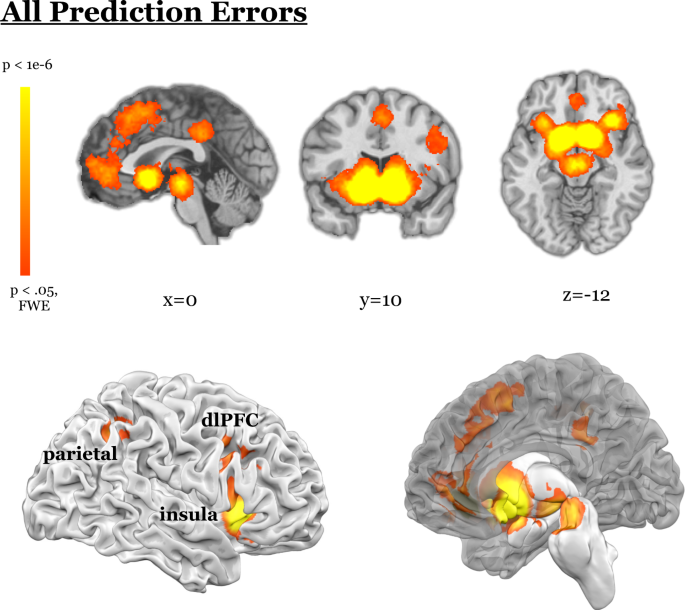
- Select a language for the TTS:
- UK English Female
- UK English Male
- US English Female
- US English Male
- Australian Female
- Australian Male
- Language selected: (auto detect) - EN
Play all audios:
ABSTRACT Prediction errors (PEs) are a keystone for computational neuroscience. Their association with midbrain neural firing has been confirmed across species and has inspired the
construction of artificial intelligence that can outperform humans. However, there is still much to learn. Here, we leverage the wealth of human PE data acquired in the functional
neuroimaging setting in service of a deeper understanding, using an MKDA (multi-level kernel-based density) meta-analysis. Studies were identified with Google Scholar, and we included
studies with healthy adult participants that reported activation coordinates corresponding to PEs published between 1999–2018. Across 264 PE studies that have focused on reward, punishment,
action, cognition, and perception, consistent with domain-general theoretical models of prediction error we found midbrain PE signals during cognitive and reward learning tasks, and an
insula PE signal for perceptual, social, cognitive, and reward prediction errors. There was evidence for domain-specific error signals––in the visual hierarchy during visual perception, and
the dorsomedial prefrontal cortex during social inference. We assessed bias following prior neuroimaging meta-analyses and used family-wise error correction for multiple comparisons. This
organization of computation by region will be invaluable in building and testing mechanistic models of cognitive function and dysfunction in machines, humans, and other animals. Limitations
include small sample sizes and ROI masking in some included studies, which we addressed by weighting each study by sample size, and directly comparing whole brain vs. ROI-based results. You
have full access to this article via your institution. Download PDF SIMILAR CONTENT BEING VIEWED BY OTHERS ASYMMETRIC CODING OF REWARD PREDICTION ERRORS IN HUMAN INSULA AND DORSOMEDIAL
PREFRONTAL CORTEX Article Open access 21 December 2023 EXPLAINING DOPAMINE THROUGH PREDICTION ERRORS AND BEYOND Article 25 July 2024 RETHINKING MODEL-BASED AND MODEL-FREE INFLUENCES ON
MENTAL EFFORT AND STRIATAL PREDICTION ERRORS Article 03 April 2023 INTRODUCTION The reward prediction error (PE) signal in primate midbrain codes a mismatch between expected and experienced
reward. It updates value expectations and drives action selection according to reinforcement learning theory [1]. It has been measured invasively in rodents [2, 3], primates [1], and humans
[4], and studied with functional magnetic resonance imaging (fMRI) in humans [5]. It has been causally implicated in learning with optogenetics [6]. Recently the scope of PE has broadened to
fMRI studies of perceptual [7], social [8], linguistic [9], and causal inferences [10]. We conducted a quantitative meta-analysis of studies of reward PE and PE cast more broadly. We sought
to determine whether PEs invoked as mechanisms across domains of processing share underlying neural substrates, or rather, each domain implements its own specific PE. We integrate findings
from multiple independent studies of PE [11]. Functional imaging studies are expensive so sample sizes are often limited, which limits statistical power to detect true responses, and
undermines confidence in cognitive neuroscience [11]. By summarizing across reported PE signals and weighting the contribution of each study by its quality [11], we learn how varieties of PE
are instantiated in the human brain. METHODS SEARCH STRATEGY AND STUDY SELECTION The Preferred Reporting Items for Systematic Reviews and Meta-Analysis (PRISMA) system guided our search and
selection (see Figure S1). We searched Google Scholar, with the following terms: (1) “predic* AND error* AND (fMRI OR imaging OR neuroimaging) AND (learning OR conditioning)”, and (2)
“reinforcement AND (fMRI OR imaging OR neuroimaging) AND (learning OR conditioning).” Separate searches were conducted for each year between 1999–2018, with publication date restricted to
December 2018 at the latest. We first reviewed abstracts, and excluded those that were irrelevant (e.g., animal data only, reviews, etc). Full text papers were then read by two authors (JAM
& PRC) to determine final inclusion. Next, we selected 13 of the most highly-cited included papers, searched for papers that cited them (i.e., forward search), and examined those papers
for inclusion. We also searched through reference sections of previously published meta-analyses, reviews, and papers identified by searching Neurosynth (Neurosynth.org) for the terms
(“prediction AND error” and “reinforcement AND learning”). 38,831 abstracts were identified: 26,106 from searches and 12,725 from other sources. After removing duplicates, 24,751 abstracts
were reviewed, and 574 full-text papers were evaluated for inclusion. Of those, 263 papers were included in analyses, contributing 464 independent contrasts, and representing 6,454
participants. Only published, peer-reviewed, original research articles were considered. Included studies met the following criteria: (1) Human adult participants 18–65; (2) They employed
fMRI; (3) They provided Talairach or Montreal Neurological Institute (MNI) coordinates; (5) They used image subtraction or parametric modeling (using computational model parameters) to
determine PE activation foci. In the absence of PE, we excluded studies that focused on the anticipation of reward or punishment, extinction, subjective value, or correlations with learning
rate parameters. We excluded papers with clinical groups; unless data from healthy comparison participants were reported separately. We excluded studies of drug administration, including
placebo, since it may be PE mediated [12]. We excluded studies of genetic polymorphisms effects on PE. DATA EXTRACTION AND REDUCTION For each included study we extracted the following
information: N (number of participants contributing to each reported coordinate), xyz coordinate for each significant reported activation, coordinate system (Talairach or MNI), whether
derived from a region of interest (ROI) or whole brain analyses, and whether statistical analyses employed a random/mixed or fixed effects model. Further, we coded several fields of
meta-data, including: (1) whether PE was engendered by a primary or secondary reward; (2) the specific type of outcome (e.g., social, money, points, feedback); (3) the type of computational
