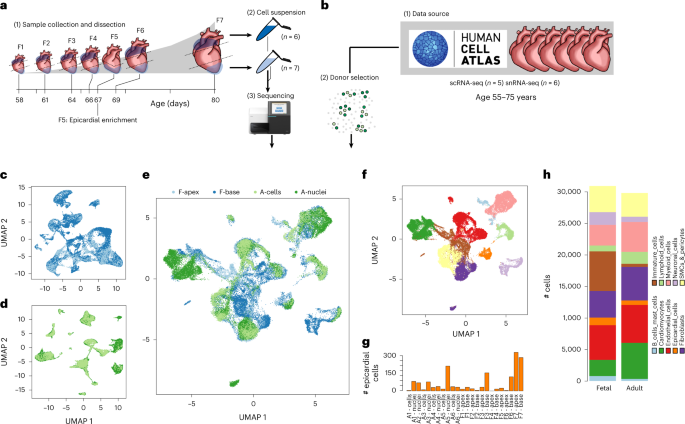
- Select a language for the TTS:
- UK English Female
- UK English Male
- US English Female
- US English Male
- Australian Female
- Australian Male
- Language selected: (auto detect) - EN
Play all audios:
ABSTRACT Re-activating quiescent adult epicardium represents a potential therapeutic approach for human cardiac regeneration. However, the exact molecular differences between inactive adult
and active fetal epicardium are not known. In this study, we combined fetal and adult human hearts using single-cell and single-nuclei RNA sequencing and compared epicardial cells from both
stages. We found that a migratory fibroblast-like epicardial population only in the fetal heart and fetal epicardium expressed angiogenic gene programs, whereas the adult epicardium was
solely mesothelial and immune responsive. Furthermore, we predicted that adult hearts may still receive fetal epicardial paracrine communication, including WNT signaling with endocardium,
reinforcing the validity of regenerative strategies that administer or reactivate epicardial cells in situ. Finally, we explained graft efficacy of our human embryonic stem-cell-derived
epicardium model by noting its similarity to human fetal epicardium. Overall, our study defines epicardial programs of regenerative angiogenesis absent in adult hearts, contextualizes animal
studies and defines epicardial states required for effective human heart regeneration. SIMILAR CONTENT BEING VIEWED BY OTHERS EPICARDIOID SINGLE-CELL GENOMICS UNCOVERS PRINCIPLES OF HUMAN
EPICARDIUM BIOLOGY IN HEART DEVELOPMENT AND DISEASE Article Open access 03 April 2023 CDH18 IS A FETAL EPICARDIAL BIOMARKER REGULATING DIFFERENTIATION TOWARDS VASCULAR SMOOTH MUSCLE CELLS
Article Open access 02 February 2022 ACTIVATION OF A TRANSIENT PROGENITOR STATE IN THE EPICARDIUM IS REQUIRED FOR ZEBRAFISH HEART REGENERATION Article Open access 13 December 2022 MAIN A
major challenge to human health is that the adult human heart does not regenerate. Myocardial infarction (MI) causes a permanent non-contractile and non-conductive scar, which leads to
chronic heart failure and arrhythmia. Much interest has followed the epicardium recently for its key role in heart development and potential to contribute to heart regeneration. The
epicardium emerges from the proepicardium during cardiogenesis as a mesothelial layer of cells surrounding the heart1. During development, epicardial cells may lose mesothelial identity and
undergo epithelial-to-mesenchymal transition (EMT), resulting in a population of epicardial-derived cells (EPDCs) that migrate into the myocardium2. These EPDCs may differentiate into smooth
muscle cells, cardiac fibroblasts and, potentially, endothelial cells3,4. Furthermore, developing epicardial cells and EPDCs secrete potent factors, including WNT, FGFs and PDGFs, which
stimulate vasculogenesis and the proliferation and maturation of cells within the myocardial tissue5. These developmental abilities also translate into a regenerative role. Adult zebrafish
hearts are capable of regeneration, and developmental epicardial genes become highly expressed at the infarcted region, coinciding with the restoration of cardiac muscle6,7. Likewise, when
cardiac regeneration is seen in embryos and neonates of small and large mammals, including humans8,9,10, the active epicardium responds with EMT and the secretion of angiogenic factors11,12.
However, these studies also illustrate that any regenerative window in mammals soon disappears after birth. In contrast, the adult mammalian epicardium is normally quiescent, with reduced
secretory and migratory capacities, and, although it appears to reactivate after injury, the response may not be strong or rapid enough for sufficient regeneration13. However, there is
evidence that a properly active epicardium can still promote regeneration of adult mammalian hearts; studies have established the efficacy and essentiality of epicardial-directed repair
mechanisms, such as thymosin-β-4, FGFs and even exosome-mediated signaling in successful cardiac regeneration14,15,16. Additionally, human embryonic stem cell (hESC)-derived epicardium
(hESC-EPI), when administered alongside hESC-derived cardiomyocytes, increases vascularization, proliferation and survival of myocardial tissue17. Altogether, the evidence suggests that the
epicardium augments heart regeneration and that timely reactivation of epicardial programs offers a promising therapeutic strategy for treating MI in humans. However, without an in-depth
understanding of the epicardium in humans, our ability to translate these models into a therapeutic context is limited. As it stands, it is still not fully known if the adult human
epicardium retains gene expression from development or if its response to injury is similar to that seen in animals or how it relates to hESC-EPI. Additionally, epicardial cells across many
species and humans are identified using _WT1_, _TBX18_ and _TCF21_ (refs. 18, 19) but can be further divided into heterogeneous subpopulations in zebrafish and in hESC-EPI20,21.
Additionally, adult human epicardium may be identified through its co-expression of _BNC1_ and _MSLN_22. However, epicardial heterogeneity may not occur clearly in mammals in vivo23 and has
not been fully explored in humans of any age. Therefore, in light of this missing knowledge, we attempted to define the key factors of epicardial-derived regeneration that are lost in adults
and aimed to capture the different transcriptional states of human epicardium, define age-associated changes in epicardial populations and reveal distinct signaling pathways that are
associated with fetal or adult epicardium. We addressed our aims using single-cell RNA sequencing (scRNA-seq) to isolate epicardial cells in silico and mitigate biases from sorting and
selection. Although scRNA-seq data of both adult and fetal hearts have been generated and analyzed independently, no attempt has been made to combine them22,24. We integrated adult and fetal
human hearts at a single-cell resolution, which allowed us to compare the epicardium in both stages. We approached our dataset from multiple angles and triangulated the epicardium in both
adult and fetal cells using prior knowledge and unbiased clustering of datasets, both mixed and separated. This approach converged on a detailed profile of human epicardial cells, allowing
us to (1) identify fetal epicardial subtypes, (2) create a library of epicardial markers for translating animal studies, (3) reveal an angiogenic program of epicardial communication not
present in adult humans and (4) validate hESC-EPIs as a model of human fetal epicardium. RESULTS TWO STAGES OF THE HUMAN HEART ARE INTEGRATED Seven healthy fetal hearts between the
gestational ages of week 8 and 12 were dissected, taking the base and apex from six donors and the apex attached to peeled epicardium from one (Fig. 1a). These 13 fetal samples were
dissociated, sequenced and aligned using Illumina’s 10x scRNA-seq platform. We removed erythrocytes (Extended Data Fig. 1) and predicted doublets and lower-quality cells to obtain
transcriptomes for 47,473 fetal cells (Supplementary Table 1). In parallel, scRNA-seq and single-nucleotide RNA sequencing (snRNA-seq) data from six healthy adult hearts containing 37,462
cells and 153,053 nuclei were obtained from the Heart Cell Atlas, selecting donors D2–D7 that contained at least three mesothelial annotated cells22 (Fig. 1b). We subsampled the datasets
before downstream integration to equalize group sizes and reduce unwanted variability using multiple stratifications (Methods). This resulted in a more balanced distribution of cell types,
donors and nuclei and amplified rarer cell type populations (Extended Data Fig. 1). After sampling, we were left with 29,779 transcriptomes from adult heart cells or nuclei and 30,889
transcriptomes from fetal heart cells (Supplementary Table 1). We then integrated the samples hierarchically using a reciprocal principal component analysis (RPCA) integration pipeline from
R package Seurat, combining sources within each donor first (Extended Data Fig. 1). We noted a reasonable degree of overlap between sources at this step (Fig. 1c,d). We then integrated the
adult and fetal datasets, resulting in a correspondence between adult and fetal cells within clusters (Fig. 1e). However, fetal cells were more loosely distributed between the well-defined
adult clusters, suggesting quantities of unspecified and immature states of cell types still progressing toward their mature adult equivalent (Fig. 1e). We began with low-resolution
clustering using the Louvain method of community detection to label basic cell type annotations, arriving at ten low-resolution clusters (Fig. 1f), and used differential expression analysis
and previous adult annotations to assign cell type labels and define the epicardial cell cluster (Fig. 1f). We noted that the number of epicardial cells was highly varied among donors, with
most being found in fetal sample F7. However, there was no significant difference between the number of epicardial cells in fetal or adult donors, suggesting that the proportion of
epicardial cells is not markedly different between stages (unpaired Student’s _t_-test; _P_ > 0.05) (Fig. 1g). Overall, most clusters were distinct; however, one cluster appeared to
bridge between multiple other cell types and expressed an ambiguous range of developmental markers. This cluster was composed mostly of fetal cells, which suggests that these were largely
unspecified immature cells (Fig. 1h). EPICARDIAL CELLS EXPRESSING EMT GENES WERE ABSENT IN ADULT HEARTS We performed subclustering and iteratively aggregated these subclusters together
across several resolutions (Extended Data Fig. 2). We selected an intermediate resolution of 19 clusters for downstream analysis where the epicardium was divided into three subpopulations:
8, 9 and 10 (Fig. 2ai). Interestingly, we measured the fraction of fetal cells and found that epicardial clusters 8 and 10 comprised 0.6% and 0% adult cells, respectively, suggesting fetal
specificity. However, cluster 9 was equally split between stages with 47.2% adult cells, suggesting an age-persistent epicardial cell type (Fig. 2aii,b). We carried out a differential
expression analysis among all clusters and combined the upregulated markers in each cluster with previous annotation of adult cells to determine the cell types present across both ages (Fig.
2c, Extended Data Fig. 2 and Supplementary Table 2). In non-epicardial cells, we found that adipocyte cluster 1 and stromal pericyte cluster 18 were almost entirely adult cells (Fig.
