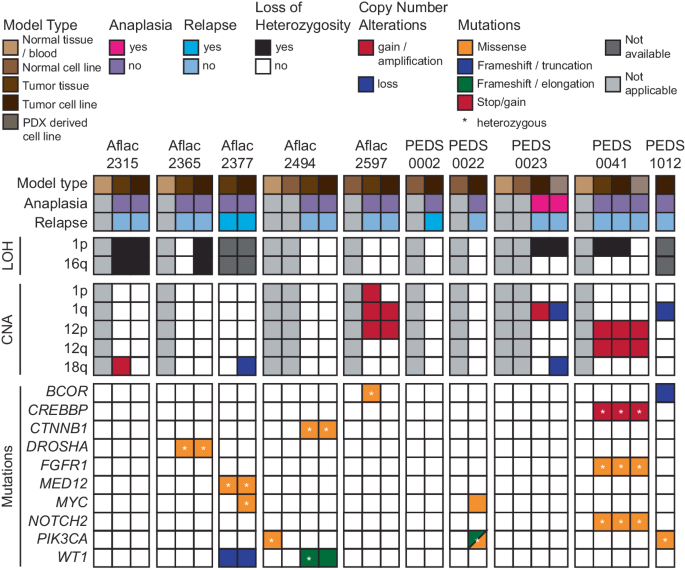
- Select a language for the TTS:
- UK English Female
- UK English Male
- US English Female
- US English Male
- Australian Female
- Australian Male
- Language selected: (auto detect) - EN
Play all audios:
ABSTRACT Wilms tumor (WT) is the most common renal malignancy of childhood. Despite improvements in the overall survival, relapse occurs in ~15% of patients with favorable histology WT
(FHWT). Half of these patients will succumb to their disease. Identifying novel targeted therapies remains challenging in part due to the lack of faithful preclinical in vitro models. Here
we establish twelve patient-derived WT cell lines and demonstrate that these models faithfully recapitulate WT biology using genomic and transcriptomic techniques. We then perform
loss-of-function screens to identify the nuclear export gene, _XPO1_, as a vulnerability. We find that the FDA approved XPO1 inhibitor, KPT-330, suppresses _TRIP13_ expression, which is
required for survival. We further identify synergy between KPT-330 and doxorubicin, a chemotherapy used in high-risk FHWT. Taken together, we identify XPO1 inhibition with KPT-330 as a
potential therapeutic option to treat FHWTs and in combination with doxorubicin, leads to durable remissions in vivo. SIMILAR CONTENT BEING VIEWED BY OTHERS SITRAVATINIB IN COMBINATION WITH
NIVOLUMAB PLUS IPILIMUMAB IN PATIENTS WITH ADVANCED CLEAR CELL RENAL CELL CARCINOMA: A PHASE 1 TRIAL Article Open access 10 January 2025 PTEN LOSS CONFERS SENSITIVITY TO RAPALOGS IN CLEAR
CELL RENAL CELL CARCINOMA Article 14 February 2022 SELECTIVE INHIBITION OF NUCLEAR EXPORT: A PROMISING APPROACH IN THE SHIFTING TREATMENT PARADIGMS FOR HEMATOLOGICAL NEOPLASMS Article Open
access 29 January 2022 INTRODUCTION Wilms tumor (WT) is the most common childhood renal tumor and represents ~6% of all pediatric cancers with a peak age of presentation at 3 years1,2,3. In
the United States, African American children have 2.5 times higher rates of WT when compared to Caucasian American or Asian American children4,5. Approximately 95% of WT patients present
with favorable histology (FHWT), whereas the other 5% present with diffuse anaplastic histology (DAWT) which is usually associated with _TP53_ mutations or deletions. Current therapy for low
risk FHWT patients (Stage I and II) includes the use of surgery with or without chemotherapy (e.g., vincristine, dactinomycin). For high-risk FHWT disease (e.g., Stage III and IV
individuals with pulmonary metastasis or invasion of the renal sinus and capsule), doxorubicin and radiation therapy are added to low-risk disease therapy. Despite increases in response and
survival over the past 50 years, ~15% of patients with WT recur6,7,8 and salvage regimens which include doxorubicin for low risk patients are successful only in 50% of patients and carry
significant morbidity. A major limiting factor in testing novel targeted therapies in WT is the lack of faithful in vitro preclinical models. Prior cell line models of WT have been
recharacterized as other pediatric cancers such as rhabdoid tumor (e.g., G401) and Ewing sarcoma (e.g., SK-NEP)9,10,11,12. Recent efforts to generate WT cell lines have been limited due to
finite passaging13,14,15,16. Despite this limitation, a repository of WT organoids and patient-derived xenografts (PDXs) has been developed in recent years17,18,19. Nomination of rational
therapeutics for organoid or in vivo PDX studies, however, requires systematic in vitro efforts using faithful cancer cell lines. Here, we have developed faithful cell line models using
genome and transcriptome sequencing of WT which recapitulate known WT biology. We then performed functional genomic screens focused on druggable targets to nominate WT therapeutics20,21,22.