model (e.g., Bayesian, temporal difference); (4) whether PE was positive (corresponding to an unexpected occurrence of reward or punishment), or negative (corresponding to an unexpected
omission of reward or punishment); (4) whether PE was signed or unsigned; (5) whether PE was calculated at the time of the cue or during the outcome; (6) whether the task involved
instrumental or Pavlovian conditioning; (7) whether the study involved an appetitive or an aversive outcome; (8a) PE type: typical reward prediction error, or atypical; prediction error
capturing a violation of beliefs; and (8b) atypical PEs were further coded as: cognitive (if expectations based on beliefs were violated, but not beliefs about rewards), effort (if
expectations based on physical or cognitive effort were violated), fictive (PE to an event that could have but did not occur or a choice that was not taken), risk (PE regarding the reward
variance or its square root, standard deviation), and perceptual PEs (generated by unexpected perceptual events without explicit reward or punishment). All information was entered by one
researcher and checked by the second researcher; any disagreements were discussed and resolved. Further details on the coding scheme for PEs are included in Table 1 and in the supplementary
materials (study coding criteria, page 15). Coordinates reported in Talairach space were converted to MNI space using the Tal2MNI algorithm implemented in Matlab
(http://imaging.mrc-cbu.cam.ac.uk/imaging/MniTalairach). STATISTICAL ANALYSIS We used Multilevel Kernel Density Analysis (MKDA) to determine the distribution of peak coordinates [11].
Coordinates from each included contrast were plotted on the standard brain, then convolved with a 10 mm spherical kernel to create a map of voxels within 10 mm of the reported peak. This
resulted in a “Contrast Indicator Map” (CIM), marked with a value of 1 for voxels within the kernel, indicating activation near this voxel, or a value of 0 for voxels outside of the kernel,
indicating no activation near this voxel. Next, a density map was obtained by taking a weighted average of the CIMs, whereby weights are the square root of the sample size for each contrast,
weighing larger studies more heavily. In line with prior meta-analyses, studies that used fixed effects analyses would be down-weighed (0.75); however, none of the included studies employed
fixed effects analyses. This created an interpretable meta-analytic statistic (P) at each voxel, representing the weighed proportion of contrasts that activate within 10 mm of each voxel.
These results were then thresholded using Monte–Carlo (MC) simulation: we compared the meta-analytic statistic (P) with a null-hypothesis density (P0) estimated via simulation. The null
hypothesis was a uniform random distribution of peaks within each contrast in the gray-matter mask of the standard brain. For each CIM, we identified contiguous activation clusters of
suprathreshold voxels. In each of 5000 MC iterations, the spatial location of the activation clusters was selected at random within a gray-matter mask. After each MC iteration, the maximum
cross-density statistic (P) over the whole brain was saved. To threshold the images, we then derived a critical Familywise Error (FWE) rate by determining the weighted cross-density
statistic (P) that exceeds the whole brain maximum in 95% of the MC maps, controlling for false positives at _p_ < 0.05 corrected. After each MC iteration, the largest cluster of
contiguous voxels was saved, and we set a cluster extent threshold at the 95% percentile across iterations, following “cluster extent-based” multiple comparison correction [13]. Our results
represent (1) Meta analysis across all included studies; (2) Meta-analyses across subsets of included contrasts (e.g., those coded as [signed PEs]); (3) Meta-contrasts that compare subsets
of contrasts (e.g., [appetitive > aversive]); and (4) formal conjunctions of separate meta-analytic maps (e.g., [signed and unsigned prediction error]). Identical thresholding procedures
were applied across these analyses: first, images were thresholded at a voxel-wise threshold of _p_ < 0.001, and then we thresholded them with a cluster extent threshold at a level of _p_
< 0.05, FWE, determined with the MC procedure. We created conjunction images using the minimum statistic method [14] with the weighted cross-density statistic (P). Each individual map
contributing to the conjunction was independently thresholded at a level of _p_ < 0.05, FWE using bootstrapping. Then, we calculated a formal conjunction across independently thresholded
images. That is, voxels were included in the conjunction image only if activity exceeded the corrected threshold in all of the contributing maps. The conjunction map was thresholded with the
minimum cluster threshold that exceeded _p_ < 0.05, FWE. Conjunction analyses license conclusions about the voxels shared by particular types of PE. If PEs across domains share voxels we
inferred that they share an underlying circuitry comprising those voxels. If this circuitry was more consistently engaged for one type of PE than the others, we inferred that this type of
PE taxed the circuitry more strongly. We believe this because the magnitude of PE activity typically correlates with the extent of behavioral learning and belief change. Overall, we aimed to
delineate the shared and unique circuitry underlying different domains of PE. RESULTS OMNIBUS CONTRAST Across all 464 contrasts from 263 included papers we found PE signals in the midbrain,
dorsal and ventral striatum, thalamus, amygdala, insula, claustrum, dorsolateral prefrontal cortex (dlPFC), ventrolateral PFC (vlPFC), parietal cortex, precuneus, orbitofrontal and medial
prefrontal cortex, occipital cortex, and posterior and anterior cingulate (Fig. 1, Table S1). PRIMARY VERSUS SECONDARY REWARDS We examined PEs for primary reinforcers (like juice, Fig. S2A,
Table S2A) compared to secondary reinforcers (like money, Fig. S2B, Table S2B). If these are coded relative to some ‘common currency’, they ought to share processing loci [15]. On the other
hand, motivational state and degree of familiarity may generate unique PE loci for primary versus secondary rewards. Conjunction analysis revealed that PE for both primary and secondary
rewards engaged both dorsal and ventral striatum, midbrain, and insula and vlPFC (Fig. S2D, Table S2E). This is consistent with a “common currency” account. However, when we compared them,
primary rewards more consistently induced PEs in the dorsal striatum, amygdala, parahippocampal gyrus, claustrum and insula, anterior cingulate, vlPFC, dmPFC, and supplementary motor area