2aii,b). The three epicardial subclusters expressed well-established epicardial signature genes _KRT19_, _RARRES2_, _UPK3B_, _WT1_ and _BNC1_ (Supplementary Tables 3 and 4). However, we
labeled cluster 8 as Epicardium_FB-like in light of its expression of fibroblast genes, including _DCN_, _COL1A1_ and _POSTN_ (Fig. 2c,d); cluster 9 as Epicardium_Mesothelial after previous
annotation and broad epicardial gene expression; and cluster 10 as Epicardium_Proliferating with its expression of cell cycle and mitotic genes _CENPF_ and _HMGB2_ (Fig. 2c). Interestingly,
the Epicardium_FB-like cluster appeared to have low _TBX18_ expression, unlike the other epicardial clusters, but expressed genes _TWIST1_ and _SPARC_, which are strongly associated with EMT
(Fig. 2d). Based on this evidence, we describe the fibroblast-like epicardial cells in cluster 8 as a transient population of mesenchymal EPDCs not yet differentiated into
epicardium-derived lineages, which may not be present in the quiescent adult heart. AGING EPICARDIUM LOSES MANY FETAL EPICARDIAL GENE PROGRAMS We isolated epicardial clusters 8, 9 and 10 and
identified six distinct transcriptional modules of co-expressed genes by analyzing dropout patterns only in the cells (Fig. 3a). Cellular commitment toward each module was calculated as a
new feature for principal component analysis (PCA) where age and cell type were orthogonally represented by components 2 and 4 (Fig. 3b); component 1 appeared to be unwanted technical
variation (Methods, Extended Data Fig. 3). Finally, we re-clustered all epicardial cells into 12 states by their commitment to each gene module using the Louvain method of community
detection (Fig. 3biii,c and Supplementary Table 5) and ordered them by mean cellular ranked-age to reveal changes in module commitment and genes caused by aging (Fig. 3d,e and Extended Data
Fig. 3). We found only one aging-associated module (A) including the genes _HP_ (haptoglobin), _SLPI_ (secretory leukocyte peptidase inhibitor) and _PLA2G2A_ (phospholipase A2 group IIA)
(Fig. 3d,e). Commitment to module A was initially low in early fetal states at 13% and increased throughout development, peaking at 44% in adult cells (Fig. 3e). However, fetal epicardium
was committed to many distinct modules (B to E). Module B was seen exclusively in the epicardial state overlapping with Epicardium_Proliferating cells (Fig. 3d,e), whereas all fetal
epicardial cells appeared to be highly committed to module C, containing genes such as _TNNT1_ (troponin T1, slow skeletal type), _SPARC_ (secreted protein acidic and cysteine rich) and
_MGP_ (matrix gla protein). Adult cells expressed between only 4% and 25% of module C genes. Our most interesting finding was in module D, with high commitment in Epicardium_FB-like cells
belonging to epicardial states 6 and 3, with 42% and 33% of its genes expressed, respectively (Fig. 3b,c,e). In contrast, adult mesothelial cells expressed between only 4% and 12% of the
genes in this module. Module D contained fibroblast genes _POSTN_ and _DCN_ (Fig. 3d) and established markers of EMT, such as _TWIST1_, suggesting a signature of EPDCs. Commitment toward
module E was seen mostly in fetal Epicardium_Mesothelial cells, including the genes _SBSPON_ (somatomedin B and thrombospondin type 1 domain containing), _CXCL14_ (C-X-C motif chemokine
ligand 14) and _SFRP5_ (secreted frizzled related protein 5). Lastly, module F may have been associated with technical variables, with more commitment seen in cells when compared with nuclei
(Extended Data Fig. 3). AGING SHIFTS EPICARDIAL FOCUS FROM ANGIOGENESIS TO IMMUNE RESPONSE We then examined the function of each gene module using GprofileR in an over-representation
analysis across Gene Ontology biological processes. First, gene module D was enriched for pro-regenerative processes related to angiogenesis, EMT and wound repair, including blood vessel
development, circulatory system development, angiogenesis, cell migration and extracellular matrix organization (Fig. 3f and Supplementary Table 6). Commitment of epicardium to module D
suggests that the fetal epicardium is poised for angiogenic response, whereas the adult epicardium is not. Second, we noticed that module A was broadly enriched for processes involved in
response to external stimuli, including response to stress, defense and immune response (Fig. 3f and Supplementary Table 6), suggesting that the human epicardium transitions toward an
immune-responsive state with age. This unexpected result reveals an unexplored characteristic of epicardial aging, which may be important for cardiovascular regeneration. Lastly, we found
broad developmental terms, including animal organ morphogenesis and cell differentiation, enriched in the early mesothelial module E and less-specific protein processing terms in fetal
module C (Fig. 3f). Processes found in module B also validated our labeling of proliferative epicardium with the terms ‘cell division’ and ‘nuclear division’. In summary, these results
suggest that aging reduces epicardial commitment toward regenerative angiogenic programs. In particular, it is important to note that the epicardial population most committed to the
regenerative module D was absent in adults. EPICARDIAL MARKERS REVEAL WNT SIGNALING IN FETAL EPICARDIUM We then evaluated the age selectivity of epicardial-specific genes by carrying out
differential expression analyses within each stage. After removing genes describing non-epicardial differences between adult and fetal stages, we found 633 genes upregulated in the
epicardial cluster when compared with other heart cell clusters (Wilcoxon rank-sum; _P_ < 1 × 10−10, log2 fold change > 0.5), constituting 147 fetal markers, 374 adult markers and 112
markers of both stages (Fig. 4a,b and Supplementary Table 7). We also ranked the epicardial genes by their ability to predict epicardial cells using precision, recall and _F-_score (Fig. 4c,
Extended Data Fig. 4 and Supplementary Table 8). First, we found several genes markedly absent in adult epicardium: _SFRP5_ (secreted frizzled related protein 5), _SFRP2_, _CXCL14_ and
_COL9A3_ (collagen type IX alpha 3 chain) (Fig. 4b). Second, the fetal epicardium was best predicted by the shared marker _CA9_ (carbonic anhydrase 9) (_F_-score = 0.51, precision = 0.3,
recall = 0.81), followed by _SFRP5_, _CFI_ (complement factor I), _TNNT1_, _LY6H_ (lymphocyte antigen 6 family member H) and _LGALS2_ (galectin 2). Most interestingly, we found that _SFRP5_
was one of 11 other fetal genes within the Gene Ontology process canonical Wnt signaling pathway, including _SFRP2_, _WNT2B_ (Wnt family member 2B), _RSPO1_ (respondin-1) and _FGF9_
(fibroblast growth factor 9). _SFRP2_ and _SFRP5_ are soluble pleiotropic modulators of WNT signaling and may be essential in myocardial repair25,26, whereas _WNT2B_ is a canonical WNT
ligand found to increase zebrafish cardiomyocyte proliferation after injury27. Additionally, _RSPO1_ was recently implicated in cardiomyocyte compaction during development and identified in
the epicardium of regenerating P1 neonatal mice but not in non-regenerating P7 mice12,28. Lastly, _FGF9_ is implicated in epicardial-mediated regenerative signaling, including
vasculogenesis29. Functionally, this WNT component is of utmost relevance to epicardial-mediated regeneration. Other notable fetal markers may promote regeneration, such as _CA9_ stimulating
cell migration under hypoxia in mice30 as well as _BMP3_ (bone morphogenetic protein 3) and _TGFB3_ (transforming growth factor beta 3). Irrespective of age, our library identified _UPK3B_
(uroplakin 3B) as the most selective epicardial marker validated by reports of its robust expression23,31,32 (Fig. 4c). This was followed by two established markers of epicardium: _ITLN1_
(intelectin-1) and _MSLN_ (mesothelin). Interestingly, _ITLN1_ was in adult module A, linking our results with a clinically observed correlation of serum omentin-1 with age33. We also
identified markers of the epicardium, such as _KLK11_ (kallikrein related peptidase 11), _CALB2_ (calbindin 2) and _SMPD3_ (sphingomyelin phosphodiesterase 3) (Supplementary Table 8). In the
adult epicardium, we found that _HP_ was the best predictive coding gene (_F_-score = 0.44, precision = 0.3, recall = 0.81) (Fig. 4c), followed by _HAS1_ (hyaluronan synthase 1), _SLPI_
(secretory leukocyte peptidase inhibitor), _FAM153B_ (family with sequence similarity 153 member B), _ALOX15_ (arachidonate 15-lipoxygenase) and _RBP4_ (retinol-binding protein 4). _HP_ was
seen in older human fetal epicardium34, suggesting a marker of maturing mesothelial cells that persists into adulthood (Fig. 4c). Lastly, we found no clear marker of the fibroblast-like
epicardial population. However, we used _UPK3B_ as a pan-epicardial marker and repeated scoring between fibroblast-like cluster 8 and mesothelial cluster 9 using only _UPK3B__+_ cells
(Extended Data Fig. 4 and Supplementary Table 8). We found that mesothelial-specific _PRG4_ (proteoglycan 4) and _ITLN1_ suggest spatial separation of epicardial cell types as _PRG4_ encodes
lubricin secreted into pericardial fluid35, and _ITLN1_ encodes omentin-1 associated with epicardial adipose tissue (Fig. 4c). Without these proteins, EPDCs may be deeper within the
myocardium than their _PRG4_-producing counterparts. Other mesothelial genes were _AQP1_, _PLA2G2A_, _SBSPON_ and _TM4SF1_ (Fig. 4c). In the EPDCs, we found _SEMA3D_, involved in concerted
endothelial cell migration36; _CCBE1_, important in mouse cardiac development37; _EGFL6_, associated with angiogenesis38, and _GPC3_, reported to modulate WNT signaling39. Overall, these
results suggested further that these epicardial cells are EPDCs no longer on the surface of the heart. Additionally, we showed that, although aged epicardium maintains a recognizable
identity, it departs from our previous understanding and that aging establishes a novel epicardial state. Lastly, these results continued to show that key regenerative signaling is absent in
adult epicardium, including angiogenic WNT signaling. ADULT HEARTS STILL RESPOND TO FETAL EPICARDIAL SIGNALING To examine the effects of restoring fetal states to adult epicardium, we
predicted paracrine interactions from the epicardium using CellPhoneDB. First, we found more communication from fetal epicardium in comparison with adult epicardium when interacting with
other cells of the adult heart. By restoring fetal states, the largest predicted increase in epicardial communication was seen in endocardial, venous endothelial and neuronal cell
populations given by 88, 66 and 62 interactions, respectively, from fetal Epicardium_FB-like compared with 30, 27 and 16 interactions from adult Epicardium_Mesothelial (Fig. 4d). We then
filtered these interactions for secreted epicardial-specific proteins from the differential expression analysis (Fig. 4a,b) and found that the volume of predicted communication between fetal
epicardial and adult endocardial cells could be pro-angiogenic (Fig. 4e). Our results show that this was an age-associated loss of epicardial secretions as opposed to a loss of receptivity
by adult hearts, as low communication from adult epicardium persisted even when interacting with the fetal heart, whereas both adult and fetal hearts received similar signals from fetal
epicardium (Fig. 4e). Specifically, signaling from fetal epicardium consisted of _NRP2_-mediated signaling with endothelial _VEGF_s. However, it is unknown whether epicardial _NRP2_ is
soluble or membrane-anchored as part of this established pathway of angiogenesis40,41. We also found further evidence of WNT signaling from the epicardium, with _WNT2B_ communication between
fetal epicardium and _FZD4_ found on adult endocardial, endothelial and stromal pericyte clusters. Reduced _FZD4_ activity has been seen to markedly decrease vascular density in kidneys42.