RESULTS WT CELL LINES FAITHFULLY RECAPITULATE GENOMIC AND TRANSCRIPTOMIC FEATURES OF WT Recent studies have shown the feasibility of generating short-term WT cell lines with limited genomic
testing13,14,15,16. We developed 12 short-term WT cell lines from 10 patients (10 patient-derived cell lines and 2 PDX-derived cell lines following one passage of the PDX in mice (Annotated
as T2); Fig. 1; “Methods”). Ten patient samples were obtained at time of diagnosis and two were obtained at time of recurrence (Aflac_2377T and CCLF_PEDS_0002T hereafter PEDS_0002T). Nine
patients had favorable histology Wilms tumor (FHWT) and one patient had diffuse anaplastic Wilms Tumor (DAWT; CCLF_PEDS_0023T hereafter PEDS_0023T). We then performed ultra-low coverage
whole genome sequencing (WGS) to infer copy number status, whole exome sequencing (WES) to identify known mutations in WT, and RNA-sequencing to assess the transcriptome in the patients’
tumor and the matched cell lines. We identified 1q gain, a poor prognostic factor in Wilms tumor biology, in two patients (Aflac_2597 and PEDS_0023) and we identified combined
loss-of-heterozygosity (LOH) in 1p and 16q in two patients (Aflac_2315 and Aflac_2365)23,24. We further observed gain in chromosome 12, which has been associated with relapse, in two
patients (Aflac_2597 and CCLF_PEDS_0041 hereafter PEDS_0041). Methylation status was not assessed in this study. We then assessed the mutational profiles of these tumors and cell lines and
observed genetic similarity between patient tumor samples and matched cell lines (Fig. 1, Supplementary Tables 1 and 2 and Supplementary Data 1). We found that the observed mutations
reflected the spectrum of mutations seen in the WT samples profiled in the National Cancer Institute (NCI) Therapeutically Applicable Research to Generate Effective Treatments (TARGET)25 as
well as other studies18,26 (Fig. 1). We identified genetic heterogeneity between the tumor and the tumor derived cell line in 5 of the 10 patient samples (Aflac_2315, Aflac_2377, Aflac_2597,
PEDS_0023, and PEDS_0041), supporting the previously observed genetic heterogeneity in Wilms tumor samples18,27. Lastly, we identified 2 of the FHWT patient-derived tumor cell lines had no
mutations typically observed in Wilms tumor, an observation also seen in 5% of patient samples in prior genomic analyses of FHWT25. To further confirm that our cell lines were consistent
with Wilms tumor, we performed RNA-sequencing of these samples and compared it to the TARGET25 and St. Jude Children’s Research Hospital’s WT datasets18 (“Methods”) using uniform manifold
approximation and projection (UMAP)28. We observed that most of our WT and normal cell lines clustered closely with the WT tumor and normal samples, respectively (Fig. 2a). However, one FHWT
cell line (Aflac_2315) did not clearly cluster with our tumor tissue and cell lines. _SIX2_ is elevated in Wilms Tumor and the mean log2 counts for our normal tissue and cell lines were
2.54 (standard deviation of 0.32) whereas in our FHWT was 3.73 (standard deviation of 0.20). Aflac_2315 had _SIX2_ log2 counts of 3.86 in the tumor and 3.39 in the cell line. More broadly,
we observed that _SIX2_ and _CITED1_ were generally upregulated across our WT cell lines, further consistent with WT biology (Fig. 2b)29,30,31. Interestingly, a gene which has a known
therapeutic target, _XPO1_, was modestly upregulated across renal tumors (Fig. 2b). Although the tumor cell lines exhibited similar expression of _XPO1_ as compared to tumor tissue, we also
found that the normal cell lines included in this study also had upregulation of _XPO1_. Collectively, our findings suggest that our patient-derived cell lines recapitulate known biology of
WT and serve as faithful representations of WT. RNAI AND CRISPR-CAS9 SCREENS IDENTIFY XPO1 AS A POTENTIAL THERAPEUTIC TARGET We then asked if we could identify genetic vulnerabilities in WT
despite the short-term lifespan of these cell lines. Following rapid expansion within the first five passages, we subjected cell lines derived from three unique patient samples (PEDS_0002,
PEDS_0023, and PEDS_0041) to targeted loss of function RNA interference and CRISPR-Cas9 screens (“Methods” and Supplementary Data 2). Given the limited number of cells that could be
expanded, we used the Druggable Cancer Targets (DCT) library consisting of 429 genes that focused on known or upcoming therapeutic targets that had small molecule inhibitors22,32 (Fig. 3a).