than secondary (Fig. S2C, Table S2C). Secondary rewards more consistently engaged the ventral striatum and subgenual cingulate (Fig. S2C, Table S2D). Further, PEs for points more commonly
engaged the ventral striatum, thalamus, medial PFC, and subgenual cingulate than those for money (Figure S7, Table S11A), while regions that were more engaged with money than points included
the subgenual cingulate extending into OFC, and distinct regions of dorsal and ventral striatum (Fig. S7, Table S11B). APPETITIVE AND AVERSIVE We examined aversive and reward PEs in human
fMRI studies. Brain regions encoding appetitive PE included vlPFC, both dorsal and ventral striatum, amygdala, thalamus, midbrain, bilateral insula, medial PFC (mPFC), anterior and posterior
cingulate, and vlPFC (Fig. S3A, Table S3A). Aversive PEs were encoded in insula, claustrum, vlPFC, supplementary motor area, dorsomedial PFC (dmPFC), precuneus, both dorsal and ventral
striatum, midbrain, amygdala, parahippocampal gyrus, thalamus, and subgenual cingulate (Fig. S3B, Table S3B). Conjunction analysis revealed both appetitive and aversive PEs engaged the
midbrain and dorsal and ventral striatum, amygdala, parahippocampal gyrus, insula, cingulate, claustrum, and vlPFC (Fig. S3D, Table S3E). However, contrasting appetitive with aversive PE, we
found more consistent appetitive PE responses in dmPFC, mPFC, subgenual cingulate, posterior cingulate, parietal lobe, and both dorsal and ventral striatum (Fig. S3C, Table S3C). There was
more activity for aversive vs. appetitive PEs in the vlPFC, insula, claustrum, dmPFC, anterior cingulate, a more posterior region of the midbrain, as well as distinct regions of dorsal and
ventral striatum, amygdala, hippocampus, and thalamus. (Fig. S3C, Table S3D). PERCEPTUAL AND COGNITIVE PREDICTION ERROR We tested whether regions of the dopamine system also underpin
learning perception and cognition. Computing a conjunction across typical reward, perceptual, and cognitive PEs revealed PE signals in the ventral striatum, dorsal striatum, pallidum,
insula, and vlPFC (Fig. 2D, Table S4D). Predictive processing models of the mind and brain posit a cost function for state transitions (rather than value). Under predictive processing,
sensory hierarchies, like the visual system, ought to compute and exhibit PEs. Indeed, this is what we observe: PEs spanning the visual cortical hierarchy (Fig. 2C, Table S4B). However, it
is unclear whether reward and perceptual prediction errors are computed by the same systems. Some theories suggest they are identical [16]. To test for such overlap, we computed a formal
conjunction of perceptual and typical reward PEs (Fig. S6A, Table S4D). This revealed overlapping activity in the dorsal and ventral striatum, thalamus, and insula. INSTRUMENTAL AND
PAVLOVIAN PREDICTION ERROR Organisms learn passively from the environment (Pavlovian), and actively from the consequences of their actions (Instrumental) [17]. Instrumental PEs engaged the
dorsal and ventral striatum, insula, midbrain, and frontal regions including vlPFC, dmPFC, mPFC, anterior and posterior cingulate, parietal regions, and occipital gyrus (Fig. 3A, Table S5A).
Pavlovian PEs engaged dorsal and ventral striatum, midbrain, anterior cingulate, amygdala, thalamus, insula, and vlPFC extending into OFC (Fig. 3B, Table S5B). The contrast of instrumental
PEs vs. Pavlovian PEs revealed that instrumental PEs were associated with activity in dorsal and ventral striatum, anterior cingulate, posterior cingulate, dorsomedial PFC, frontal eye field
and parietal cortex (Fig. 5C, Table S6C). Pavlovian PEs were more likely to be associated with activity in amygdala, parahippocampal gyrus, putamen, insula, thalamus, dlPFC, dmPFC,
precentral gyrus, a distinct region of cingulate gyrus, and temporal and occipital regions (Fig. 3C, Table S5D). A conjunction revealed dorsal and ventral striatum, pallidum, midbrain and
insula (Fig. 3D, Table S5E). There were more consistent Pavlovian PEs in the OFC. This is congruent with preclinical data, wherein rodent OFC lesions impair updating of stimulus-outcome
associations, but not instrumental learning [18]. However, there may be dissociable learning mechanisms within OFC [19]. It appears medial OFC was more consistently engaged by instrumental
PE, and lateral more consistently engaged by Pavlovian PE. This aligns with rodent work that suggests lateral OFC lesions impair Pavlovian (but not instrumental) learning [20], and medial
lesions impair Instrumental learning [21]. Furthermore, there was also substantial overlap between Instrumental versus Pavlovian learning and appetitive versus aversive PEs (Fig. S8, Table
S12). This may speak to the relative importance of positive versus negative reinforcement in action selection. However, we favor a more mundane explanation: The apparent similarity between
instrumental/appetitive and Pavlovian/aversive PEs may be a function of prevailing experimental trends: human functional neuroimaging studies of instrumental learning rarely employ negative
outcomes. Pavlovian conditioning studies in human participants often employ aversive outcomes. Using a chi-squared test, we found that valence labels (appetitive and aversive) were
differentially related to the instrumental and Pavlovian labels (χ2 = 11.77, _p_ = 0.0006). Specifically, the odds of Instrumental studies being appetitive compared to Pavlovian studies were
3.48–1 [95% CI = 1.72,7.10; relative risk: 1.55, 95% CI = 1.16,2.08]. ACTIVE INFERENCE? Some predictive coding accounts posit a PE minimization mechanism for one’s actions and their impact
upon perception––in this way dopaminergic PEs impact perception [16]. Recent preclinical data support this idea [22]. Active inference accounts suggest that actions that minimize PEs are
selected [23]. The conjunction of Pavlovian, Instrumental, and Perceptual PE thus defines possible circuits for active inference. This analysis revealed claustrum, bilateral insula extending
into OFC, and dorsal and ventral striatum (Fig. S5, Table S9). PRECISION-WEIGHTED AND SOCIAL PREDICTION ERROR Some accounts center on the precision of PE: they contribute to learning to the
extent that they are reliable. We compare and contrast the circuits underlying PEs with and without precision-weighting (Fig. S4). In formal associative learning theory, there are models
that track signed PEs––PEs that have a positive or negative sign – and others that track unsigned PEs, which may increase cue processing, associability, and learning about stimuli with
unpredictable consequences [24] or decrease them, focusing instead on stimuli that serve as reliable predictors [25]. None of the papers in our meta-analysis fell in this latter category.