These interactions corroborate fetal angiogenic potential while highlighting target cells for epicardial WNT signaling. To our surprise, only a small volume of communication was seen with
cardiomyocytes from both adult and fetal epicardium (Fig. 4d). One of these was another WNT signaling protein, _RSPO1_, predicted to interact with _LRG4_ in cardiomyocytes as well as smooth
muscle cells, adipocytes, fibroblasts and neuronal cells (Fig. 4e). Lastly, we found _TGFB3_ signaling in fetal but not adult epicardium, agreeing with previous observations of low
expression in adults43,44. Of relevance to angiogenesis, _TGFB3_ was decreased in a low-EMT model of mouse epicardium while correlating with reduced vascular density of adjacent
myocardium32, and its elevated expression after MI might reduce scarring after injury45. Interestingly, many fetal interactions were from epicardial-specific collagens, such as _COL11A1_ and
_COL9A3_ (Extended Data Fig. 4), which may play an important part in epicardial-mediated matrix reorganization. Another epicardial collagen, _COL3A1_, was also found here but was
upregulated more broadly in fetal hearts when compared with adults and was omitted (Supplementary Table 7). Lastly, the adult epicardium was predicted to communicate with adult endothelial
cells via _EGFR_, a complex and pleiotropic regulator of proliferation and survival of myocardial tissue (Fig. 4e). However, we could not determine if epicardial _EGFR_ was a soluble form.
Other adult epicardial interactions agree with adult immuno-inflammatory focus, with members of the TNF ligand or receptor superfamilies _TNFSF14_ or _TNFRSF11B_, interleukins _IL15_ and
_IL6_ and chemokines _CCL2_ and _CXCL1_ seen to interact with receptors on endothelial cells as well as other cells of the adult or fetal heart. Additionally, adult epicardial _PLA2G2A_
interacting here with integrin complexes has previously been associated with coronary heart disease and infarction46. These results provide evidence that an in situ reactivation of fetal
epicardial programs might increase regenerative communication with endothelial cells to drive angiogenesis and vascularization, which are key processes in cardiac regeneration. HESC-DERIVED
EPICARDIUM CLOSELY RESEMBLES FETAL EPICARDIUM We previously harnessed active epicardium to augment heart regeneration using hESC-EPIs in situ17. However, the mechanisms governing this
therapeutic success were unknown. To address this and identify commonalities between in vitro and in vivo epicardium, we harvested hESC-EPIs during the final 9 days of differentiation47 and
generated an scRNA-seq time course (Fig. 5a,b). This protocol yields a heterogeneous epicardium21, confirmed in our results as a divergent differentiation into two branches, which expressed
either _PODXL_ and _BNC1_ (lineage A) or _TCF21_ and _THY1_ (lineage B) (Fig. 5c,d). To determine how well hESC-EPI models in vivo epicardium, we trained a random forest classifier on the
adult and fetal in vivo heart scRNA-seq high-resolution clusters and found that the number of epicardial predictions increased over differentiation, occurring in lineage A (Fig. 5e,f). In
contrast, lineage B became classified as fibroblasts or fibroblast-like cells (Fig. 5e,f). Performance of our model was assessed using six-fold cross-validation where the non-proliferating
epicardial clusters were predicted with high accuracy (Extended Data Fig. 5). These results reflect the separation of Epicardium_Mesothelial and Epicardium_FB-like populations found in vivo.
Both populations appeared to stem from a population predicted as fetal Immature_FB-like cells (Fig. 5f). We then calculated the mean expression of epicardial gene modules during the
hESC-EPI differentiation and found that angiogenic module D increased throughout, reaching a similar expression to the in vivo fetal epicardium (Fig. 5g). In contrast, adult module A was
absent in hESC-EPIs (Fig. 5g). We also noticed that the expression of the more mesothelial gene module E appeared to be higher in the _PODXL_+ branch A, agreeing with random forest
predictions. We also observed a transiently high initial but decreasing expression of the proliferation-associated gene module B (Fig. 5g). Additionally, we found that the expression of many
epicardial-specific genes increased throughout differentiation, including _TNNT1_, _MGP_, _SPARC_ and _COL9A3_ in module C; _DCN_, _TWIST1_, _TFPI2_, _POSTN_ and _RAMP1_ in module D; and
_CXCL14_, _NRP2_ and _SLIT3_ in module E (Fig. 5g). Lastly, the in vitro model also expressed several epicardial WNT signaling genes, such as _SFRP2_, _WNT2B_ and _TFPI2_, seen more
obviously in higher-depth sequencing data of hESC-EPIs produced following the same protocol21. Conservation of these genes across both in vivo and in vitro systems implies similar epicardial
function in both environments and highlights in vivo pathways that hESC-EPIs may use when augmenting hESC-cardiomyocyte grafts17. EPDCS ARE FOUND WITHIN THE MYOCARDIUM AND SUB-EPICARDIAL
SPACE Lastly, we used immunocytochemistry to spatially resolve fetal epicardial cell populations in a strategy combining new markers from our analysis and _POSTN_ (periostin) highly
expressed in gene module D (Fig. 3d). We performed a uniform manifold approximation and projection (UMAP) pseudostain on the integrated dataset (Fig. 2a) for visualizing combinations of top
epicardial markers in the red and green RGB channels (Fig. 6a) and selected _TM4SF1_ and _PRG4_ for separating Epicardium_FB-like and Epicardium_Mesothelial clusters; _POSTN_ and _DCN_ for
identifying EPDCs; and _KRT19_ and _MSLN_ for the positive selection of EPDCs against fibroblasts or endocardial cells. Our _UPK3B_ stain was ineffective (Extended Data Fig. 6). No
co-localization was observed between _TM4SF1_ or _PRG4_ and _POSTN_ or _DCN_, validating our pseudostain and scRNA-seq analysis (Fig. 6bi,bii). However, co-localization of _POSTN_ was found
with _KRT19_ or _MSLN_ on the epicardium, agreeing with shared fibroblast and epicardial genes seen in the scRNA-seq data (Fig. 6biii,biv). These double-positive cells were found in the
mesothelial layer but remained negative for _PRG4_ or _TM4SF1_ and may be switching state and preparing for EMT (Fig. 6b). Furthermore, we found that _POSTN_+ cells were also sparsely
distributed within the myocardial tissues and sub-epicardial layer, which indicates EPDCs that have lost mesothelial hallmarks. We also analyzed spatial transcriptomics in one fetal heart
aged 9 weeks, 4 days and projected epicardial populations spatially using Cell2Location (Fig. 6c). We validated epicardial spots using markers found in this study (Extended Data Fig. 7) and
also monitored the spatial distribution of epicardial gene modules. However, this was not informative, as modules were generated by comparing between epicardial states, not between
epicardial cells and other cells of the human heart (Extended Data Fig. 7). We found that epicardial clusters were identified on the periphery of the myocardium as expected (Fig. 6c).
However, although the Epicardium_Mesothelial cluster remained in spots on the surface of the heart, the Epicardium_FB-like cluster was enriched in spots deeper within the myocardium (Fig.
6c). Overall, these results provide further spatial evidence that our fibroblast-like epicardium is a transient migratory EPDC population that loses mesothelial identity after EMT, forming a
key part of the developmental and regenerative dynamics absent in adult hearts. DISCUSSION Epicardial activity appears to be an important element of heart regeneration. On the one hand,
active epicardium plays a substantial role in successful cardiac regeneration in adult zebrafish, newts and developing mammalian systems. On the other hand, the epicardium is reportedly
quiescent in adult mammalian and human hearts, which lack regenerative capabilities. However, despite the apparent importance of the epicardium, few studies have yet defined how aging alters
the regenerative programs in human epicardial cells, presenting an opportunity for finding novel therapeutic mechanisms in treating ischemic injury. In addressing this unexplored space, we
combined and compared fetal and adult hearts from humans at single-cell resolution, to our knowledge for the first time, and focused on epicardial cells within them. We revealed both
compositional and molecular differences between the adult and fetal epicardium that, in part, may underpin the limited regeneration seen in adult human hearts. We found that the adult
epicardium (1) has a limited population of mesenchymal EPDCs; (2) has reduced paracrine communication; (3) lacks fetal-specific regenerative and angiogenic epicardial gene programs; and (4)
is more primed for response to immune stimuli. This is the first time that human EPDC transcriptomes have been described at a single-cell resolution, as determined by their expression of
mesenchymal genes _TWIST1_ and _SPARC_ as well as the combined expression of known epicardial and fibroblast genes. These characteristics are shared with cell populations found in developing
mouse and chick hearts19,32. Additionally, the position of these cells was consistent with migrating EPDCs using immunohistochemistry using antibodies for _POSTN_, _TM4SF1_ and established
epicardial markers. A major finding of our study was the lack of EPDCs in adult human cardiac tissue. This might be explained by quiescent adult epicardial cells being less responsive to
EMT-driving stimuli as previously demonstrated in cultured adult EPDCs48. Current knowledge suggests that epicardial cells undergo EMT, forming EPDCs, which subsequently differentiate into
other cardiac cell types3,4, resulting in a departure from epicardial cell states. Therefore, we hypothesize that fewer migrating adult epicardial cells results in a reduced quantity of
transient EPDCs at steady state due to differentiation or efflux from the transient EPDC cell type. Our results agree with current understanding of adult epicardial quiescence, and, although
experiments have shown that adult epicardial cells may be pro-regenerative when transplanted, these cells are either primed or likely primed during culture conditions14,49. Our study is the
first exhaustive documentation of the age-associated loss of epicardial signals involved in angiogenesis, proliferation and survival in healthy non-primed adult epicardium. For human heart
regeneration, one strategy is to restore epicardial activity by reverting the adult epicardium to fetal states or by administering active epicardial cells generated from pluripotent stem
cells47. Our study provides a roadmap for this translational effort, as, for the first time, we now have a transcriptome-wide description of the ingredients required to bring fetal-like
regenerative function back into adult epicardium. First, the regenerative human epicardium may drive angiogenesis through _NRP2_, _VEGFA_, _CXCL14_ and _SLIT3_ with adult endothelial cells;
new vessel growth is likely sourced from pre-existing endothelial cells50,51. Angiogenesis resulting from these interactions has been confirmed in mice where epicardial SLIT2-mediated
co-localization with _ROBO4_-expressing endothelial cells was essential for vascularization32 and may also be important for angiogenesis in human tissue51. Furthermore, _SLIT_/_ROBO_
signaling may also involve epicardial _CXCL12_/_CXCR4_ (refs. 52, 53) in an interaction that may include the early epicardial-specific gene and allosteric _CXCR4_ and _CXCL14_.
Interestingly, _CXCL14_ has not been found in animal epicardium to date and may be a key difference between animal and human epicardial signaling. Second, we should also aim to reactivate
paracrine WNT signaling, including _SFRP_s _2_ and _5_, _RSPO1_ and _WNT2B_. Previous animal studies showed high _SFRP2_ expression during cardiogenesis and regeneration with anti-fibrotic
properties, specifically in post-injury epicardium25,31,54. However, _SFRP5_ has not been seen in animal studies and may be more relevant in humans. Studies found that _SFRP5_ was inversely
proportional to cardiovascular disease risk factors, positively correlated with faster recovery after MI and seen to protect against re-perfusion injury26,55,56. Although the local targets
of these WNT proteins may be unknown, evidence suggests that _WNT2B_ may increase proliferation in cardiomyocytes and fibroblasts27,57 and that restoring these elements of WNT signaling may
be key to adult heart regeneration. Other vital epicardial ingredients involve extracellular matrix remodeling, proliferation and survival of myocardial tissue driven by _TGFB3_, _BMP3_,
_RSPO1_ (ref. 28) and a variety of epicardial-specific collagens, such as _COL11A1_ (ref. 58). Lastly, we demonstrated that hESC-EPIs contain many of these ingredients and have proven
effectiveness in animal model grafts17, giving confidence in this recipe, and that bringing fetal programs back into adult epicardium is a viable strategy for adult human heart regeneration.