For the RNAi screens, we introduced the DCT lentiviral library into PEDS_0002T, PEDS_0041_T1, and PEDS_0041_T2 cells and then used Model-based Analysis of Genome-wide CRISPR-Cas9 Knockout
(MAGeCK) to identify 20 genes which were required for survival across these patient-derived cell lines33 (Fig. 3b and Supplementary Fig. 1a–d). We subsequently performed CRISPR-Cas9 screens
in PEDS_0002T, PEDS_0023_T1 and PEDS_0023_T2 to identify 24 genes that when deleted led to decreased viability across these cell lines (Fig. 3b and Supplementary Fig. 1e–k). From these
orthogonal screens, we identified seven genes which overlapped between RNAi and CRISPR-Cas9 screens. These included genes involved in nuclear export (_KPNB1_ and _XPO1_), regulators of the
cell cycle (_KIF11_ and _POLA1_), DNA damage (_UBA1_ and _DDB1_), and cell survival (_BIRC5_) (Fig. 3b). We focused on the role of the nuclear export inhibitor KPT-330 (selinexor) due to its
recent FDA-approval in multiple myeloma and diffuse large B-cell lymphoma34. Further, KPT-330 has recently been identified as a potential effective therapy which targets renal tumors with
aberrant XPO1 activation35. We first assessed the expression levels of _XPO1_ across 85 cancer types through the University of California Santa Cruz Treehouse Childhood Cancer Initiative36.
From 12,719 patient samples, we found 85.4% of Wilms Tumor samples were in the top 20% of samples with high _XPO1_ levels (Fig. 3c). To validate the requirement of XPO1 in WT cell lines, we
assessed cell viability of WT cells using KPT-330 and _XPO1_ suppression with RNAi (Fig. 3d, e and Supplementary Figs. 2a–c and 3). Specifically, we determined the IC50 values for our WT
cell lines and compared these to normal kidney cell lines. Among tumor cell lines we observed an average IC50 of 1.79 µM ± 1.8, with over half of these cell lines showing an IC50 at
nanomolar concentrations (e.g., 25–800 nM). Among normal cell lines we observed a 6-fold increase in the average IC50 of 11.64 µM ± 6.6 with a _P_ value of 0.0002 (Fig. 3d). Moreover, we
observed increased sensitivity to KPT-330 in all tumor cell lines for the three matched tumor-normal pairs (PEDS_0023, Aflac_2494 and Aflac_2597). The increased sensitivity of tumor cells as
compared to normal cells suggests KPT-330 may be a selective inhibitor with limited off-target toxicity. We observed that _XPO1_ expression was not correlated with KPT-330 sensitivity (_r_2
= 0.004137, Supplementary Fig. 4a) suggesting that on target activity is not entirely dependent on elevated _XPO1_ transcript levels. However, we found that the relative fold change in
_XPO1_ levels was significantly higher in tumor cell lines as compared to normal cell lines (_P_ value 0.044; Supplementary Fig. 4b). These findings are consistent with prior findings that
suggest on target activity of KPT-330 decreases the abundance of XPO1 protein (Fig. 3e and Supplementary Fig. 4c, d), inducing a positive feedback loop which increases XPO1 mRNA levels
(Supplementary Fig. 4b)37,38,39,40,41. However, the downstream mechanisms of action vary by cancer type. Taken together, these findings suggest that XPO1 is a potential selective therapeutic
target in WT. XPO1 INHIBITION INDUCES CELL DEATH THROUGH THE TRIP13/P53 AXIS We next investigated a potential mechanism of action for the nuclear export inhibitor KPT-330 in WT. We