All the papers that employed unsigned PE modeled errors to increase learning rates; thus, we consider them precision-weighted PE in the predictive processing sense (i.e., that they weight
the impact of PE by its variability). Both signed and unsigned PEs were associated with activity in the midbrain, dorsal and ventral striatum, insula, supplementary motor area, and frontal
eye field (Fig. S3D, Table S8E). However, comparing signed to unsigned PEs revealed more activity in dorsal and ventral striatum, pallidum, medial PFC, and anterior and posterior cingulate
for signed PEs, while unsigned PEs were associated with more consistent activations in cerebellum, dlPFC, dmPFC and a distinct cingulate region, supplementary motor area, supramarginal
gyrus, parietal regions, middle temporal gyrus, claustrum, and disparate regions of insula compared to signed PEs (Fig. S3C, Table S8C). These findings may also inform the mechanisms of
social inference. At issue is whether there are dedicated, informationally encapsulated, social modules in the mind and brain [26]. Alternatively, social inference may, through phylogeny,
have co-opted general precision-weighted inference mechanisms. We computed the intersection between regions evincing PEs during social tasks, and those reflecting non-social PEs, and in
particular precision-weighted PEs. We found extensive overlap (Fig. 4D, Table S6C). Dorsal and ventral striatum, pallidum, vlPFC, orbitofrontal cortex, and insula appeared in the
intersection between social and precision-weighted non-social PEs. We also found relatively more activation for social PEs than other non-social PEs in cingulate, dmPFC, and ventromedial and
orbitofrontal PFC (Fig. 4C). Notably, these regions are not unique to social processes or to social PEs [27, 28], although recent single-unit recordings in human dmPFC did show selectivity
for PE about others’ beliefs, there were neurons selective for sensory PEs, and others sensitive to both [29]. Dorsal and ventral striatum, anterior and posterior cingulate, a distinct
region of mPFC, thalamus, left insula, midbrain, and parahippocampal gyrus were more engaged for non-social PE than social PE. MIDBRAIN PES We employed FWE cluster extent thresholding. It
affords sensitivity to weak and diffuse signals, but has poor spatial specificity, which is problematic for smaller regions like the midbrain. We observed midbrain PE signals in our omnibus
analysis, as well as for reward PE, and cognitive PE, even with this thresholding approach. However, when we focus on the midbrain and relax the cluster extent threshold, we observe midbrain
PE responses for perceptual and social tasks (Fig. S8A, Fig. S9A). REGIONS OF INTEREST VS. WHOLE BRAIN ANALYSES Limiting analyses to a-priori regions of interest (ROIs) is common in
functional neuroimaging. In PE studies, masking often focuses on striatum and midbrain. There is nothing erroneous about this approach, however, it runs the risk of the Drunkards Search
Principle––people search where it is easiest to look (e.g., reasonably, where others have looked). Whilst a-priori preclinical data support searching for reward PEs in the striatum, doing so
may have led our field to ignore other sources of reward PE in the human brain. To explore this possibility, we compared activations from contrasts that employed a-priori ROIs with those
that did not. Results from whole brain contrasts consistently reported PE signals in the dlPFC, mPFC, parietal, and visual cortices more so than studies that employed ROI masks that were
often limited to the basal ganglia and midbrain (Fig. 5A, Fig. 5B, Table S10). A contrast comparing PEs from whole brain compared to ROI analyses showed widespread cortical and subcortical
regions, including medial and lateral PFC, insula, parietal regions, and temporal and occipital cortex (Fig. 5C). We further assessed reporting bias in terms of number of reported foci as a
function of sample size (Fig. S11), but note that our MKDA approach uses contrast as a unit of analysis, weighted by sample size, and is less likely to be distorted by studies with larger
numbers of foci. DISCUSSION We conducted the most comprehensive meta-analysis of fMRI studies of PE to date. Previous meta-analyses examined signed and unsigned PEs [30], Pavlovian and
instrumental learning [5, 31, 32], as well as outcome type and valence [5, 32]. We consider all of these tasks and more, concurrently, and include more studies. Our conjunction analyses
(Figs. 2D, 3D, 4D, purple) suggest a core circuit that processes PE across domains incorporating dorsal and ventral striatum, and insula. We conceptually replicate Sharpe and colleagues
[33], wherein midbrain dopamine neurons respond to violations of causal and perceptual expectation. We observed these signals in the striatum, particularly when we examined the intersection
between Pavlovian, Instrumental and perceptual PE signals. Consistent with predictive processing accounts of vision [34, 35]––we also observed perceptual PE signals spanning the visual
processing hierarchy. There appeared to be regionally compartmentalized PEs for primary and secondary rewards. Primary rewards elicited PEs in the dorsal striatum and amygdala, while
secondary reward PEs were in ventral striatum. This is consistent with the representational transition that occurs with learning [36]. We also found separable PEs for valence domains: caudal
regions of the caudate-putamen are involved in the learning of safety signals and avoidance learning [37,38,39], more anterior striatum is selective for rewards, while more posterior is
selective for losses [40]. We found posterior midbrain aversive PE, consistent with preclinical findings that dopamine neurons––which respond to negative valence––are located more
posteriorly in the midbrain and project to medial prefrontal regions [41]. Additionally, we found both appetitive and aversive PEs in the amygdala, consistent with animal studies
[42,43,44,45,46,47]. The presence of both appetitive and aversive PE signals in the amygdala is consistent with its expanding role regulating learning based on surprise and uncertainty [48]
rather than fear per se. Perhaps conspicuous in its absence, given preclinical work, is the hippocampus, which is often held to be a nexus for reward PE, memory PE, and perceptual PE [49].