A surprising result was the focus of adult epicardium on response to immune and external stimuli, which may be an undiscovered age-associated element of normal epicardial aging. This
observation adds complexity and further weight to proper understanding of immune response regulation in cardiac regeneration as noted in experimental evidence in mice where a rapid transient
immune response is key for proper regeneration11. This disparity between fetal and adult response programs places the epicardium further still as a key mediator of the immune response in
cardiac regeneration with a coordinated age-associated upregulation of genes. Further still, this suggests that aging may elevate epicardial immuno-sensitivity as opposed to elevating the
stimulatory abilities of immune cells. Lastly, our analysis also suggests a component of programmed aging that governs the loss of pro-regenerative functions with upregulated genes, such as
_PLA2G2A_59 or _TNFSF14_ (ref. 60). These genes have been implicated in inhibiting tumor angiogenesis and migration, which opens a discussion on what elements require deactivation as well as
reactivation to produce a pro-regenerative epicardium. It is important to note that our study did not capture adult hearts from a diseased population but, instead, focussed on the healthy
state. Therefore, we could not compare the active fetal epicardium to injury-reactivated epicardium. We consider that these fetal programs could also become expressed in the
injury-reactivated adult epicardium. For example, _SFRP2_ and _SLIT3_ are expressed in adult mice after injury31. Indeed, one experiment in neonatal mice revealed an increase in _RSPO1_ in
the regenerative P1 but not in non-regenerative P7 hearts after MI12. This forms one independent validation of healthy-state adults as a model of non-regenerative epicardium in humans, as
_RSPO1_ was also decreased in our adult epicardium. In our analysis, we grouped epicardial cells from multiple heart regions where there may be region-specific cellular compositions1.
However, this is unlikely to affect the main biological comparison. On a final note, it is incorrect to assume that the entire regenerative capacity of the heart rests upon the active
epicardium; other cells also play a major role in regeneration. Addressing this comprehensively is beyond the scope of this study. However, our integrated dataset may also be used for future
tissue-targeted and organ-wide studies on the age-associated changes in the human heart. The next step for clinical translation is to disentangle the gene networks that regulate adult and
fetal epicardial states. In doing so, we might identify the molecular switches required to revert the adult epicardium into a fetal state and restore these key pathways. Finally, because we
have detailed both active and inactive states of the human epicardium, benchmarked cross-species epicardial markers in humans and shown that stem-cell-derived epicardium contains
regenerative epicardial programs, this study serves as a valuable roadmap toward reactivating the adult epicardium and promoting heart regeneration in adult humans. METHODS ADULT DATA
COLLECTION The unique molecular identifier (UMI) counts matrix for 486,134 adult heart cells or nuclei was acquired from the Heart Cell Atlas accessing the full version of the h5ad formatted
dataset22. We then subset this matrix to retain only the six donors (D2–D7) with at least three cells annotated as ‘meso’ in the available ‘cell_state’ metadata, leaving 190,515 nuclei or
cells. FETAL SAMPLE COLLECTION Fetuses were obtained after elective termination of pregnancy with full consent (approved by the ethics committee of NHS East of England LREC no. 96/085) and
stored overnight in Hibernate-A Medium (Gibco) at 4 °C. The next day after collection, the apex and base of each heart was dissected and dissociated61. In brief, tissue was dissociated using
6.6 mg ml−1 of _Bacillus licheniformis_ protease, 5 mM CaCl2 and 20 U ml−1 of DNase I, where the mixture was triturated on ice for 20 seconds every 5 minutes until clumps of tissue were no
longer visible. The digestion was stopped with ice-cold 10% FBS in PBS. For sample F5, the apex and peeled epicardium was incubated with Liberase for 30 minutes, followed by washes. Cells
were then washed with 10% FBS, resuspended in 1 ml of PBS and viability assessed using Trypan blue. Cells were submitted for 10x library preparation for 3′ single-cell sequencing on a
NovaSeq 6000 (Illumina) using V3 chemistry at the Cancer Research UK (CRUK) Cambridge Institute. Sample F5 was collected as a pilot sample and prepared separately from the other fetal
samples, and only the apex attached to a careful epicardial peeling was taken and dissociated. Sample F5 was sequenced independently at the Sanger Institute using HiSeq 4000 (Illumina).
HESC-EPI DIFFERENTIATION AND COLLECTION Differentiation of epicardium was carried out according to our previously published protocols47. In brief, H9-hESCs (WiCell) were initially
differentiated into lateral plate mesoderm (LM) in the presence of _FGF2_ and _BMP4_. The LM is then exposed to _WNT3A_, _BMP4_ and retinoic acid, resulting in hESC-EPIs after 8–9 days.
Cells were harvested on days 1, 2, 3, 4, 8 and 9 of differentiation after the LM stage by re-suspension in PBS. Samples were submitted for 10x library preparation for 3′ single-cell
sequencing at the CRUK Cambridge institute. RNA-SEQ PRE-PROCESSING AND PROCESSING For fetal samples F1, F2, F3, F4, F6 and F7, demultiplexing, cell calling, alignment and counts matrix
generation were carried out using Cell Ranger version 6.1. For our pilot sample F5, an earlier version of Cell Ranger was used. For the hESC-EPI samples, Cell Ranger version 3.02 was used.
All samples were aligned against the human reference genome GRCh38 using default parameters. After the counts matrices were generated, all fetal samples were treated the same. Poor-quality
cells were removed from the read counts matrices in R, retaining only cells with a depth of between 1,000 and 15,000 UMIs, expression of over 400 genes or fraction of mitochondrial genes
under 15%. These thresholds were chosen following the boundaries of the adult dataset. Doublets in fetal datasets were called using Scrublet62 on the UMI matrices after alignment, with an
expected doublet detection rate of 0.06. Erythrocyte contamination was seen in fetal samples, and erythrocyte-containing barcodes were identified using a two-compartment Gaussian mixture
mode on the mean expression of hemoglobin genes in each sample. Fetal samples were then integrated and clustered. Clusters containing over 50% of the erythrocyte compartment were removed
(Extended Data Fig. 1). For adult samples, pre-processing steps were not repeated as they were carried out before downloading the data. Finally, genes not expressed in any cell were removed
from further analysis, leaving 27,956 gene features. STRATIFIED SAMPLING OF DATASETS Adult data were subsampled across mixed stratifications of ‘cell_state’, ‘donor’ and ‘cell_source’
annotations after data acquisition before any analysis. To do this, we built an algorithm using a single parameter _x_ to control the sampling rate across _K_ clusters of size _N__K_, where
_x_ is the approximate sample size to take from each cluster _K_. Iteratively, our algorithm randomly sampled cells from each donor in each cluster _K_ without replacement until the number
of cells in the newly sampled cluster, _k_, exceeded _x_ (_n__k_ > _x_). For cases where _N__K_ < _x_, the number of sampled cells is equal to the cluster size (_n__k_ = _N__K_),
effectively sampling all available cells and donors. This method was chosen to maximize minority cluster representation in the combined dataset while reducing source and donor biases. In the
adult data, we aimed to subsample each ‘cell_state’ to the total number of epicardial cells (_n_ = 717) in a two-stage sampling-stratified strategy. First, adult cells were sampled from
‘cell_state’ evenly distributed across ‘donor’ until each ‘cell_state’ consisted of at least the number of ‘Meso’-labeled nuclei (_x__cells_ = 597). Second and similarly, adult nuclei were
sampled from ‘cell_state’ evenly distributed across ‘donor’ until 597 nuclei were sampled from each ‘cell_state’ annotation (_x__nuclei_ = 597). Finally, these newly sampled cells and nuclei
were combined, and then the barcodes were re-sampled to the size of all ‘Meso’-annotated barcodes in the ‘cell_state’ annotation (Meso-labeled adult barcodes, _x__adult_ = 717) to create
evenly distributed groups of cell source, donor and cell type with a maximum similar cell type quantity to the number of epicardial cells (Extended Data Fig. 1). To sample cells from the
fetal datasets before integration, we first created new cell type stratifications. To do this, fetal samples were integrated using Seurat’s RPCA pipeline63. Following the established
vignette, each fetal sample was log-transformed and scaled before integrating. After PCA, nearest neighboring cells were calculated using integrated distances, and fetal cells were clustered
using the Louvain method of community detection (cells = 47,473, neighbors = 20, resolution = 0.5). This resulted in 21 cluster-based stratifications that were then sampled evenly across
donors to achieve the size of the putative epicardial cell population identified using canonical epicardial markers (fetal epicardial cells, _x__fetal_ = 1,598) (Extended Data Fig. 1). The
clustering parameters were chosen such that subsequent sampling of the number of epicardial cells from each cluster will result in a balance of adult and fetal cells in the sampled dataset
(adult cells = 29,779, fetal cells = 30,889). We included all fetal epicardial cells with the aim of retaining the maximum information available for this uncommon cell population.
