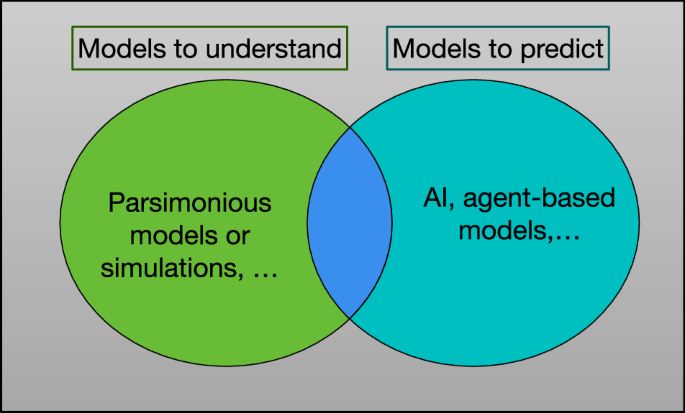
- Select a language for the TTS:
- UK English Female
- UK English Male
- US English Female
- US English Male
- Australian Female
- Australian Male
- Language selected: (auto detect) - EN
Play all audios:
ABSTRACT Mobility is a complex phenomenon encompassing diverse transportation modes, infrastructure elements, and human behaviors. Tackling the persistent challenges of congestion,
pollution, and accessibility requires a range of modeling approaches to optimize these systems. While AI offers transformative potential, it should not be the sole solution. Parsimonious
models remain crucial in generating innovative concepts and tools and fostering collaborative efforts among researchers, policymakers, and industry stakeholders. SIMILAR CONTENT BEING VIEWED
BY OTHERS A GLOBAL EMPIRICAL STUDY ON HOW STREET NETWORKS FACILITATE DRIVING LONGER DISTANCES Article Open access 24 October 2023 HUMAN MOBILITY IS WELL DESCRIBED BY CLOSED-FORM
GRAVITY-LIKE MODELS LEARNED AUTOMATICALLY FROM DATA Article Open access 04 February 2025 EXPLORING THE INTERPLAY BETWEEN POPULATION PROFILE AND OPTIMAL ROUTES IN U.S. CITIES Article Open
access 08 May 2025 CHALLENGES FOR SUSTAINABLE MOBILITY As cities evolve and their populations increase, the question of how people move within (and between) urban environments becomes
increasingly critical. With the growing accessibility of data1 (in particular mobile phone data2), numerous models for human mobility have been developed3, and more recently, machine
learning techniques4. The various modeling approaches are employed to address the challenges of congestion, pollution, and inefficiency in transportation, and play a crucial role in
understanding the dynamics of transportation systems and designing effective strategies to improve their performance. We note that the future of modeling mobility is, however, not just about
predicting traffic patterns or optimizing public transportation; it is about envisioning sustainable and inclusive solutions that cater to the diverse needs of urban dwellers, a much more
difficult goal to achieve. Sustainable mobility faces several challenges ranging from infrastructure and technological innovations, multimodal integration, and environmental planning to more
social sciences-related questions such as affordability, equity and accessibility, and behavioral changes. The list of these challenges makes it clear that the solutions cannot be purely
technological and will need an interdisciplinary effort, ranging from civil engineering to social sciences. Many papers discuss the future of urban mobility and possible scenarios5,6 for
transportation modes likely to be offered in the coming years. In particular, car-dominated urban mobility seems to face an uncertain future, and autonomous and electric vehicles might not
provide a solution that can compete with mass transit6, at least from the point of view of delays due to congestion7. It is, therefore, crucial to explore how urban mobility could evolve,
using simulations that incorporate new modes of transport, mobility options, and multimodality. At a more “physical” level, sustainable mobility relies on urban planning strategies that
prioritize walkability, mixed land use, and transit-oriented development. However, implementing these principles often conflicts with existing zoning regulations, development patterns, and
automobile-centric infrastructure designs. Also, it is essential to develop the infrastructure to support sustainable transportation modes such as public transit, cycling lanes, and charging
stations for electric vehicles. There are, however, some complex challenges: electric vehicles rely on energy grids powered by fossil fuels in some regions, and cycling infrastructure may
have environmental impacts during their construction. Balancing the environmental benefits of sustainable mobility with potential drawbacks is essential and needs to be taken into account in
modeling these problems. Also, modeling social aspects and human responses to changes will be essential to address these questions, which present obvious challenges. The authors in8 explore
various significant factors, including the influence of spatial cognition on human mobility, the formation of new mental maps affecting movement behaviors, the adaptation of humans to
emerging transportation modes, and the impact of algorithms on our mobility patterns. Encouraging individuals to adopt sustainable travel behaviors requires overcoming entrenched habits and
attitudes. Education, incentives, and behavioral nudges can help promote mode shifts towards more sustainable options (and more equity), but changing societal norms and cultural perceptions
of mobility will take time, and modeling these effects is a very difficult task. In this context, it can be noted that AI techniques for opinion mining can be very useful for assessing
citizen’s opinions (see, for example, ref. 9). Enumerating these diverse challenges highlights the intricate nature of mobility, emphasizing that its modeling is far from straightforward.