performed fluorescence-activated cell sorting analyses to assess the changes in the cell cycle following KPT-330 treatment. We found that changes in G1 were not consistent across our FHWT
cell lines (Fig. 4a and Supplementary Table 3). However, we saw decreases of S phase and significant increases in G2/M suggesting that KPT-330 in our FHWT cell lines led primarily to a G2/M
arrest (Fig. 4a). We focused our efforts on understanding the mechanisms in FHWT. To understand the transcriptional changes driving this G2/M arrest, we treated p53 wild-type PEDS_0041_T1
with DMSO or KPT-330 using the IC50 concentrations (e.g., 6 µM) for 24 hours and performed RNA-sequencing (“Methods”). We then performed differential expression analyses and found 1120 genes
differentially expressed (Fig. 4b and Supplementary Data 3). We examined gene sets enriched or suppressed upon KPT-330 treatment using Gene Set Enrichment Analyses (GSEA)42 and observed 32
hallmark gene sets significantly enriched or suppressed (Supplementary Data 4). We found gene sets suppressed affecting the progression of G1 (e.g., E2F) and G2/M (Fig. 4c) along with
activation of the p53 pathway (Supplementary Data 4). We then confirmed the activation of p53 by observing accumulation of p53 following treatment with KPT-330 by 48 hours (Fig. 3e and
Supplementary Figs. 4c–e and 5a). KPT-330 is known to affect tumor suppressor genes and transcription factors such as p53 through nuclear accumulation of p53 and prevention of MDM2/4 related
degradation43. Since KPT-330 has been associated with upregulating p53 activity, we sought to determine if p53 was indeed important in mediating WT cell death in the context of nuclear
export inhibition44. We depleted _TP53_ using CRISPR-Cas9 in CCLF_PEDS1012T (hereafter PEDS1012T) and CCLF_PEDS_0022T (hereafter PEDS_0022T) as compared to a control gRNA to _LacZ_
(Supplementary Fig. 5b). We then assessed the IC50 of KPT-330 and found a 12- to 20-fold increase in these values when _TP53_ was deleted (Fig. 4d and Supplementary Fig. 5c). This
significant change in IC50s suggests a critical role of _TP53_ in KPT-330 induced cell death in FHWT. We subsequently assessed the 1120 genes differentially expressed following KPT-330
treatment (Fig. 4b) in the FHWT cell lines. Notably, we found expression of _TRIP13_ (Thyroid Hormone Receptor Interactor 13) to be downregulated in KPT-330 treated cells. TRIP13 has been
associated with G2/M arrest45. In addition, TRIP13 previously was found to interact with co-factors of p53 in injured renal epithelial cells46 and more recently, has been identified as a
cancer predisposition gene in WT47. We then focused on evaluating the functional role of TRIP13 in our FHWT cell lines. At the basal level, pediatric renal tumors generally had a modest and
significant increase in expression of _TRIP13_ (Supplementary Fig. 5d) as compared to adjacent normal kidney controls. We then suppressed the expression of _TRIP13_ with RNAi in p53
wild-type FHWT cells using two different _TRIP13_ shRNA constructs (“Methods”). To mitigate off-target effects, we used a seed control to one shRNA construct and an RFP non-targeting
control48. Our cell viability results showed significant cell death in our cell lines transduced with either _TRIP13_ shRNA constructs (14–59% cell viability; Fig. 4e and Supplementary Fig.