INTEGRATION OF ADULT AND FETAL DATA Raw UMI counts matrices of subsampled adult and fetal datasets were combined following Seurat’s RPCA integration pipeline63. In brief, we defined 24 new
integration groups within the dataset combining the unique combinations of ‘cell_source’ and ‘donor’ annotations. Each integration group was then log-transformed and scaled individually, and
variable features were identified, followed by PCA. We selected 2,806 anchor genes for integration as found to be variable in at least 25% of the integration groups. Additionally, to
perform our integration, we defined a hierarchical sample tree for integrating these 24 groups, which prioritized (1) the donor-matched integration of adult nuclei into adult cells and fetal
base into apex, followed by (2) integration between fetal donors and then (3) integration of all adult data into fetal data (Extended Data Fig. 1). We chose this method as our samples
consisted of different sequencing samples or data sources from the same donor (four sources: nuclei, cell, apex and base). Clustering across the integrated dataset was performed using the
Louvain algorithm in Seurat, giving ten distinct cell types (resolution = 0.1, _k_ neighbors = 20). Each cell type cluster was then similarly and separately subclustered, giving 56
high-resolution clusters. Then, to represent the data over multiple cell type and state granularities, we aggregated these high-resolution clusters together hierarchically by joining
biologically similar cell types together at decreasing resolutions until we arrived at the initial low-resolution clustering (Extended Data Fig. 2). EPICARDIAL MARKERS AND EPICARDIAL MARKERS
LIBRARY A stage-separated epicardial marker analysis was performed in parallel using the fetal and adult datasets after annotation with the newly defined stage-independent clusters in
Seurat with a one-cluster-versus-all strategy on log-transformed counts across clusters of resolutions 1 and 2. We used Wilcoxon rank-sum tests and applied thresholds of _P_ < 1 × 10−10
and absolute log2 fold change > 0.5 throughout the study where significance scores were adjusted for multiple comparisons using Bonferroni correction. In Fig. 1, we reported resolution 1
results comparing the broader gene expression of epicardial cells with all other cell types without resolving epicardial subclusters. Additionally, two lists of unwanted variation genes were
created using differential expression analyses. The first list compared all adult cells and all fetal cells to capture a non-specific age-associated gene list of six adult genes and 92
fetal genes. The second list compared adult cells and adult nuclei to capture the genes associated with the nuclei ‘cell_source’, identifying 69 nuclei genes. These genes were omitted from
the results and resulted in 633 and 724 epicardial genes at clustering resolutions 1 and 2 (unique from epicardial subclusters), respectively. The 724 resolution 2 marker genes were then
scored using the number of gene-positive cells in each group of fetal-epicardial, adult-epicardial or non-epicardial cells where, for each gene and for each group, we identified its
precision (analogous to specificity) determined by the fraction of positive cells that belong to the group; recall (analogous to sensitivity), as the fraction of group cells that were
positive; and the _F_-score, calculated as the harmonic mean between recall and precision (_F__1_ = 2 _×_ (recall × precision) / (recall + precision)). To further enrich this library
distinguishing between epicardial cell types ‘FB-like’ and ‘Mesothelial’, we restricted the dataset to _UPK3B_+ cells as _UPK3B_. This was necessary as there were no clear single markers
that separated the EPDCs from other cells of the heart as well as epicardial cells. Using _UPK3B_+ cells only, we then re-calculated recall, precision and _F_-scores of the epicardial genes
using the groups of ‘FB-like’ and ‘Mesothelial’. This generated a list of differential markers within the _UPK3B_+ population of cells to discern between potential EPDCs and mesothelial
epicardium. Then, for each group of ‘Fetal’, ‘Shared’, ‘Adult’, ‘Mesothelial’ or ‘EPDC’ cells, genes were re-ordered by subtracting the _F_-score for other groups from the _F_-score for all
groups. This resulted in a per-group ordering of genes by selectivity. LIGAND RECEPTOR ANALYSIS WITH CELLPHONEDB The combined adult and fetal counts matrix was transformed into counts per
million (CPM) as recommended and subsequently log2-transformed in the CellPhoneDB statistical analysis pipeline using the curated interactions database (version 2.0)64. For this analysis,
the adult epicardial subclusters of Adult_Epicardium_Proliferating and Adult_Epicardium_FB-like were omitted as there were fewer than three cells in each (_n_ = 0 and 2, respectively). The
numbers of significant interactions for the remaining epicardial clusters were then counted in either adult or fetal stages (_P_ < 0.05) and visualized in an ordered heat map. The results
from CellPhoneDB were filtered in R, retaining only epicardial-specific markers identified in the parallel marker analysis. This was further filtered for putative secreted protein-coding
genes using the Human Protein Atlas as a reference. GENE–GENE CO-OCCURRENCE AND EPICARDIAL GENE MODULE CONSTRUCTION Cells annotated as epicardium after clustering were isolated in a new
matrix, and a set of epicardial features for clustering was selected using differential expression analysis between adult and fetal epicardial cells (Wilcoxon rank-sum test; _P_ < 1 ×
10−10, log2 fold change > 0.5) (Supplementary Table 10). After removing the previously identified control genes, the epicardial matrix of 1,912 cells across 1,594 genes was binarized,
counting positive expression as a value of at least 1. For gene module construction, this matrix was further subset to omit nuclei barcodes, as gene clustering was shown to be affected
largely by their expression in nuclei or cells, giving a binary matrix of 1,315 cells and 1,594 genes. However, nuclei were added back into the matrix after module construction, and we
observed that nuclei largely retained gene module patterns, independently validating our results. We implemented the approach by Qiu (ref. 65) in R to cluster gene-dropout patterns. In
brief, we calculated the co-occurrence of each gene pair across all cells with a modified chi-square statistic. Then, for each gene pair, all chi-square statistics below a given threshold
were discarded to retain only the high-scoring gene–gene pairs. This threshold was calculated using random permutations of the data. An undirected graph of highly concerted genes was then
formed from the remaining gene pairs, weighted by the chi-square statistics and adjusted by a Jaccard index. Finally, this gene–gene graph was clustered using a conservative Louvain method
for community detection, removing all clusters with fewer than 20 genes. Genes in resulting gene modules were ordered by their mean chi-square value with other genes in the module, ranking
the genes by pattern specificity. New gene module commitment features for all epicardial cells, including nuclei, were calculated by the mean of binarized expression of each gene module, and
a PCA was carried out. We represented components 2 and 4, as component 1 was technical noise, correlating with library size and source (Kendall rank correlation, _P_ < 0.05) (Fig. 3b and
Extended Data Fig. 3), whereas components 2 and 4 correlated with cell type and age and were orthogonally represented in the PCA. The Louvain method for community detection was used to
generate epicardial states, and states were ordered by age using their mean of PCA component 2, which highly correlated with the sample-ranked age of each cell (Kendall’s tau = _−_0.67, _P_
< 0.01, _n_ = 1,912). Gene set overrepresentation analysis of Gene Ontology terms across gene modules was carried out using the R package gprofiler2 (ref. 66) against a background of all
expressed genes in epicardial cells. HESC-EPI ANALYSIS AND CLASSIFICATION After pre-processing of the hESC-EPI data, 300 cells were randomly sampled in silico from each timepoint. The
sampled dataset of 1,800 cells was log-transformed, subjected to PCA and projected into two UMAP dimensions using Seurat. The Louvain method of community detection was then used to cluster
cells, and the clusters were annotated into either of previously identified lineages based on marker expression. To classify the hESC-EPIs, a random forest classification model was trained
on the in vivo dataset to discern among 34 cell types in either adult or fetal stages from clustering resolution 3 (Extended Data Fig. 4). In training and testing the random forest
classifier, raw UMI matrices were processed in an experiment-independent manner, and cells were adjusted for library size using CPM and transformed using log2 with a pseudocount of 1.
Features selected for the model were the top 50 differentially expressed marker genes from each cluster in the resolution 2 clusters from the stage-separated analysis (Supplementary Table
2), giving a total of 1,445 unique features. The model was constructed on a sample of 33% of cells and assessed using six-fold cross-validation and independently validated on the remaining
66% of cells (Extended Data Fig. 5). The sampled hESC-EPI dataset of 1,800 cells was then predicted using the model trained on the 33% cell fraction. IMMUNOFLUORESCENT STAINING Fetal hearts
were collected from donors BRC2281 and BRC2375, aged 9 weeks and 10 weeks, 3 days, respectively, and were dissected and fixed in 4% paraformaldehyde (Alfa Aesar) overnight with gentle
rocking at 4 °C. Then, cryoprotection was done in 30% sucrose (Sigma/Merck) for a further 24 hours at 4 °C. Tissues were embedded in OCT (Sakura Tek) and frozen on dry ice. Then, 10-µm
sections were cut serially using a Leica cryostat. Sections were air dried for at least 10 minutes before storage at −80 °C. Immunofluorescent staining was carried out by thawing the slides
for 10 minutes and rehydrating with tris-buffered saline (TBS) for a further 10 minutes at room temperature. The tissue was permeabilized for 10 minutes in a permeabilization buffer made up
of 0.25% saponin in TBS, followed by a 5-minute wash with 0.2% Tween 20 in TBS. A 0.3 M glycine in antibody dilution blocking buffer was applied for 1 hour. Then, this solution was decanted,
and the primary antibody solution was applied overnight at 4 °C (all primary antibodies were used at a dilution of 1:100). The next day, the tissue was subjected to three 5-minute washes,
and the secondary antibody solution was applied (all secondary antibodies were used at a dilution of 1:1,000) for 1 hour at room temperature. The secondary antibody solution was washed for 5
minutes a following two times, and a DAPI solution was added at a diluation of 1:2,000 in TBS for 15 minutes at room temperature. This was washed for 5 minutes. To finish the process,
VectaShield was applied and a coverslip attached. Each tissue was left for at least 2 hours before imaging. The primary antibodies used in this study for immunofluorescent imaging included:
_UPK3B_, PA552696 (Themo Fisher Scientific); _MSLN_, sc33672 (Santa Cruz Biotechnology); _KRT19_, sc6278 (Santa Cruz Biotechnology); _POSTN_, MAB3548 (R&D Systems); _DCN_, AF143 (R&D
Systems); _PRG4_, MABT400 (Sigma-Aldrich); and _TM4SF1_, MAB8164 (R&D Systems). The secondary antibodies used included: Alexa Fluor 488 donkey anti-rabbit A21206 (Invitrogen) for
_UPK3B_; Alexa Fluor 647 goat anti-mouse A21240 (Invitrogen) for MSLN, KRT19 and TM4SF1; Alexa Fluor 568 goat anti-rat A11077 (Invitrogen) for POSTN; Alexa Fluor 647 chicken anti-mouse
A21463 (Invitrogen) for PRG4; and Alexa Fluor 568 donkey anti-goat A11057 (Invitrogen) for DCN. A hydrophobic pen was used throughout the staining process to surround the tissue. VISIUM
SLIDE AND LIBRARY PREPARATION FOR SPATIAL TRANSCRIPTOMICS AND CELL2LOCATION A single heart, with a gestational age of 9 weeks and 4 days was frozen and embedded in OCT medium using a
dry-ice-cooled bath of isopentane. OCT-embedded samples were sectioned using a cryostat (Leica, CX3050S) and cut at 10 μm. RNA integrity number (fresh-frozen samples) was obtained using an
Agilent 2100 Bioanalyzer. The Tissue Optimization protocol from 10x Genomics was performed to obtain a permeabilization time of 35 minutes, and the Visium Spatial Gene Expression experiment
was performed as per the manufacturer’s protocol (10x Genomics). Hematoxylin and eosin (H&E)-stained Visium Gene Expression slides were imaged at ×40 on a Hamamatsu NanoZoomer S60. After
transcript capture, Visium Library Preparation Protocol from 10x Genomics was performed. The cDNA library was diluted to a final concentration of 2.25 nM (200 μl volume) and sequenced on 2×
SP flow cells of Illumina NovaSeq 6000. Space Ranger (version 1.1.0, 10x Genomics) was used for the read-mapping to the human reference genome (GRCh38) with default parameters. Anatomical
microstructures were manually annotated using the paired histology H&E image. To map clusters onto Visium results, we used Cell2Location67. In brief, Cell2Location first estimates
reference signatures of cell types obtained from the scRNA-seq data using a negative binomial regression model. Then, the abundance of each cell type is calculated in each Visium spot by
decomposing spot mRNA counts using the cell type signatures. A spot resolution hyperparameter was estimated using H&E-stained images of the Visium slides, resulting in 20 cells per spot
for parameterizing the Cell2Location pipeline. ETHICS STATEMENT Collection of human fetuses for this study from anonymous female donors was approved by NHS East of England under LREC no.