From a practical standpoint, addressing these challenges requires collaboration among governments, businesses, civil society organizations, and individuals, further complicating the search
for solutions. By tackling infrastructure gaps, promoting behavioral change, fostering innovation, and prioritizing equity, sustainable mobility can become a reality for communities around
the world, but it will be very likely difficult to achieve10,11. It is, therefore, crucial to identify the tools that will help us to achieve these goals. Models, predictions, and
simulations play a pivotal role in this context. Specifically, we will argue here that parsimonious models provide a valuable perspective that should not be overlooked or dismissed.
PREDICTING VS. UNDERSTANDING The scientific study of mobility and transport is at least one hundred years old, and it is impossible to discuss the whole history of this field here.
Historically, earlier discoveries were often driven by the need for simplicity, leading to the development of parsimonious models (also called “descriptive”). Here and throughout the
subsequent discussion, when we mention a parsimonious model, we are referring to a set of assumptions regarding the main mechanisms and ingredients, and calculations conducted within this
framework establish relations between various quantities. What qualifies as parsimonious can be open to subjective judgment, and we can find a large range of models with a number of
parameters going from zero or one to much more. A famous saying attributed to Von Neumann12 says “with four parameters I can fit an elephant, and with five I can make him wiggle his trunk”
which gives a sense of what is meant by parsimonious modeling for a physicist. Ideally, a parsimonious model should incorporate a minimal set of parameters and mechanisms, enabling it to
account for a broad range of empirical observations. This balance between parameter count and data validation is what renders parsimonious models truly valuable. Notably, the gravity model,
introduced in the 1930s and still employed today for predicting the traffic flow between two areas, identified key parameters governing human mobility13,14,15. Additional approaches emerged,
including discrete choice models16, or intervening opportunities17, with subsequent efforts focused on enhancing these approaches. For instance, the radiation model proposed in 2012
exemplifies a parsimonious (yet with predictive capabilities) model18. More generally, over the past century, a wide array of approaches has been suggested, ranging from purely empirical
methods to highly theoretical frameworks, some of which have not been validated with data. Despite the diversity of these studies, two broad categories of models can be identified based on
their primary objectives: understanding or predicting. Predictive models are designed to forecast future trends and outcomes, utilizing numerical techniques such as agent-based models,
microsimulations (see, for example, ref. 19 and references therein), and, more recently, AI techniques. In contrast, models aimed at understanding seek to reveal the underlying mechanisms
and dynamics that drive mobility patterns. These models are usually parsimonious, often relying on analytical approaches but occasionally employing numerical methods, such as simulations on
very simple systems (Fig. 1). In addition, we can, in general, delineate two sub-categories within parsimonious approaches: those primarily aimed at comprehending a phenomenon and
highlighting dominant mechanisms, and those that, while simplifying reality, possess the capability to provide quantitative predictions validated by empirical data. Here, we explore the
interplay between predictive and models to understand (mainly represented by parsimonious models), highlighting their respective strengths and roles in modeling transportation systems.
PREDICTIVE MODELS: ARTIFICIAL INTELLIGENCE While predictive models include all sorts of techniques (such as agent-based and other simulation techniques), we will focus here on the very
promising use of Artificial Intelligence (AI). AI has the potential to address major societal problems, including sustainability20, and also plays a pivotal role in shaping the future of
cities21,22, human mobility, and transportation systems21. This includes predictive maintenance23, autonomous vehicles management24, traffic management25, ride-sharing, and mobility
services, public transportation optimization26, personalized navigation, etc. We cannot here discuss all these applications, but for the example of traffic management, AI algorithms (such as
reinforcement learning, for example, ref. 26) optimize traffic flow by adjusting signal timings, rerouting vehicles to avoid congested areas, reducing delays, and improving travel times.