This may be because the hippocampus is constantly and commonly engaged throughout task performance. Its PEs may not be resolved by the sluggish BOLD response, which is based on local field
potentials and may represent the projections into a region (and therefore the striatal PE signals we observed may be the culmination of the processing in CA1, CA3, and subiculum).
Furthermore, we have only recently been able to image subfields of the hippocampus (with higher field strengths and more rapid sequences); as higher resolution PE papers accrue we will
revisit the meta-analysis of PEs. Precision weighting of PE has been increasingly emphasized, wherein PEs are accommodated or assimilated depending on their inverse variance. The core PE
circuit seems to deal in precision-weighted as well as signed PE (Fig. 2D, Fig. S3). The human insula has consistently been implicated in precision weighting of reward [50,51,52] and
perceptual PEs [53]. We confirmed these associations. The extensive overlap between precision weighted PEs (across domains) and social PEs (a specific domain, Fig. 4D) is consistent with the
idea that social and non-social inferences share underlying cognitive and neural machinery. A recent study observed overlap between unsigned PE and social conformity in the same
participants––notably in the anterior insular cortex, as we observed [54]. However, multivoxel pattern analyses (MVPA) of the same data suggested independent voxels coded unsigned reward PEs
and social conformity [54]. It is apparent from simulations that univariate and MVPA are sensitive to different data features [55]. MVPA is sensitive to voxel level variability even when
the same linear relationship is present in all voxels [55], and it is insensitive to variability in mean activation across a region [55]. Thus, MVPA is not necessarily the golden-road to
inferences about computation, and, furthermore, the same computational model ought to have been applied to the reinforcement learning and social conformity data, whilst exploring their
shared and unique underlying computational architecture. We take this approach, centering PE across social and non-social studies. Our approach and results are more consistent with the
univariate analysis––suggesting a shared focus on social and precision-weighted PE in the anterior insula and thus, a domain-general account of social inference. However, the social vs.
non-social PE contrast revealed regions that were more consistently engaged by social PEs, but also somewhat engaged by reward PEs more broadly (Fig. 7C). To establish specificity of social
functions, Lockwood et al argue there must be dissociation between social and non-social in algorithm or implementation, and domain-general processes must be ruled out [27]. Here, we found
domain-general precision-weighting had algorithmic and implementational overlap with social inference, consistent with the idea that social inferences tax the general inference machinery,
rather than a separate social processing module. We did, however, find greater responses to social than non-social PE in the dmPFC (Fig. 4C), consistent with the engagement of this region
during theory of mind tasks [56] and single unit recording [29]. It is possible that this region represents PEs specific to the social domain, although this was not clearly the case at the
single neuron level [29]. Some theories of cognitive function and dysfunction posit domain-specific mechanisms [57]. Others hypothesize more domain-general processes [58,59,60]. The
involvement of social versus non-social PE signals in some phenomenon of interest might serve a means of adjudicating. Many of the extra-striatal PE signals we report may have been ignored
because of the practice of a-priori ROI masking. The field had strong expectations of basal ganglia and midbrain PE based on the preclinical electrophysiology. Initially, it was important to
confirm these signals in the human brain by focusing specifically on those regions. However, such an approach has failed to capitalize on one of the strengths of functional neuroimaging;
namely that we can acquire an image of the whole brain every few seconds [61]. Analyses that explored PE signals outside of the narrow striatal field of view tended to report PEs in the
lateral frontal, parietal, and visual cortices. SUMMARY We believe our work has three main mechanistic implications: * 1. There is a core, domain general circuit incorporating the striatum
and insula, and likely midbrain, which signals PEs during perception, cognition, and action, with social agents as well as non-social tasks. This circuitry may serve as a locus of
translation across species. * 2. There are domain-specific and circumscribed PE mechanisms––for example in visual perceptual tasks, and social tasks. Focusing on social PEs may inform the
social deficits observed in people with serious mental illnesses. For the visual, the interplay between perceptual PEs proper, and more general mechanisms may inform the mechanisms of
perception, as well as perceptual aberrations in illness. * 3. The practice of ROI masking, whilst well-grounded in monkey and more recently rodent work, has perhaps limited our inquiries
into PE signaling in humans. The current novel finding that there are numerous cortical targets for general and specific error signals bodes well for mechanistic investigations using
transcranial magnetic stimulation in human health and disease. CONCLUSION These results provide insights into animals, humans, and machines. Preclinical researchers might search for the more
nuanced extra-striatal PEs we found, targeting homologous structures in animal brains. Indeed, this work has already begun [62]. Thus far, careful dissections of circuit mechanisms of PE
have involved several brain regions [63]. Human fMRI of PE, including the present work, may help contextualize and expand this effort by providing whole brain insights. This must of course
be coupled with a deeper inquiry into and appreciation of the mechanisms of the BOLD signal, which likely reflects local field potentials, and thus inputs to a region rather than spiking
within it [64]. Combining incisive and precise recording and manipulation techniques along with BOLD measurements will be particularly revealing, and has been already [65, 66]. Human
researchers might use these maps to choose tasks, analyses, or a priori circuits of interest to study, should they have specific questions or suspect particular PE dysfunctions. Further, by
delineating which algorithms might be implemented in the human brain, and how, our data may be relevant to the development of human-inspired artificial intelligence [67, 68]. More broadly,
we answer critics of fMRI by offering concrete examples of functional imaging data informing models of cognitive function, proffering new testable predictions. DATA AVAILABILITY All maps
featured (included in the figures and described in the text) are available on Neurovault (https://neurovault.org/collections/IMXQLEGV/). These may be used to generate region of interest
masks for each feature, comparison, or conjunction. Tables of coordinates for each contrast and conjunction are available on the Open Science Framework (OSF,
https://osf.io/6nuw7/?view_only=6fa6a080c3434409a07100f7c8088d87), as well as in supplementary information. REFERENCES * Schultz W, Dayan P, Montague PR. A neural substrate of prediction and
reward. Science. 1997;275:1593–9. Article CAS PubMed Google Scholar * Pan WX, Schmidt R, Wickens JR, Hyland BI. Dopamine cells respond to predicted events during classical conditioning:
evidence for eligibility traces in the reward-learning network. J Neurosci. 2005;25:6235–42. Article CAS PubMed PubMed Central Google Scholar * Cohen JY, Haesler S, Vong L, Lowell BB,
Uchida N. Neuron-type-specific signals for reward and punishment in the ventral tegmental area. Nature. 2012;482:85–8. Article CAS PubMed PubMed Central Google Scholar * Zaghloul KA,
Blanco JA, Weidemann CT, McGill K, Jaggi JL, Baltuch GH, et al. Human substantia nigra neurons encode unexpected financial rewards. Science. 2009;323:1496–9. Article CAS PubMed PubMed
Central Google Scholar * Garrison J, Erdeniz B, Done J. Prediction error in reinforcement learning: a meta-analysis of neuroimaging studies. Neurosci Biobehav Rev. 2013;37:1297–310.