96/085. Full informed consent was given by all donors after elective termination of pregnancy. Donors were made aware of the possible use of donated fetuses, and no financial compensation
was given. Donors were free to withdraw consent at any time. REPORTING SUMMARY Further information on research design is available in the Nature Portfolio Reporting Summary linked to this
article. DATA AVAILABILITY Both raw and processed RNA sequencing data generated during this study can be found in the Gene Expression Omnibus using the accession identifiers GSE216019 (fetal
scRNA-seq data) and GSE216177 (hESC-EPI differentiation). Matrices of adult heart scRNA-seq data are available from the Heart Cell Atlas27 and can be accessed at
https://www.heartcellatlas.org/#DataSources. Specifically, the adult data file accessed was downloaded here: https://cellgeni.cog.sanger.ac.uk/heartcellatlas/data/global_raw.h5ad. Finally,
the integrated data combining both adult and fetal data may be explored interactively at http://sinha.stemcells.cam.ac.uk/. The human reference genome (GRCh38) is available at
https://cf.10xgenomics.com/supp/cell-exp/refdata-gex-GRCh38-2020-A.tar.gz. CODE AVAILABILITY The R code and reports generated during this analysis are freely available from GitHub
(https://github.com/Hindrance/Adult_Foetal_Epicardial_Comparison_2022). CHANGE HISTORY * _ 11 APRIL 2023 A Correction to this paper has been published:
https://doi.org/10.1038/s44161-023-00269-z _ REFERENCES * Risebro, C. A., Vieira, J. M., Klotz, L. & Riley, P. R. Characterisation of the human embryonic and foetal epicardium during
heart development. _Development_ 142, 3630–3636 (2015). CAS PubMed PubMed Central Google Scholar * Krainock, M. et al. Epicardial epithelial-to-mesenchymal transition in heart
development and disease. _J. Clin. Med._ 5, 27 (2016). Article PubMed PubMed Central Google Scholar * Dettman, R. W., Denetclaw, W., Ordahl, C. P. & Bristow, J. Common epicardial
origin of coronary vascular smooth muscle, perivascular fibroblasts, and intermyocardial fibroblasts in the avian heart. _Dev. Biol._ 193, 169–181 (1998). Article CAS PubMed Google
Scholar * Katz, T. C. et al. Distinct compartments of the proepicardial organ give rise to coronary vascular endothelial cells. _Dev. Cell_ 22, 639–650 (2012). Article CAS PubMed PubMed
Central Google Scholar * Carmona, R. et al. The embryonic epicardium: an essential element of cardiac development. _J. Cell. Mol. Med._ 14, 2066–2072 (2010). Article CAS PubMed PubMed
Central Google Scholar * Lepilina, A. et al. A dynamic epicardial injury response supports progenitor cell activity during zebrafish heart regeneration. _Cell_ 127, 607–619 (2006). Article
CAS PubMed Google Scholar * González-Rosa, J. M., Martín, V., Peralta, M., Torres, M. & Mercader, N. Extensive scar formation and regression during heart regeneration after
cryoinjury in zebrafish. _Development_ 138, 1663–1674 (2011). Article PubMed Google Scholar * Bryant, D. M. et al. A systematic analysis of neonatal mouse heart regeneration after apical
resection. _J. Mol. Cell. Cardiol._ 79, 315–318 (2015). Article CAS PubMed Google Scholar * Haubner, B. J. et al. Functional recovery of a human neonatal heart after severe myocardial
infarction. _Circ. Res._ 118, 216–221 (2016). Article CAS PubMed Google Scholar * Ye, L. et al. Early regenerative capacity in the porcine heart. _Circulation_ 138, 2798–2808 (2018).
Article PubMed Google Scholar * Wang, Z. et al. Mechanistic basis of neonatal heart regeneration revealed by transcriptome and histone modification profiling. _Proc. Natl Acad. Sci. USA_
116, 18455–18465 (2019). Article CAS PubMed PubMed Central Google Scholar * Wang, Z. et al. Cell-type-specific gene regulatory networks underlying murine neonatal heart regeneration at
single-cell resolution. _Cell Rep._ 33, 108472 (2020). Article CAS PubMed PubMed Central Google Scholar * Zhou, B. et al. Adult mouse epicardium modulates myocardial injury by secreting
paracrine factors. _J. Clin. Invest._ 121, 1894–1904 (2011). Article CAS PubMed PubMed Central Google Scholar * Smart, N. et al. Myocardial regeneration: expanding the repertoire of
thymosin β4 in the ischemic heart. _Ann. N Y Acad. Sci._ 1269, 92–101 (2012). Article CAS PubMed PubMed Central Google Scholar * Virag, J. A. et al. Fibroblast growth factor-2 regulates
myocardial infarct repair: effects on cell proliferation, scar contraction, and ventricular function. _Am. J. Pathol._ 171, 1431–1440 (2007). Article CAS PubMed PubMed Central Google
Scholar * Villa Del Campo, C. et al. Regenerative potential of epicardium-derived extracellular vesicles mediated by conserved miRNA transfer. _Cardiovasc. Res._ 118, 597–611 (2021).
Article Google Scholar * Bargehr, J. et al. Epicardial cells derived from human embryonic stem cells augment cardiomyocyte-driven heart regeneration. _Nat. Biotechnol._ 37, 895–906 (2019).
Article CAS PubMed PubMed Central Google Scholar * Braitsch, C. M., Combs, M. D., Quaggin, S. E. & Yutzey, K. E. Pod1/Tcf21 is regulated by retinoic acid signaling and inhibits
differentiation of epicardium-derived cells into smooth muscle in the developing heart. _Dev. Biol._ 368, 345–357 (2012). Article CAS PubMed PubMed Central Google Scholar * Mantri, M.
et al. Spatiotemporal single-cell RNA sequencing of developing chicken hearts identifies interplay between cellular differentiation and morphogenesis. _Nat. Commun._ 12, 1771 (2021). Article
CAS PubMed PubMed Central Google Scholar * Weinberger, M., Simões, F. C., Patient, R., Sauka-Spengler, T. & Riley, P. R. Functional heterogeneity within the developing zebrafish
epicardium. _Dev. Cell_ 52, 574–590 (2020). Article CAS PubMed PubMed Central Google Scholar * Gambardella, L. et al. BNC1 regulates cell heterogeneity in human pluripotent stem
cell-derived epicardium. _Development_ 146, dev174441 (2019). Article CAS PubMed PubMed Central Google Scholar * Litviňuková, M. et al. Cells of the adult human heart. _Nature_ 588,
466–472 (2020). Article PubMed PubMed Central Google Scholar * Lupu, I.-E., Redpath, A. N. & Smart, N. Spatiotemporal analysis reveals overlap of key proepicardial markers in the
developing murine heart. _Stem Cell Rep._ 14, 770–787 (2020). Article CAS Google Scholar * Suryawanshi, H. et al. Cell atlas of the foetal human heart and implications for
autoimmune-mediated congenital heart block. _Cardiovasc. Res._ 116, 1446–1457 (2020). Article CAS PubMed Google Scholar * Wu, Y. et al. Multiple roles of sFRP2 in cardiac development and
cardiovascular disease. _Int. J. Biol. Sci._ 16, 730–738 (2020). Article CAS PubMed PubMed Central Google Scholar * Nakamura, K. et al. Secreted frizzled-related protein 5 diminishes
cardiac inflammation and protects the heart from ischemia/reperfusion injury. _J. Biol. Chem._ 291, 2566–2575 (2016). Article CAS PubMed Google Scholar * Peng, X. et al. _Wnt2bb_ induces
cardiomyocyte proliferation in zebrafish hearts via the _jnk1/c-jun/creb1_ pathway. _Front. Cell Dev. Biol._ 8, 323 (2020). Article PubMed PubMed Central Google Scholar * Li, G. et al.
Single cell expression analysis reveals anatomical and cell cycle-dependent transcriptional shifts during heart development. _Development_ 146, dev173476 (2019). Article CAS PubMed PubMed
Central Google Scholar * Khosravi, F., Ahmadvand, N., Bellusci, S. & Sauer, H. The multifunctional contribution of FGF signaling to cardiac development, homeostasis, disease and
repair. _Front. Cell Dev. Biol._ 9, 672935 (2021). Article PubMed PubMed Central Google Scholar * Barker, H. et al. Role of carbonic anhydrases in skin wound healing. _Exp. Mol. Med._
49, e334 (2017). Article CAS PubMed PubMed Central Google Scholar * Hesse, J. et al. Single-cell transcriptomics defines heterogeneity of epicardial cells and fibroblasts within the
infarcted murine heart. _eLife_ 10, e65921 (2021). Article CAS PubMed PubMed Central Google Scholar * Quijada, P. et al. Coordination of endothelial cell positioning and fate
specification by the epicardium. _Nat. Commun._ 12, 4155 (2021). Article CAS PubMed PubMed Central Google Scholar * Alissa, E. M., Al-Salmi, M. M., Alama, N. A. & Ferns, G. A. Role
of omentin-1 and C-reactive protein in obese subjects with subclinical inflammation. _J. Clin. Transl. Endocrinol._ 3, 7–11 (2016). PubMed Google Scholar * Cui, Y. et al. Single-cell
transcriptome analysis maps the developmental track of the human heart. _Cell Rep._ 26, 1934–1950 (2019). Article CAS PubMed Google Scholar * Park, D. S. J. et al. Human pericardial
proteoglycan 4 (lubricin): implications for postcardiotomy intrathoracic adhesion formation. _J. Thorac. Cardiovasc. Surg._ 156, 1598–1608 (2018). Article PubMed Google Scholar * Hamm, M.
J., Kirchmaier, B. C. & Herzog, W. Sema3d controls collective endothelial cell migration by distinct mechanisms via nrp1 and PlxnD1. _J. Cell Biol._ 215, 415–430 (2016). Article CAS
PubMed PubMed Central Google Scholar * Bonet, F., Inácio, J. M., Bover, O., Añez, S. B. & Belo, J. A. CCBE1 in cardiac development and disease. _Front. Genet._ 13, 836694 (2022).
Article CAS PubMed PubMed Central Google Scholar * Kang, J. et al. The emerging role of EGFL6 in angiogenesis and tumor progression. _Int. J. Med. Sci._ 17, 1320–1326 (2020). Article
CAS PubMed PubMed Central Google Scholar * Kolluri, A. & Ho, M. The role of glypican-3 in regulating Wnt, YAP, and hedgehog in liver cancer. _Front. Oncol._ 9, 708 (2019). Article
PubMed PubMed Central Google Scholar * Rossignol, M., Gagnon, M. L. & Klagsbrun, M. Genomic organization of human neuropilin-1 and neuropilin-2 genes: identification and distribution
of splice variants and soluble isoforms. _Genomics_ 70, 211–222 (2000). Article CAS PubMed Google Scholar * Roy, S. et al. Multifaceted role of neuropilins in the immune system:
potential targets for immunotherapy. _Front. Immunol._ 8, 1228 (2017). Article PubMed PubMed Central Google Scholar * Descamps, B. et al. Frizzled 4 regulates arterial network
organization through noncanonical Wnt/planar cell polarity signaling. _Circ. Res._ 110, 47–58 (2012). Article CAS PubMed Google Scholar * Molin, D. G. et al. Expression patterns of
_Tgfβ1–3_ associate with myocardialisation of the outflow tract and the development of the epicardium and the fibrous heart skeleton. _Dev. Dyn._ 227, 431–444 (2003). Article CAS PubMed
Google Scholar * Doetschman, T. et al. Transforming growth factor beta signaling in adult cardiovascular diseases and repair. _Cell Tissue Res._ 347, 203–223 (2012). Article CAS PubMed
Google Scholar * Xue, K. et al. The role and mechanism of transforming growth factor beta 3 in human myocardial infarction-induced myocardial fibrosis. _J. Cell. Mol. Med._ 23, 4229–4243
(2019). Article CAS PubMed PubMed Central Google Scholar * Shuvalova, Y. A. et al. The association of _PLA2G2A_ single nucleotide polymorphisms with type IIa secretory phospholipase A2
level but not its activity in patients with stable coronary heart disease. _Gene_ 564, 29–34 (2015). Article CAS PubMed Google Scholar * Iyer, D. et al. Robust derivation of epicardium
and its differentiated smooth muscle cell progeny from human pluripotent stem cells. _Development_ 142, 1528–1541 (2015). CAS PubMed PubMed Central Google Scholar * Moerkamp, A. T. et
al. Human fetal and adult epicardial-derived cells: a novel model to study their activation. _Stem Cell Res. Ther._ 7, 174 (2016). Article PubMed PubMed Central Google Scholar * Winter,
E. M. et al. Preservation of left ventricular function and attenuation of remodeling after transplantation of human epicardium-derived cells into the infarcted mouse heart. _Circulation_
116, 917–927 (2007). Article CAS PubMed Google Scholar * He, L. et al. Preexisting endothelial cells mediate cardiac neovascularization after injury. _J. Clin. Invest._ 127, 2968–2981
(2017). Article PubMed PubMed Central Google Scholar * Paul, J. D. et al. SLIT3-ROBO4 activation promotes vascular network formation in human engineered tissue and angiogenesis in vivo.
