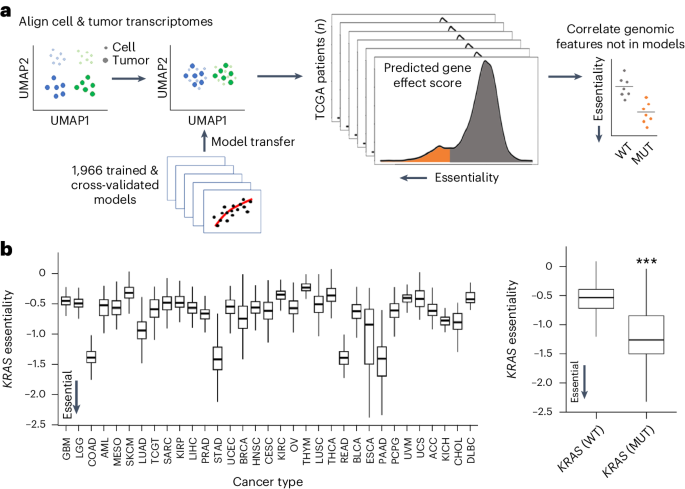
- Select a language for the TTS:
- UK English Female
- UK English Male
- US English Female
- US English Male
- Australian Female
- Australian Male
- Language selected: (auto detect) - EN
Play all audios:
Cancer dependency maps have accelerated the discovery of essential genes and potential drug targets. Here we used machine learning to build translational dependency maps of patients’ tumors
and normal tissue biopsies, which identified oncogenes and synthetic lethalities that are predictive of drug responses and patients’ outcomes. Access through your institution Buy or
subscribe This is a preview of subscription content, access via your institution ACCESS OPTIONS Access through your institution Access Nature and 54 other Nature Portfolio journals Get
Nature+, our best-value online-access subscription $29.99 / 30 days cancel any time Learn more Subscribe to this journal Receive 12 digital issues and online access to articles $119.00 per
year only $9.92 per issue Learn more Buy this article * Purchase on SpringerLink * Instant access to full article PDF Buy now Prices may be subject to local taxes which are calculated during
checkout ADDITIONAL ACCESS OPTIONS: * Log in * Learn about institutional subscriptions * Read our FAQs * Contact customer support REFERENCES * Gao, J. et al. Integrative analysis of complex
cancer genomics and clinical profiles using the cBioPortal. _Sci. Signal._ 6, pl1 (2013). THIS PAPER PROVIDES AN OVERVIEW AND PRACTICAL GUIDE TO CLINICAL CANCER GENOMICS DATASETS AVAILABLE
THROUGH THE CBIOPORTAL. Article PubMed PubMed Central Google Scholar * Ostroverkhova, D., Przytycka, T. M. & Panchenko, A. R. Cancer driver mutations: predictions and reality.
_Trends Mol. Med._ 7, 554–566 (2023). THIS REVIEW ARTICLE DESCRIBES THE STATE OF THE ART AND CURRENT LIMITATIONS OF IDENTIFYING AND CHARACTERIZING DRIVER MUTATIONS IN PATIENT TUMORS. Article
Google Scholar * Boehm, J. S. et al. Cancer research needs a better map. _Nature_ 589, 524–526 (2021). THIS COMMENTARY DESCRIBES THE CELL-BASED CANCER DEPENDENCY MAP (DEPMAP). Article
Google Scholar * Han, K. et al. CRISPR screens in cancer spheroids identify 3D growth-specific vulnerabilities. _Nature_ 580, 136–141 (2020). THIS STUDY DEMONSTRATES THAT SOME CANCER
VULNERABILITIES ARE MORE PROMINENT IN THREE-DIMENSIONAL SPHEROIDS THAN IN TWO-DIMENSIONAL MONOLAYERS. Article CAS PubMed PubMed Central Google Scholar * Martinez-Ruiz, C. et al.
Genomic-transcriptomic evolution in lung cancer and metastasis. _Nature_ 616, 543–552 (2023). THIS LANDMARK WORK BY THE TRACERX CONSORTIUM DEEPLY CHARACTERIZED THE GENOMIC AND TRANSCRIPTOMIC
EVOLUTION OF HUMAN TUMORS, HIGHLIGHTING THE COMPLEXITY OF INTRATUMOR HETEROGENEITY THAT SHOULD BE ACCOUNTED FOR IN FUTURE TRANSLATIONAL DEPENDENCY MAPS. Article CAS PubMed PubMed Central
Google Scholar Download references ADDITIONAL INFORMATION PUBLISHER’S NOTE Springer Nature remains neutral with regard to jurisdictional claims in published maps and institutional
affiliations. THIS IS A SUMMARY OF: Shi, X. et al. Building a translational cancer dependency map for The Cancer Genome Atlas. _Nat. Cancer_ https://doi.org/10.1038/s43018-024-00789-y
(2024). RIGHTS AND PERMISSIONS Reprints and permissions ABOUT THIS ARTICLE CITE THIS ARTICLE Using machine learning to translate tumor dependencies. _Nat Cancer_ 5, 1141–1142 (2024).
https://doi.org/10.1038/s43018-024-00790-5 Download citation * Published: 23 July 2024 * Issue Date: August 2024 * DOI: https://doi.org/10.1038/s43018-024-00790-5 SHARE THIS ARTICLE Anyone
you share the following link with will be able to read this content: Get shareable link Sorry, a shareable link is not currently available for this article. Copy to clipboard Provided by the
Springer Nature SharedIt content-sharing initiative