Article PubMed Google Scholar * Steinberg EE, Keiflin R, Boivin JR, Witten IB, Deisseroth K, Janak PH. A causal link between prediction errors, dopamine neurons and learning. Nat
Neurosci. 2013;16:966–73. Article CAS PubMed PubMed Central Google Scholar * Iglesias S, Mathys C, Brodersen KH, Kasper L, Piccirelli M, den Ouden HE, et al. Hierarchical prediction
errors in midbrain and basal forebrain during sensory learning. Neuron. 2013;80:519–30. Article CAS PubMed Google Scholar * Behrens TE, Hunt LT, Woolrich MW, Rushworth MF. Associative
learning of social value. Nature. 2008;456:245–9. Article CAS PubMed PubMed Central Google Scholar * Brown M, Kuperberg GR. A hierarchical generative framework of language processing:
linking language perception, interpretation, and production abnormalities in schizophrenia. Front Hum Neurosci. 2015;9:643. PubMed PubMed Central Google Scholar * Corlett PR, Aitken MR,
Dickinson A, Shanks DR, Honey GD, Honey RA, et al. Prediction error during retrospective revaluation of causal associations in humans: fMRI evidence in favor of an associative model of
learning. Neuron. 2004;44:877–88. CAS PubMed Google Scholar * Kober H, Wager TD. Meta‐analysis of neuroimaging data. Wiley Interdiscip Rev: Cogn Sci. 2010;1:293–300. Article Google
Scholar * Schenk LA, Sprenger C, Onat S, Colloca L, Büchel C. Suppression of striatal prediction errors by the prefrontal cortex in placebo hypoalgesia. J Neurosci. 2017;37:9715–23. Article
CAS PubMed PubMed Central Google Scholar * Friston KJ, Worsley KJ, Frackowiak RS, Mazziotta JC, Evans AC. Assessing the significance of focal activations using their spatial extent.
Hum Brain Mapp. 1994;1:210–20. Article CAS PubMed Google Scholar * Friston KJ, Holmes AP, Price CJ, Büchel C, Worsley KJ. Multisubject fMRI studies and conjunction analyses. Neuroimage.
1999;10:385–96. Article CAS PubMed Google Scholar * Levy DJ, Glimcher PW. The root of all value: a neural common currency for choice. Curr Opin Neurobiol. 2012;22:1027–38. Article CAS
PubMed PubMed Central Google Scholar * Friston KJ, Shiner T, FitzGerald T, Galea JM, Adams R, Brown H, et al. Dopamine, affordance and active inference. PLoS Comput Biol. 2012;8:e1002327.
Article CAS PubMed PubMed Central Google Scholar * Dickinson, A, Balliene, BW, The Role of Learning in the Operation of Motivational Systems, in Stevens’ Handbook of Experimental
Psychology. 2002. * Ostlund SB, Balleine BW. Orbitofrontal cortex mediates outcome encoding in Pavlovian but not instrumental conditioning. J Neurosci. 2007;27:4819–25. Article CAS PubMed
PubMed Central Google Scholar * Rich EL, Wallis JD. Medial-lateral organization of the orbitofrontal cortex. J Cogn Neurosci. 2014;26:1347–62. Article PubMed PubMed Central Google
Scholar * Panayi MC, Killcross S. The role of the rodent lateral orbitofrontal cortex in simple Pavlovian cue-outcome learning depends on training experience. Cereb Cortex Commun.
2021;2:tgab010. Article PubMed PubMed Central Google Scholar * Ma C, Jean-Richard-Dit-Bressel P, Roughley S, Vissel B, Balleine BW, Killcross S, et al. Medial orbitofrontal cortex
regulates instrumental conditioned punishment, but not Pavlovian conditioned fear. Cereb Cortex Commun. 2020;1:tgaa039. Article PubMed PubMed Central Google Scholar * Schmack K, Bosc M,
Ott T, Sturgill JF, Kepecs A. Striatal dopamine mediates hallucination-like perception in mice. Science. 2021; 372. https://doi.org/10.1126/science.abf4740. * Friston KJ. The free-energy
principle: a rough guide to the brain? Trends Cogn Sci. 2009;13:293–301. Article PubMed Google Scholar * Pearce JM, Hall G. A model for Pavlovian learning: variations in the effectiveness
of conditioned but not of unconditioned stimuli. Psychol Rev. 1980;87:532–52. Article CAS PubMed Google Scholar * Mackintosh NJ. A theory of attention: variations in the associability
of stimuli with reinforcement. Psychological Rev. 1975;82:pp–298. Article Google Scholar * Adolphs R. Social cognition and the human brain. Trends Cogn Sci. 1999;3:469–79. Article CAS
PubMed Google Scholar * Lockwood PL, Apps MAJ, Chang SWC. Is there a ‘social’ brain? implementations and algorithms. Trends Cogn Sci. 2020;24:802–13. Article PubMed PubMed Central
Google Scholar * Zaki J, Kallman S, Wimmer GE, Ochsner K, Shohamy D. Social cognition as reinforcement learning: feedback modulates emotion inference. J Cogn Neurosci. 2016;28:1270–82.
