platform has
rapidly delivered a robust pipeline of programs addressing unmet medical needs. These include novel compounds for multiple validated but challenging oncology targets whose existing clinical
candidates have toxicity issues. It also has identified allosteric binders to immunology targets. The company expects to nominate its first development candidate within the next 12–18
months, and will continue to advance other oncology and autoimmune programs into the lead-optimization stage. The long-term strategy is to bring its internal programs to the clinic while
engaging in complementary partnerships to expand the impact of the platform. The first of these partnerships was signed in 2023 with Nimbus Therapeutics to discover small-molecule
therapeutics for multiple indications, but Anagenex has the capacity for a second multi-target partnership in any therapeutic area. “Going after targets that have frustrated the industry for
decades is always a risky business. The power and efficiency of Anagenex’s platform make that risk tolerable, especially considering the potential impact on patients,” said Ryan Kruger,
CSO. “The scale and focus of our custom chemistry and selection system can address some of the hardest targets in drug discovery, delivering precision small molecules to patients faster.”