_J. Mol. Cell. Cardiol._ 64, 124–131 (2013). Article CAS PubMed Google Scholar * Cavallero, S. et al. CXCL12 signaling is essential for maturation of the ventricular coronary endothelial
plexus and establishment of functional coronary circulation. _Dev. Cell_ 33, 469–477 (2015). Article CAS PubMed PubMed Central Google Scholar * Schneider, C. et al. Primate heart
regeneration via migration and fibroblast repulsion by human heart progenitors. Preprint at https://www.biorxiv.org/content/10.1101/2020.07.03.183798v1.full (2020). * He, W. et al.
Exogenously administered secreted frizzled related protein 2 (Sfrp2) reduces fibrosis and improves cardiac function in a rat model of myocardial infarction. _Proc. Natl Acad. Sci. USA_ 107,
21110–21115 (2010). Article CAS PubMed PubMed Central Google Scholar * Carstensen-Kirberg, M. et al. Inverse associations between serum levels of secreted frizzled-related protein-5
(SFRP5) and multiple cardiometabolic risk factors: KORA F4 study. _Cardiovasc. Diabetol._ 16, 109 (2017). Article PubMed PubMed Central Google Scholar * Du, Y. et al. High serum secreted
frizzled-related protein 5 levels associates with early improvement of cardiac function following ST-segment elevation myocardial infarction treated by primary percutaneous coronary
intervention. _J. Atheroscler. Thromb._ 26, 868–878 (2019). Article CAS PubMed PubMed Central Google Scholar * Liu, S. et al. Yap promotes noncanonical Wnt signals from cardiomyocytes
for heart regeneration. _Circ Res._ 129, 782–797 (2021). Article CAS PubMed PubMed Central Google Scholar * Lincoln, J., Florer, J. B., Deutsch, G. H., Wenstrup, R. J. & Yutzey, K.
E. ColVa1 and ColXIa1 are required for myocardial morphogenesis and heart valve development. _Dev. Dyn._ 235, 3295–3305 (2006). Article CAS PubMed Google Scholar * Ganesan, K. et al.
Inhibition of gastric cancer invasion and metastasis by _PLA2G2A_, a novel β-catenin/TCF target gene. _Cancer Res._ 68, 4277–4286 (2008). Article CAS PubMed Google Scholar * He, B. et
al. Vascular targeting of LIGHT normalizes blood vessels in primary brain cancer and induces intratumoural high endothelial venules. _J. Pathol._ 245, 209–221 (2018). Article CAS PubMed
PubMed Central Google Scholar * Menon, R. et al. Single-cell analysis of progenitor cell dynamics and lineage specification in the human fetal kidney. _Development_ 145, dev164038 (2018).
Article PubMed PubMed Central Google Scholar * Wolock, S. L., Lopez, R. & Klein, A. M. Scrublet: computational identification of cell doublets in single-cell transcriptomic data.
_Cell Syst._ 8, 281–291 (2019). Article CAS PubMed PubMed Central Google Scholar * Stuart, T. et al. Comprehensive integration of single-cell data. _Cell_ 177, 1888–1902 (2019). Article
CAS PubMed PubMed Central Google Scholar * Efremova, M., Vento-Tormo, M., Teichmann, S. A. & Vento-Tormo, R. CellPhoneDB: inferring cell–cell communication from combined expression
of multi-subunit ligand–receptor complexes. _Nat. Protoc._ 15, 1484–1506 (2020). Article CAS PubMed Google Scholar * Qiu, P. Embracing the dropouts in single-cell RNA-seq analysis.
_Nat. Commun._ 11, 1169 (2020). Article CAS PubMed PubMed Central Google Scholar * Raudvere, U. et al. g:Profiler: a web server for functional enrichment analysis and conversions of
gene lists (2019 update). _Nucleic Acids Res._ 47, W191–W198 (2019). Article CAS PubMed PubMed Central Google Scholar * Kleshchevnikov, V. et al. Cell2location maps fine-grained cell
types in spatial transcriptomics. _Nat. Biotechnol._ 40, 661–671 (2022). Article CAS PubMed Google Scholar Download references ACKNOWLEDGEMENTS The authors would like to thank R. Barker
at the University of Cambridge for assistance in obtaining the fetal tissue samples used in all analyses. Additionally, we are grateful for support from the Wellcome Sanger Cellular
Generation and Phenotyping team and the Core DNA Pipelines team. This research was funded by the British Heart Foundation (BHF) Senior Fellowship (FS/18/46/33663)(S.S. and L.G.); the
Oxbridge BHF Centre for Regenerative Medicine (RM/17/2/33380) (V.K.S.); and BHF grants PG/17/24/32886 (L.G.) and RG/17/5/32936 (H.D.). We also acknowledge core support from the Wellcome
Trust, the Medical Research Council and the Wellcome Trust–Medical Research Council Cambridge Stem Cell Institute. This research was funded, in whole or in part, by the Wellcome Trust (grant
no. 203151/Z/16/Z). Finally, this project has been made possible, in part, by the Wellcome Trust (WT206194, S.A.T), the Wellcome Trust Clinical PhD Fellowship (J.C) and the Overseas
Research Fellowship of the Takeda Science Foundation (K.K). For the purpose of open access, the author has applied a CC BY public copyright licence to any Author Accepted Manuscript version
arising from this submission. AUTHOR INFORMATION Author notes * These authors jointly supervised this work: Laure Gambardella, Sanjay Sinha. AUTHORS AND AFFILIATIONS * Wellcome-MRC Cambridge
Stem Cell Institute, Jeffrey Cheah Biomedical Centre, Cambridge Biomedical Campus, University of Cambridge, Cambridge, UK Vincent R. Knight-Schrijver, Hongorzul Davaapil, Semih Bayraktar,
Alexander D. B. Ross, Ludovic Vallier, Laure Gambardella & Sanjay Sinha * Department of Paediatrics, University of Cambridge, Cambridge, UK Alexander D. B. Ross * Department of Medical
Genetics, University of Cambridge, Cambridge, UK Alexander D. B. Ross * Wellcome Sanger Institute, Wellcome Genome Campus, Cambridge, UK Kazumasa Kanemaru, James Cranley, Monika Dabrowska,
Minal Patel, Krzysztof Polanski, Sarah Teichmann & Laure Gambardella * John van Geest Centre for Brain Repair, Cambridge University, Cambridge, UK Xiaoling He * Berlin Institute of
Health (BIH), BIH Centre for Regenerative Therapies (BCRT), Charité - Universitätsmedizin, Berlin, Germany Ludovic Vallier * Max Planck Institute for Molecular Genetics, Berlin, Germany
Ludovic Vallier * Cavendish Laboratory, Department of Physics, University of Cambridge, Cambridge, UK Sarah Teichmann Authors * Vincent R. Knight-Schrijver View author publications You can
also search for this author inPubMed Google Scholar * Hongorzul Davaapil View author publications You can also search for this author inPubMed Google Scholar * Semih Bayraktar View author
publications You can also search for this author inPubMed Google Scholar * Alexander D. B. Ross View author publications You can also search for this author inPubMed Google Scholar *
Kazumasa Kanemaru View author publications You can also search for this author inPubMed Google Scholar * James Cranley View author publications You can also search for this author inPubMed
Google Scholar * Monika Dabrowska View author publications You can also search for this author inPubMed Google Scholar * Minal Patel View author publications You can also search for this
author inPubMed Google Scholar * Krzysztof Polanski View author publications You can also search for this author inPubMed Google Scholar * Xiaoling He View author publications You can also
search for this author inPubMed Google Scholar * Ludovic Vallier View author publications You can also search for this author inPubMed Google Scholar * Sarah Teichmann View author
publications You can also search for this author inPubMed Google Scholar * Laure Gambardella View author publications You can also search for this author inPubMed Google Scholar * Sanjay
Sinha View author publications You can also search for this author inPubMed Google Scholar CONTRIBUTIONS V.K.S. conceived, designed and performed the analysis and wrote the paper. H.D.
coordinated and prepared fetal samples for sequencing and sectioning. S.B. carried out the immunocytochemistry. A.R. and L.V. contributed data sample F5. K.K. and J.C. carried out the
spatial transcriptomics and Cell2Location analysis. M.D., M.P. and K.P. carried out and optimized the spatial transcriptomics protocols. X.H. coordinated and collected all fetal tissue
samples. S.T. contributed spatial transcriptomics data. L.G. produced the hESC-EPI differentiation samples and dataset. L.G. and S.S. conceived and designed the analysis and wrote the paper.
All authors reviewed and edited the manuscript. CORRESPONDING AUTHORS Correspondence to Laure Gambardella or Sanjay Sinha. ETHICS DECLARATIONS COMPETING INTERESTS In the past 3 years,
S.A.T. has consulted or been a member of scientific advisory boards at Roche, Genentech, Biogen, GlaxoSmithKline, Qiagen and ForeSite Labs and is an equity holder of Transition Bio. All
other authors declare no competing interests. PEER REVIEW PEER REVIEW INFORMATION _Nature Cardiovascular Research_ thanks the anonymous reviewers for their contribution to the peer review of
this work. ADDITIONAL INFORMATION PUBLISHER’S NOTE Springer Nature remains neutral with regard to jurisdictional claims in published maps and institutional affiliations. EXTENDED DATA
EXTENDED DATA FIG. 1 STRATIFIED SAMPLING, INTEGRATION, AND QUALITY OF ADULT AND FETAL SCRNA-SEQ DATASETS. Processing of adult and fetal datasets and integration with A, subsampling
stratifications and step-wise subsampling strategy for adult cells and nuclei; B, naive integration of fetal data and uniform manifold approximation and projection (UMAP) showing I,
clustering; II, predicted doublets; III, erythrocyte detection using a two-compartment Gaussian mixture model on the summed expression of erythrocyte genes _HBB, HBG1, HBG2, HBM, HBA2, HBA1,
HBQ1, ALAS2_ allowing to identify; IV, high erythrocyte fraction clusters for removal; validated by V, the summed expression of erythrocyte genes. C, Fetal samples contained a greater
quantity of I, UMIs and; II, unique genes expressed and were downsampled to 15 000 UMIs and; III, the resulting relationship between depth and complexity was similar in both adult and fetal
samples. Fetal datasets were integrated after erythrocyte removal as shown and clustered ready for subsampling and integration as shown in D, I-V, Louvain clustering using resolutions of
0.1–2 respectively. Resolution 0.5 was selected with 21 clusters, which when subsampled down to the number of epicardial cells identified using epicardial markers such as VI, UPK3B (n =
1598), produced approximately the same number of fetal cells as subsampled adult cells. Integration of subsampled adult and fetal data; E, was performed hierarchically by prioritising donors
in a custom integration tree. Distributions for each box in 1c(i-ii) were drawn from n = 1562, 3504, 5074, 1479, 3454, 1866, 2845, 2124, 3400, 959, 3763, 4226, 2072, 1709, 1941, 1919, 8023,
724, 3630, 1832, 576, 8597, 5460, and 6764 cells respectively in the order of plotted groups. Each distribution’s centre horizontal line denotes population median, while box edges and
whiskers are drawn at 1 and 1.5 × interquartile range respectively. EXTENDED DATA FIG. 2 ANNOTATION AND CLUSTERING OF INTEGRATED HEART SAMPLES WAS CARRIED OUT OVER SEVERAL RESOLUTIONS.