Article PubMed Google Scholar * Jamali M, Grannan BL, Fedorenko E, Saxe R, Báez-Mendoza R, Williams ZM. Single-neuronal predictions of others’ beliefs in humans. Nature, 2021; 610–4. *
Fouragnan E, Retzler C, Philiastides MG. Separate neural representations of prediction error valence and surprise: evidence from an fMRI meta‐analysis. Hum brain Mapp. 2018;39:2887–906.
Article PubMed PubMed Central Google Scholar * D’Astolfo L, Rief W. Learning about expectation violation from prediction error paradigms–A meta-analysis on brain processes following a
prediction error. Front Psychol. 2017;8:1253. Article PubMed PubMed Central Google Scholar * Chase HW, Kumar P, Eickhoff SB, Dombrovski AY. Reinforcement learning models and their neural
correlates: an activation likelihood estimation meta-analysis. Cogn, Affect, Behav Neurosci. 2015;15:435–59. Article Google Scholar * Sharpe MJ, Chang CY, Liu MA, Batchelor HM, Mueller
LE, Jones JL, et al. Dopamine transients are sufficient and necessary for acquisition of model-based associations. Nat Neurosci. 2017;20:735–42. Article CAS PubMed PubMed Central Google
Scholar * Friston K. A theory of cortical responses. Philos Trans R Soc Lond B Biol Sci. 2005;360:815–36. Article PubMed PubMed Central Google Scholar * Rao RP, Ballard DH. Predictive
coding in the visual cortex: a functional interpretation of some extra-classical receptive-field effects. Nat Neurosci. 1999;2:79–87. Article CAS PubMed Google Scholar * Haber SN, Fudge
JL, McFarland NR. Striatonigrostriatal pathways in primates form an ascending spiral from the shell to the dorsolateral striatum. J Neurosci. 2000;20:2369–82. Article CAS PubMed PubMed
Central Google Scholar * Rogan MT, Leon KS, Perez DL, Kandel ER. Distinct neural signatures for safety and danger in the amygdala and striatum of the mouse. Neuron. 2005;46:309–20. Article
CAS PubMed Google Scholar * Josselyn SA, Falls WA, Gewirtz JC, Pistell P, Davis M. The nucleus accumbens is not critically involved in mediating the effects of a safety signal on
behavior. Neuropsychopharmacology. 2005;30:17–26. Article PubMed Google Scholar * Menegas W, Akiti K, Amo R, Uchida N, Watabe-Uchida M. Dopamine neurons projecting to the posterior
striatum reinforce avoidance of threatening stimuli. Nat Neurosci. 2018;21:1421–30. Article CAS PubMed PubMed Central Google Scholar * Seymour B, Daw N, Dayan P, Singer T, Dolan R.
Differential encoding of losses and gains in the human striatum. J Neurosci. 2007;27:4826–31. Article CAS PubMed PubMed Central Google Scholar * Lammel S, Lim BK, Ran C, Huang KW,
Betley MJ, Tye KM, et al. Input-specific control of reward and aversion in the ventral tegmental area. Nature. 2012;491:212–7. Article CAS PubMed PubMed Central Google Scholar * Belova
MA, Paton JJ, Morrison SE, Salzman CD. Expectation modulates neural responses to pleasant and aversive stimuli in primate amygdala. Neuron. 2007;55:970–84. Article CAS PubMed PubMed
Central Google Scholar * Belova MA, Paton JJ, Salzman CD. Moment-to-moment tracking of state value in the amygdala. J Neurosci. 2008;28:10023–30. Article CAS PubMed PubMed Central
Google Scholar * Morrison SE, Salzman CD. Re-valuing the amygdala. Curr Opin Neurobiol. 2010;20:221–30. Article CAS PubMed PubMed Central Google Scholar * Paton JJ, Belova MA, Morrison
SE, Salzman CD. The primate amygdala represents the positive and negative value of visual stimuli during learning. Nature. 2006;439:865–70. Article CAS PubMed PubMed Central Google
Scholar * Sangha S, Chadick JZ, Janak PH. Safety encoding in the basal amygdala. J Neurosci. 2013;33:3744–51. Article CAS PubMed PubMed Central Google Scholar * Shabel SJ, Janak PH.
Substantial similarity in amygdala neuronal activity during conditioned appetitive and aversive emotional arousal. Proc Natl Acad Sci USA. 2009;106:15031–6. Article CAS PubMed PubMed
Central Google Scholar * Roesch MR, Calu DJ, Esber GR, Schoenbaum G. Neural correlates of variations in event processing during learning in basolateral amygdala. J Neurosci.
2010;30:2464–71. Article CAS PubMed PubMed Central Google Scholar * Lisman JE, Grace AA. The hippocampal-VTA loop: controlling the entry of information into long-term memory. Neuron.
2005;46:703–13. Article CAS PubMed Google Scholar * Preuschoff K, Mohr PN, Hsu M. Decision making under uncertainty. Front Neurosci. 2013;7:218. Article PubMed PubMed Central Google
Scholar * Schultz W, Preuschoff K, Camerer C, Hsu M, Fiorillo CD, Tobler PN, et al. Explicit neural signals reflecting reward uncertainty. Philos Trans R Soc Lond B Biol Sci.
2008;363:3801–11. Article PubMed PubMed Central Google Scholar * Preuschoff K, Bossaerts P. Adding prediction risk to the theory of reward learning. Ann N. Y Acad Sci. 2007;1104:135–46.