5e–g). In addition, when we overexpressed TRIP13 in our FHWT cell lines, we found a modest 15–27% increase in cell counts as compared to a luciferase overexpression control (Supplementary
Fig. 5h). Previous observations in patient-derived lymphoblasts from a patient with Wilms tumor which harbor a loss of function mutation in _TRIP13_ have reported a decrease in proliferation
upon TRIP13 overexpression. Other studies looking at cancers such as glioblastoma, colorectal carcinoma, osteosarcoma, non-small cell lung cancer, and hepatocellular carcinoma have shown
that increased _TRIP13_ expression led to increased proliferation, migration, and invasion49,50,51,52,53. These findings suggest TRIP13 has differing roles in different lineages and
contexts. Here, in our tumor cells which do not harbor _TRIP13_ mutations, we see that suppression of _TRIP13_ leads to decreased viability. We found TRIP13 levels increased in our _TP53_
deleted cells and were unchanged upon KPT-330 treatment when compared to a non-targeting control (Supplementary Fig. 5i). Collectively, these results suggest that FHWT (e.g., _TP53_
wild-type) cells require TRIP13 for survival and when overexpressed, lead to a modest increase in proliferation. We then assessed the transcriptional changes seen upon _TRIP13_ suppression
by RNA sequencing. We used the PEDS_0041_T1 and Aflac_2377T cell lines to determine the consequences of suppressing _TRIP13_ as compared to our non-targeting shRNA controls (“Methods”). We
found 789 differentially expressed genes (Fig. 4f and Supplementary Data 5). We used GSEA to identify gene sets significantly enriched upon suppression of _TRIP13_. We found the gene sets
involved in E2F as significantly downregulated which was confirmed with decreased cyclin D1 levels (Fig. 4f, g, Supplementary Fig. 5e–g, and Supplementary Data 6)54. We further found,
similar to XPO1 inhibition, that suppression of _TRIP13_ is anti-correlated with the G2/M checkpoint (Fig. 4g). These findings suggest that suppression of _TRIP13_ acts similarly to
treatment with KPT-330. We subsequently asked how suppression of TRIP13 contributed to the phenotypes seen when FHWT cells were treated with KPT-330. We looked at the overlap between
differentially expressed genes from our RNA-sequencing experiments (Fig. 4h and Supplementary Data 7). We found 46 genes upregulated and 20 genes downregulated. Interestingly, upregulated
genes _MYCT1_, and _SAMD9_ have been implicated as tumor suppressors in other cancers such as hematologic malignancies55,56,57. Furthermore, an over-representation analysis identified an
immune response (FDR 1.66e-3). When we evaluated the downregulated genes with _TRIP13_ suppression, we found genes involved in the cell cycle such as _ASPM_ and _CDCA7_. ASPM is essential
for mitotic spindle function in neurons58 and CDCA7 regulates CCNA2, a cyclin with roles in G1 and G2/M, in esophageal squamous cell carcinomas59. This was further confirmed when we
performed an over-representation test (FDR 1.94e-2). In sum, our findings show that treatment with KPT-330 in part leads to suppression of TRIP13 which in turn leads to alterations in the
cell cycle. KPT-330 AND DOXORUBICIN ARE SYNERGISTIC IN VITRO AND IN VIVO Doxorubicin was added to vincristine and dactinomycin in Stage III FHWT patients in the 1980s to account for higher
risk disease (e.g., pulmonary metastasis or tumor rupture)60. Despite improved overall survival with the addition of doxorubicin in patients with higher risk FHWT, these cancers recur in 15%
of patients7. Over the past two decades, only one Phase II clinical trial for relapsed FHWT has been opened (clinicaltrials.gov: NCT04322318). This trial uses a chemotherapy backbone
without novel therapeutic targets. Thus, there is a need to identify potential synergistic combination strategies which could be tested in the Phase I/II setting. We first assessed the role
of adding KPT-330 to vincristine, dactinomycin or doxorubicin to determine if there was synergy or additivity with our cell line models of FHWT as measured by CellTiter-Glo. We found the
combination of KPT-330 with vincristine or actinomycin with KPT-330 was not synergistic whereas KPT-330 had an additive to synergistic effect with doxorubicin (Fig. 5a). We then evaluated
doxorubicin sensitivity in these patient-derived WT cell lines. We found that all WT were sensitive to doxorubicin with IC50s in the low nanomolar range (~40–256 nM) as compared to the
normal cell lines which had an IC50 range of 131–516 nM (Fig. 5b and Supplementary Fig 6; _P_ value 0.0134). Subsequently, we evaluated the effect of combination KPT-330 and doxorubicin
treatment across our cell lines. The combination was synergistic (e.g., Bliss and ZIP scores >10) in 25% of cell lines tested (3 out of 12) across multiple concentrations of KPT-330 and
doxorubicin (Fig. 5c). For the remaining 9 tumor cell lines, two of which were DAWT, the combination was found to be additive with synergy scores ranging from 1 to 9. In contrast, this
combination was not synergistic in the normal cell lines with scores ranging from −5 to −12 (Fig. 5c). Taken together, we found a synergistic interaction between KPT-330 and doxorubicin in
FHWT as compared to normal kidney cell lines. We followed up these in vitro studies with in vivo studies to further validate the potential synergistic effect of KPT-330 and doxorubicin. Of
our patient samples, we were able to generate PDXs from both PEDS_0041 and PEDS_0023. Given that PEDS_0023 has features of anaplastic Wilms tumor, we performed our in vivo studies with the
PDX from PEDS_0041, a patient with FHWT. Further the cell line derived from this PDX (PEDS_0041_T2) displayed a synergistic interaction between doxorubicin and KPT-330 (Fig. 5d).