Integrated adult and fetal data were iteratively clustered by aggregating high-resolution sub-clusters as shown in A, uniform manifold approximation and projection (UMAP) embeddings showing
I, 10 initial low resolution clustering followed by; II, sub-clustering, and III-IV, aggregation of biologically similar cell clusters over two intermediate resolutions. Sub-clustering of
low-resolution clusters depicted with; B sub-cluster UMAPs illustrating the identification of cell types and states using previous adult annotations, cell sources, and unsupervised
clustering. EXTENDED DATA FIG. 3 FURTHER OBSERVATIONS AND TECHNICAL HURDLES IN GENERATING EPICARDIAL GENE MODULES. The gene expression matrix of epicardial cells was isolated from the rest
and binarised. Co-occurrence clustering of genes including nuclei in the matrix created two gene modules and a, principal component analysis (PCA) on cellular commitment to modules confirms
that the main separation of the dataset was between cells and nuclei. Gene Ontology enrichment was carried out; b, revealing broad terms associated with nuclear or cytosolic compartments.
Use of Kendall’s rank correlation; c, between cell variables and PCA components with components 2 and 4 highly correlating with age and clusters resepctively as variables of interest for
visualisation. d, the mean expression of the 6 gene modules in the 19 resolution-2 clusters as well as cells grouped by source and; e, the expression of the top 20 genes in each gene module
across age-ordered epicardial cells (age component PC2). EXTENDED DATA FIG. 4 EPICARDIAL-SPECIFIC GENES INCLUDED MANY COLLAGENS FORMING A LARGE COMPONENT OF EPICARDIAL-SPECIFIC
COMMUNICATION. Predictive ligand receptor analysis with CellPhoneDB of communication shown in a, from epicardial cells with other heart cell types with a large component of fetal-specific
and epicardial selective collagens interacting both with fetal and with adult hearts. Significance of each interaction was calculated in CellPhoneDB using the fraction of mean expression
between gene pairs that are equivalent or higher than the gene-pair expression in 1000 random permutations. Recall and precision statistics for the positive expression of each epicardial
marker was scored b.i – b.iv, on their abilities to predict non-epicardial cells, fetal, adult, or all epicardial cells, which was repeated; b.v & b.vi, in only UPK3B positive cells in
predicting either EPDCs or Mesothelial epicardial cells. EXTENDED DATA FIG. 5 TRAINING AND VALIDATION OF A RANDOM FOREST ON HIGH-RESOLUTION CELL TYPES. A training dataset cells for random
forest classification was sampled as shown by a, the uniform manifold approximation and projection (UMAP) of integrated data. Sampled cells were shown in black covering 33 % of the dataset
and with 6 folds representing the dataset evenly as shown by the coloured sub-plots. Cross-validation (6-folds) suggests reasonable model accuracy for most clusters by comparing b, precision
and recall (mean and standard deviation, k = 6). Prediction of the remaining naive 66 % of cells as test data shown in c, the confusion matrix between the reference and predicted cell
classes with high scores for most cell types. EXTENDED DATA FIG. 6 Predicted separation between EPDCs and Mesothelial epicardium with UPK3B, TM4SF1, and POSTN using a, pseudostain on
integrated uniform manifold approximation and projection embeddings followed by immunocytochemical staining using antibodies. The UPK3B antibody did not label the epicardium clearly as a
result of undefined technical difficulties. Lower-magnification imaging of b, immunofluorescence using antibodies for KRT19 and POSTN as one strategy for distinguishing between EPDCs and
Mesothelial epicardium in fetal cardiac tissue. Images shown are representative of stains carried out on three sections with each combination on a single heart (a, BRC2281; b, BRC2375).
EXTENDED DATA FIG. 7 Spatial transcriptomics of a fetal heart with a gestational age of 9 weeks and 4 days. a, H&E staining and spot annotation, bi-iv, epicardial markers; and ci-vi,
expression scores of epicardial gene modules as calculated from the top 20 genes in each module. This experiment was carried out once. SUPPLEMENTARY INFORMATION REPORTING SUMMARY
SUPPLEMENTARY TABLE 1 SAMPLE BASIC STATISTICS. Sample information, including sample labels, age, region, donor, number of cells called by Cell Ranger and number of cells retained after
quality control steps. Ages of fetal samples are approximate as post-conception days. SUPPLEMENTARY TABLE 2 RAW OUTPUT FROM ALL CLUSTER MARKERS. Differential expression analysis results for
all clusters compared with the whole dataset identifying upregulated genes using two-sided Wilcoxon rank-sum tests on the age-integrated dataset (log2 fold change > 0.5, _P_ < 1 ×
10−10). _P_ values were adjusted for multiple comparisons using Bonferroni correction. The table contains all clusters, and the results are unfiltered. Genes are ranked according to their
_P_ value. SUPPLEMENTARY TABLE 3 MESOTHELIAL MARKERS FROM RESOLUTION 2 CLUSTERING. The top 100 differentially upregulated genes of mesothelial epicardial cluster 9 compared with all other
clusters as identified using two-sided Wilcoxon rank-sum tests on the age-integrated dataset (log2 fold change > 0.5, _P_ < 1 × 10−10). _P_ values were adjusted for multiple
comparisons using Bonferroni correction. Genes are ranked according to their log2 fold change against the overall dataset. Epicardial genes upregulated in only mesothelial cluster 9 are
shown in blue. SUPPLEMENTARY TABLE 4 EPDC MARKERS FROM RESOLUTION 2 CLUSTERING. The top 100 differentially upregulated genes of fibroblast-like epicardial cluster 8 compared with all other
clusters as identified using two-sided Wilcoxon rank-sum tests on the age-integrated dataset (log2 fold change > 0.5, _P_ < 1 × 10−10). Genes are ranked according to their log2 fold
change against the overall dataset. Epicardial genes upregulated in only fibroblast-like cluster 8 are shown in red. SUPPLEMENTARY TABLE 5 GENES IN EPICARDIAL GENE MODULES AND THEIR
EXPRESSION IN EPICARDIAL STATES. Gene module genes ordered by the mean of their chi-squared scores for all other genes in their module alongside the mean expression of each gene in the
epicardial subclusters seen in Fig. 3b. Spreadsheet file with multiple sheets, one for each gene module. SUPPLEMENTARY TABLE 6 GENE ONTOLOGY ENRICHMENT ANALYSIS RESULTS FOR EACH MODULE.
Spreadsheet file with multiple sheets, one for each gene module. All associated terms are included irrespective of significance. Gene set significance was calculated with gProfiler using
hypergeometric tests against a background of genes expressed in this study (_n_ = 27,956) and adjusted for multiple comparisons using gprofiler’s g:SCS algorithm. SUPPLEMENTARY TABLE 7
STAGE-SELECTIVE MARKERS FOR EPICARDIUM. Epicardial-specific markers were split into fetal, adult or the shared intersect between stages as identified using two-sided Wilcoxon rank-sum tests
(log2 fold change > 0.5, _P_ < 1 × 10−10). Also includes a control set of genes identified as an overall adult versus fetal effect using using two-sided Wilcoxon rank-sum tests
comparing of all adult cells compared with all fetal cells (log2 fold change > 0.5, _P_ < 1 × 10−10). _P_ values were adjusted for multiple comparisons using Bonferroni correction.
Genes in this control set were removed from results owing to epicardial non-specificity. Spreadsheet file with multiple sheets, one for each of fetal, shared, adult or control genes.
SUPPLEMENTARY TABLE 8 LIBRARY OF EPICARDIAL MARKERS. Epicardial-specific markers were scored by precision, recall and _F_-score when predicting epicardial cells in adult, fetal or all
epicardial cells irrespective of stage as well as non-epicardial cells. This supplementary spreadsheet also includes a library of markers used to separate between EPDCs and mesothelial
epicardium under the assumption of epicardial positivity for _UPK3B_. Data are associated with Extended Fig. 5. SUPPLEMENTARY TABLE 9 GENES DIFFERENTIALLY EXPRESSED IN ADULT EPICARDIUM
COMPARED WITH FETAL EPICARDIUM. Used as input into gene–gene co-occurrence analysis for gene module construction, this is a list of genes resulting from a Wilcoxon rank-sum test comparing
epicardium of both stages (log2 fold change > 0.5, _P_ < 1 × 10−10). SOURCE DATA SOURCE DATA FIG. 4 CellPhoneDB statistical analysis results for predicted epicardial-mediated
interactions with the other heart cells of both adult and fetal hearts. Corresponds to results plotted in Fig. 4d,e. RIGHTS AND PERMISSIONS OPEN ACCESS This article is licensed under a
Creative Commons Attribution 4.0 International License, which permits use, sharing, adaptation, distribution and reproduction in any medium or format, as long as you give appropriate credit
to the original author(s) and the source, provide a link to the Creative Commons license, and indicate if changes were made. The images or other third party material in this article are
included in the article’s Creative Commons license, unless indicated otherwise in a credit line to the material. If material is not included in the article’s Creative Commons license and
your intended use is not permitted by statutory regulation or exceeds the permitted use, you will need to obtain permission directly from the copyright holder. To view a copy of this
license, visit http://creativecommons.org/licenses/by/4.0/. Reprints and permissions ABOUT THIS ARTICLE CITE THIS ARTICLE Knight-Schrijver, V.R., Davaapil, H., Bayraktar, S. _et al._ A
single-cell comparison of adult and fetal human epicardium defines the age-associated changes in epicardial activity. _Nat Cardiovasc Res_ 1, 1215–1229 (2022).
https://doi.org/10.1038/s44161-022-00183-w Download citation * Received: 14 October 2021 * Accepted: 03 November 2022 * Published: 21 December 2022 * Issue Date: December 2022 * DOI:
https://doi.org/10.1038/s44161-022-00183-w SHARE THIS ARTICLE Anyone you share the following link with will be able to read this content: Get shareable link Sorry, a shareable link is not
currently available for this article. Copy to clipboard Provided by the Springer Nature SharedIt content-sharing initiative