Article PubMed Google Scholar * Powers AR, Mathys C, Corlett PR. Pavlovian conditioning-induced hallucinations result from overweighting of perceptual priors. Science. 2017;357:596–600.
Article CAS PubMed PubMed Central Google Scholar * Levorsen M, Ito A, Suzuki S, Izuma K, Testing the reinforcement learning hypothesis of social conformity. Hum Brain Mapp, 2020. *
Davis T, LaRocque KF, Mumford JA, Norman KA, Wagner AD, Poldrack RA. What do differences between multi-voxel and univariate analysis mean? How subject-, voxel-, and trial-level variance
impact fMRI analysis. Neuroimage. 2014;97:271–83. Article PubMed Google Scholar * Denny BT, Kober H, Wager TD, Ochsner KN. A meta-analysis of functional neuroimaging studies of self- and
other judgments reveals a spatial gradient for mentalizing in medial prefrontal cortex. J Cogn Neurosci. 2012;24:1742–52. Article PubMed PubMed Central Google Scholar * Raihani NJ, Bell
V. An evolutionary perspective on paranoia. Nat Hum Behav. 2019;3:114–21. Article PubMed Google Scholar * Reed EJ, Uddenberg S, Suthaharan P, Mathys CD, Taylor JR, Groman SM, et al..
Paranoia as a deficit in non-social belief updating. Elife. 2020; 9:e56345. https://doi.org/10.7554/eLife.56345. * Suthaharan P, Reed EJ, Leptourgos P, Kenney JG, Uddenberg S, Mathys CD, et
al. Paranoia and belief updating during the COVID-19 crisis. Nat Hum Behav. 2021;5:1190–202. * Feeney EJ, Groman SM, Taylor JR, Corlett PR. Explaining delusions: reducing uncertainty through
basic and computational neuroscience. Schizophr Bull. 2017;43:263–72. Article PubMed PubMed Central Google Scholar * Moeller S, Yacoub E, Olman CA, Auerbach E, Strupp J, Harel N, et al.
Multiband multislice GE‐EPI at 7 tesla, with 16‐fold acceleration using partial parallel imaging with application to high spatial and temporal whole‐brain fMRI. Magn Reson Med.
2010;63:1144–53. Article PubMed PubMed Central Google Scholar * Millard SJ, Bearden CE, Karlsgodt KH, Sharpe MJ, The prediction-error hypothesis of schizophrenia: new data point to
circuit-specific changes in dopamine activity. Neuropsychopharmacology, 2021. https://doi.org/10.1038/s41386-021-01188-y. * Watabe-Uchida M, Eshel N, Uchida N. Neural circuitry of reward
prediction error. Annu Rev Neurosci. 2017;40:373–94. Article CAS PubMed PubMed Central Google Scholar * Logothetis NK, Pauls J, Augath M, Trinath T, Oeltermann A. Neurophysiological
investigation of the basis of the fMRI signal. Nature. 2001;412:150–7. Article CAS PubMed Google Scholar * Ferenczi EA, Zalocusky KA, Liston C, Grosenick L, Warden MR, Amatya D, et al.
Prefrontal cortical regulation of brainwide circuit dynamics and reward-related behavior. Science. 2016;351:aac9698. Article PubMed PubMed Central CAS Google Scholar * Lohani S,
Poplawsky AJ, Kim SG, Moghaddam B. Unexpected global impact of VTA dopamine neuron activation as measured by opto-fMRI. Mol Psychiatry. 2017;22:585–94. Article CAS PubMed Google Scholar
* Richards BA, Lillicrap TP, Beaudoin P, Bengio Y, Bogacz R, Christensen A, et al. A deep learning framework for neuroscience. Nat Neurosci. 2019;22:1761–70. Article CAS PubMed PubMed
Central Google Scholar * Marblestone AH, Wayne G, Kording KP. Toward an integration of deep learning and neuroscience. Front Comput Neurosci. 2016;10:94. Article PubMed PubMed Central
Google Scholar Download references FUNDING JAM and HK were supported by R01 DA043690. PRC was supported by R21MH116258 and R01MH12887. This work was supported by the Yale University
Department of Psychiatry, the Connecticut Mental Health Center (CMHC) and Connecticut State Department of Mental Health and Addiction Services (DMHAS). The funders had no role in study
design, data collection and analysis, decision to publish or preparation of the manuscript. AUTHOR INFORMATION Author notes * These authors contributed equally: Philip R. Corlett, Jessica A.
Mollick. AUTHORS AND AFFILIATIONS * Department of Psychiatry, Yale University, New Haven, CT, USA Philip R. Corlett, Jessica A. Mollick & Hedy Kober Authors * Philip R. Corlett View
author publications You can also search for this author inPubMed Google Scholar * Jessica A. Mollick View author publications You can also search for this author inPubMed Google Scholar *
Hedy Kober View author publications You can also search for this author inPubMed Google Scholar CORRESPONDING AUTHORS Correspondence to Philip R. Corlett or Hedy Kober. ETHICS DECLARATIONS
COMPETING INTERESTS The authors declare no competing interests. ADDITIONAL INFORMATION PUBLISHER’S NOTE Springer Nature remains neutral with regard to jurisdictional claims in published maps
and institutional affiliations. SUPPLEMENTARY INFORMATION SUPPLEMENTAL MATERIAL SUPPLEMENTARY TABLES RIGHTS AND PERMISSIONS Reprints and permissions ABOUT THIS ARTICLE CITE THIS ARTICLE
Corlett, P.R., Mollick, J.A. & Kober, H. Meta-analysis of human prediction error for incentives, perception, cognition, and action. _Neuropsychopharmacol._ 47, 1339–1349 (2022).
https://doi.org/10.1038/s41386-021-01264-3 Download citation * Received: 07 September 2021 * Revised: 23 December 2021 * Accepted: 28 December 2021 * Published: 11 January 2022 * Issue Date:
June 2022 * DOI: https://doi.org/10.1038/s41386-021-01264-3 SHARE THIS ARTICLE Anyone you share the following link with will be able to read this content: Get shareable link Sorry, a
shareable link is not currently available for this article. Copy to clipboard Provided by the Springer Nature SharedIt content-sharing initiative