The impact of this sort of approach will certainly continue to grow in the near future. In the case of public transportation optimization27, AI can enhance public transit systems by
predicting demand (on a short time scale so far), optimizing schedules, and improving route planning, which benefits commuters and reduces environmental impact. Basically, AI algorithms
analyze historical data, real-time traffic conditions, and passenger demand patterns. From this analysis, these algorithms optimize bus and train schedules in order to minimize waiting times
for passengers, and predictive models help anticipate peak hours and allocate resources accordingly, ensuring timely services. AI can also help in network design such as optimizing bus stop
locations, for example, ref. 28. AI also powers self-driving cars29, enabling them to perceive their surroundings, make decisions, and navigate without human intervention. These vehicles
promise safer roads, reduced congestion, and improved efficiency. However, it is important to note that embracing emerging technologies such as autonomous vehicles, shared mobility services,
and mobility-as-a-service platforms presents challenges related to data privacy, cybersecurity, and regulatory frameworks (see, for example, the review about privacy issues in human
mobility studies30. Other applications include road condition monitoring thanks to AI-powered sensors, which can help transportation agencies prioritize maintenance and repairs, ensuring
safer roads for commuters; traffic incident detection thanks to video feeds analysis (from surveillance cameras), that detect accidents, breakdowns, or roadblocks promptly, such that
authorities can respond faster, minimizing disruptions and ensuring smoother traffic flow; and others such as pedestrian detection, driver monitoring, smart parking management, automated
license plate recognition, ride-sharing and mobility as a service, AI-driven navigation apps, etc. Basically, all these predictive models harness historical data and statistical algorithms
to forecast future mobility patterns, offering invaluable insights for planning and decision-making. In particular, machine learning techniques such as regression analysis, time series
forecasting, and neural networks excel at extrapolating trends and identifying patterns within complex datasets. By leveraging predictive models, cities can anticipate traffic congestion,
optimize public transit schedules, and allocate resources more effectively. While AI tools offer numerous benefits in transportation, they also come with several drawbacks and challenges,
and the most important ones are the following. First, AI algorithms rely heavily on data for training and decision-making. However, transportation data can be incomplete, inaccurate, or
outdated, leading to biased or unreliable predictions and decisions31. Second, AI algorithms can perpetuate or amplify biases present in the data used for training32. For example, if the
input transportation data reflects existing inequalities or discriminatory practices, AI tools may inadvertently reinforce these biases in decision-making processes. Third, many AI
algorithms, such as deep learning models, are often regarded as “black boxes” due to their complex structures and internal workings. Although this is not always regarded as a bad thing33,
this lack of interpretability makes it challenging to understand how AI tools arrive at their decisions, hindering transparency and accountability in transportation systems. PARSIMONIOUS
MODELS: CRITICAL PARAMETERS AND DOMINANT MECHANISMS Parsimonious models, on the other hand, focus on understanding the underlying dynamics and causal relationships driving mobility behavior,
in sharp contrast with AI tools. These models often employ mathematical modeling, statistical techniques, network analysis, and simulation methods to dissect complex urban systems. By
uncovering factors such as land use patterns, socioeconomic demographics, and spatial interactions, parsimonious models provide a nuanced understanding of why certain mobility patterns
emerge and evolve over time. These models are often a caricature of reality, sometimes simplifying it to the extreme by retaining only a very small number of parameters and mechanisms. They
usually take into account crucial ingredients from complexity science, such as short-term and long-term dynamics and multiple interactions between agents in the system34. In transportation,
these models aim to describe and analyze the current state or behavior of transportation systems without necessarily making predictions or prescribing actions, but help transportation
planners, engineers, and policymakers understand existing patterns, identify trends, and assess the performance of transportation systems. Some examples of these models include traffic flow,
travel demand, mode choice, or environmental models, illustrating the wide range of their use. There is, therefore, a very large number of such models in the scientific literature, and we
cannot list them all. For the sake of concreteness, we discuss this idea of parsimonious models on two simple examples35,36 that illustrate their capacity to help us understand a phenomenon
and sometimes also to make predictions. In the first example35, we considered a simplified model of mode choice between car and mass rapid transit for the journey-to-work: an individual has
a probability \(p\) to be in the vicinity of a mass rapid transit station and in this case, has to compare the generalized cost of car versus mass transit (and with probability \(1-p\), the
individual takes his car). This model leads to the very simple result that the share of car traffic \(T/P\) (car users over the population) is equal to the proportion \(1-p\) of individuals
who do not have access to mass rapid transit. Such a result can be tested on various cities and, despite its simplicity, proved to be very accurate (see Fig. 2, left). Furthermore, this
model allows us to compute the CO2 emitted per capita (which is roughly proportional to the gasoline consumption per capita) by cars in cities, and the calculation leads to a product of
three factors: $${Q}_{{{CO}}_{2}} \sim (1-p)\sqrt{A}(1+\tau )$$ (1) where \(A\) is the surface area of the city, and \(\tau\) is the additional delay due to congestion (Fig. 2, right). This
model indicates that the renowned finding of Newman and Kenworthy37, which suggests that gasoline consumption per capita is a “simple” decreasing function of urban density, is likely to be
incorrect. The result (1) suggests that if we want to mitigate the CO2 emission (or similarly gasoline consumption), an important ingredient is to increase the public transport density or
the access to it. This simple model thus supports quantitatively the transit-oriented development idea38 and allows us to both predict some quantities and to understand what is the dominant
mechanism (here the accessibility to a mass rapid transit station). However, this generic model should be applied cautiously before drawing broad policy conclusions and must be tailored to
specific contexts. In contrast, there are models that may not offer predictions for individual cases but nonetheless enable the identification of dominant mechanisms and enhance our
understanding of a phenomenon. For instance, investigating empirically the sources of population growth in cities leads to a stochastic equation36 describing the temporal changes of its
population \(P(t)\): $$\frac{{dP}}{{dt}}=\eta P+D{P}^{\beta }\zeta .$$ (2) where \(\eta\) is a Gaussian noise, \(\zeta\) a Levy noise (and \(D\), \(\beta\) are positive constant). An
important point to emphasize is that this equation reveals that urban population growth originates primarily from two separate sources: stochastic growth resulting from the balance between
births and deaths (corresponding to the first term of the r.h.s. with a Gaussian noise), and a second source (term with the Levy noise) arising from inter-urban migrations. Even if this
equation cannot predict what will happen for a given city, an interesting result is that this inter-urban migration term described by a Levy noise has the property to exhibit very large
fluctuations that correspond to migration “shocks”. This shows that the fate of a city is not written in stone but can be influenced by policies and governance. This is here an example of a
parsimonious model that allows us to identify the main mechanism of a phenomenon, even if it cannot predict the future of a specific case. There are obviously many other instances, but the
aim here is to showcase the strengths of a parsimonious model: if corroborated by data, it elucidates the primary mechanisms and identifies the crucial parameters—an endeavor that proves
challenging for AI tools. DISCUSSION While predictive and parsimonious models serve distinct purposes, and even if the distinction between them is sometimes blurred, their integration holds
probably the key to comprehensive urban mobility analysis. Predictive models can inform short-term decision-making and tactical interventions, such as optimizing traffic signal timings or
rerouting public transit routes. Meanwhile, parsimonious models offer a deeper understanding of long-term trends, facilitating strategic planning initiatives like urban redevelopment or
transportation infrastructure investments. Advanced technologies enable predictive models to incorporate real-time data feeds, enhancing their accuracy and responsiveness to dynamic urban
environments. Similarly, parsimonious models benefit from access to large-scale, granular datasets, allowing for more nuanced insights into emerging mobility phenomena. The synergy between
predictive and parsimonious models fosters feedback loops that drive continuous learning and improvement. Predictive models generate hypotheses about future mobility trends, which can be
supported by parsimonious modeling techniques. Conversely, parsimonious models uncover hidden patterns and anomalies that inform the development of more robust predictive algorithms,
creating a virtuous cycle of knowledge generation and refinement. Even if the limits of AI in our understanding of cities and mobility need to be explored39, the integration of predictive
and parsimonious models will hopefully emerge as a fundamental pillar of urban mobility planning and governance. DATA AVAILABILITY No datasets were generated or analysed during the current
study. REFERENCES * Zhao, K., Tarkoma, S., Liu, S. & Vo, H. Urban human mobility data mining: an overview, in _IEEE International Conference on Big Data_, Washington DC (USA) 1911–1920.
(IEEE, 2016). * Blondel, V., Decuyper, A. & Krings, G. A survey of results on mobile phone datasets analysis. _EPJ Data Sci._ 4, 1–55 (2015). * Barbosa, H. et al. Human mobility: models
and applications. _Phys. Rep._ 734, 1–74 (2018). Article ADS MathSciNet Google Scholar * Luca, M., Barlacchi, G., Lepri, B. & Pappalardo, L. A survey on deep learning for human
mobility. _ACM Comput. Surv._ 55, 1–44 (2021). Article Google Scholar * Miskolczi, M., Földes, D., Munkácsy, A. & Jászberényi, M. Urban mobility scenarios until the 2030s. _Sustain.
Cities Soc._ 72, 103029 (2021). Article Google Scholar * Ceder, A. et al. Urban mobility and public transport: future perspectives and review. _Int. J. Urban Sci._ 25, 455–479 (2021).
Article Google Scholar * Louf, R. & Barthelemy, M. How congestion shapes cities: from mobility patterns to scaling. _Sci. Rep._ 4, 5561 (2014). Article CAS PubMed PubMed Central
Google Scholar * Pappalardo, L., Manley, E., Sekara, V. & Alessandretti, L. Future directions in human mobility science. _Nat. Comput. Sci._ 3, 588 (2023). Article PubMed Google
Scholar * Ghahramani, M., Galle, N., Duarte, F., Ratti, C. & Pilla, F. Leveraging artificial intelligence to analyze citizens’ opinions on urban green space. _City Environ. Interact._
10, 100058 (2021). Article Google Scholar * Banister, D. The sustainable mobility paradigm. _Transp. Policy_ 15, 73–80 (2008). * Hickman, R., Hall, P. & Banister, D. Planning more for
sustainable mobility. _J. Transp. Geogr._ 33, 210–219 (2013). * Wikipedia. “Von Neumann’s Elephant,” [Online]. Available: https://en.wikipedia.org/wiki/Von_Neumann%27s_elephant. [Accessed
06, 2024]. * Reilly, W., & Reilly, W. J. _The Law of Retail Gravitation_ (Knickerbocker Press, 1931). * Zipf, G. The P 1 P 2/D hypothesis: on the intercity movement of persons. _Am.
Sociol. Rev._ 11, 677–686 (1946). Article Google Scholar * Erlander, S. & Stewart, N. _The Gravity Model in Transportation Analysis: Theory and Extensions_ (VSP, 1990). * Bierlaire, M
(1998) in _Operations Research and Decision Aid Methodologies in Traffic and Transportation Management._ 203–227 (Springer, 1998). * Stouffer, S. Intervening opportunities: a theory relating
mobility and distance. _Am. Sociol. Rev._ 5, 845–867 (1940). Article Google Scholar * Simini, F., González, M., Maritan, A. & Barabási, A. A universal model for mobility and migration
patterns. _Nature_ 484, 96–100 (2012). Article ADS CAS PubMed Google Scholar * Wise, S., Crooks, A. & Batty M., Transportation in agent-based urban modelling. In _Agent Based
Modelling of Urban Systems: First International Workshop, ABMUS 2016, Held in Conjunction with AAMAS, Singapore, Singapore, May 10, 2016_, _Revised, Selected, and Invite, in First
International Workshop, ABMUS 2016, Held in Conjunction with AAMAS_, Singapore (2016). * Nishant, R., Kennedy, M. & Corbett, J. Artificial intelligence for sustainability: challenges,
opportunities, and a research agenda. _Int. J. Inf. Manag._ 53, 102104 (2020). Article Google Scholar * Bharadiya, J. Artificial intelligence in transportation systems a critical review.
_Am. J. Comput. Eng._ 6, 34–45 (2023). Article Google Scholar * Allam, Z. & Dhunny, Z. On big data, artificial intelligence and smart cities. _Cities_ 89, 80–91 (2019). Article Google
Scholar * Zonta, T. et al. Predictive maintenance in the Industry 4.0: a systematic literature review. _Comput. Ind. Eng._ 150, 106889 (2020). Article Google Scholar * Ma, Y., Wang, Z.,
Yang, H. & Yang, L. Artificial intelligence applications in the development of autonomous vehicles: a survey. _IEEE/CAA J. Autom. Sinica._ 7, 315–329 (2020). Article Google Scholar *
Ouallane, A., Bahnasse, A., Bakali, A. & Talea, M. Overview of road traffic management solutions based on IoT and AI. _Proc. Comput. Sci._ 198, 518–523 (2022). Article Google Scholar *
Walraven, E., Spaan, M. & Bakker, B. Traffic flow optimization: a reinforcement learning approach. _Eng. Appl. Artif. Intell._ 52, 203–212 (2016). * Abduljabbar, R., Dia, H., Liyanage,
S. & Bagloee, S. Applications of artificial intelligence in transport: an overview. _Sustainability_ 11, 189 (2019). Article Google Scholar * Shatnawi, N., Al-Omari, A. & Al-Qudah,
H. Optimization of bus stops locations using GIS techniques and artificial intelligence. _Proc. Manuf._ 44, 52–59 (2020). Google Scholar * Badue, C. et al. Self-driving cars: a survey.
_Expert Syst. Appl._ 165, 113816 (2021). Article Google Scholar * Monreale, A. & Pellungrini, R. A survey on privacy in human mobility. _Trans. Data Priv._ 16, 51–82 (2023). Google
Scholar * Gallotti, R., Maniscalco, D., Barthelemy, M. & De Domenico, M. The distorting lens of human mobility data, https://arxiv.org/abs/2211.10308 (2022) . * Schwartz, R. et al.
_Towards a Standard for Identifying and Managing Bias in Artificial Intelligence_. (US Department of Commerce, National Institute of Standards and Technology, 2022). * Castelvecchi, D. Can
we open the black box of AI? _Nature_ 538, 20 (2016). Article ADS CAS PubMed Google Scholar * Caldarelli, G. et al. The role of complexity for digital twins of cities. _Nat. Comput.
Sci._ 3, 374–381 (2023). Article CAS PubMed Google Scholar * Verbavatz, V. & Barthelemy, M. Critical factors for mitigating car traffic in cities. _PLoS One_ 14, e0219559 (2019).
Article CAS PubMed PubMed Central Google Scholar * Verbavatz, V. & Barthelemy, M. The growth equation of cities. _Nature_ 587, 397–401 (2020). Article ADS CAS PubMed Google
Scholar * Newman, P. & Kenworthy, J. Gasoline consumption and cities: a comparison of US cities with a global survey. _J. Am. Plan. Assoc._ 55, 24–37 (1989). Article Google Scholar *
Ibraeva, A., de Almeida Correia, G., Silva, C. & Antunes, A. Transit-oriented development: a review of research achievements and challenges. _Transp. Res. Part A Policy Pract._ 132,
110–130 (2020). Article Google Scholar * Batty, M. Artificial intelligence and smart cities. _Environ. Plan. B Urban Analyt. City Sci._ 45, 3–6 (2018). Article Google Scholar Download
references AUTHOR INFORMATION AUTHORS AND AFFILIATIONS * Université Paris-Saclay, CNRS, CEA, Institut de Physique Théorique, Gif-sur-Yvette, France Marc Barthelemy * Centre d’Analyse et de
Mathématique Sociales CAMS, UMR 8557 CNRS-EHESS, Ecole des Hautes Etudes en Sciences Sociales, Paris, France Marc Barthelemy Authors * Marc Barthelemy View author publications You can also
search for this author inPubMed Google Scholar CONTRIBUTIONS M.B. designed and wrote the manuscript. All authors reviewed the manuscript. CORRESPONDING AUTHOR Correspondence to Marc
Barthelemy. ETHICS DECLARATIONS COMPETING INTERESTS The author declares no competing interests ADDITIONAL INFORMATION PUBLISHER’S NOTE Springer Nature remains neutral with regard to
jurisdictional claims in published maps and institutional affiliations. RIGHTS AND PERMISSIONS OPEN ACCESS This article is licensed under a Creative Commons
Attribution-NonCommercial-NoDerivatives 4.0 International License, which permits any non-commercial use, sharing, distribution and reproduction in any medium or format, as long as you give
appropriate credit to the original author(s) and the source, provide a link to the Creative Commons licence, and indicate if you modified the licensed material. You do not have permission
under this licence to share adapted material derived from this article or parts of it. The images or other third party material in this article are included in the article’s Creative Commons
licence, unless indicated otherwise in a credit line to the material. If material is not included in the article’s Creative Commons licence and your intended use is not permitted by
statutory regulation or exceeds the permitted use, you will need to obtain permission directly from the copyright holder. To view a copy of this licence, visit
http://creativecommons.org/licenses/by-nc-nd/4.0/. Reprints and permissions ABOUT THIS ARTICLE CITE THIS ARTICLE Barthelemy, M. The role of parsimonious models in addressing mobility
challenges. _npj. Sustain. Mobil. Transp._ 1, 11 (2024). https://doi.org/10.1038/s44333-024-00011-7 Download citation * Received: 22 April 2024 * Accepted: 25 September 2024 * Published: 06
November 2024 * DOI: https://doi.org/10.1038/s44333-024-00011-7 SHARE THIS ARTICLE Anyone you share the following link with will be able to read this content: Get shareable link Sorry, a
shareable link is not currently available for this article. Copy to clipboard Provided by the Springer Nature SharedIt content-sharing initiative