Interestingly, the PEDS_0041_T1 cell line and PEDS_0041_T2 were some of the least sensitive WT cell lines to KPT-330 and doxorubicin treatments (Figs. 3d and 5d). We treated tumor xenografts
with placebo, doxorubicin, KPT-330, or the combination of doxorubicin and KPT-330 for 28 days and then monitored tumor growth until endpoints were reached or after 150 days following
treatment initiation. At 22 days, we compared tumor volume of treatment arms to vehicle. We found that treatment with KPT-330 led to a 49% decrease (_P_ value = 0.046), doxorubicin led to
87% decrease (_P_ value <0.005) and the combination led to 99% decrease (_P_ value < 0.005) in tumor volume with similar findings when comparing log2 fold change (Fig. 5e). We then
assessed the effects following cessation of therapy. We found that monotherapy with KPT-330 or doxorubicin led to a median survival of 39 and 53.5 days, respectively (Fig. 5f, g). Further,
the combination of KPT-330 and doxorubicin had an undefined median survival with five of eight mice exhibiting a complete response (Fig. 5f, g). Taken together, these observations suggest
that inhibition of XPO1 in combination with doxorubicin chemotherapy is synergistic in vivo. DISCUSSION Here, we have developed short-term cell line models of Wilms Tumor that maintain a
faithful representation of their genomics and transcriptomics (Figs. 1 and 2). Further, we show that they can be used to perform systematic loss-of-function studies (Fig. 3). We find that
integration of targeted RNA interference and CRISPR-Cas9 screens identifies the nuclear export apparatus as a therapeutic target in FHWT. XPO1 regulates a spectrum of cellular processes by
controlling the active transport of over 200 proteins out of the nucleus, including tumor suppressors (TSs) and transcription factors (TFs) (such as TOP2A, p53, WTX, APC, Rb, and PI3K/AKT)3.
Due to its important physiological role, inhibition of XPO1 by the small molecule inhibitor KPT-330 (selinexor) is widely being tested in phase I and phase II clinical trials in multiple
malignancies and has FDA approval in several hematologic malignancies4,5. As part of the Pediatric Preclinical Testing Program, KPT-330 was tested as a single agent across a panel of
pediatric hematologic and solid tumor PDXs which included several WT xenografts61. In this study, the group used KPT-330 in 3 WT PDXs. They found one PDX (KT-10) had maintained a complete
response at 42 days post-treatment initiation while the other two had partial responses. However, mechanisms and biomarkers remain unknown. Here, we show that inhibition of nuclear export
leads to primarily a G2/M arrest in FHWT and loss of p53 leads to significant resistance to KPT-330 (Fig. 3). While the role of p53 in response to KPT-330 has been previously reported62, we
identified that suppression or inhibition of nuclear export leads to suppression of TRIP13 in FHWT. We find that although TRIP13 is traditionally thought to affect G2/M50,63, loss of TRIP13
affects both G1 and G2/M in FHWT (Fig. 4). Furthermore, when we compare the differentially expressed genes between KPT-330 treated cells as compared to cells with _TRIP13_ suppression, we
find several potential tumor suppressors such as _MYCT1_ and _SAMD9_, a gene having antiproliferative properties, whose expression increases. Future studies will explore these findings to
better elucidate the function of TRIP13 in FHWT in immune response and cell cycle. Finally, we show that there is significant synergy when KPT-330 is combined with the current standard of
care topoisomerase II inhibitor, doxorubicin, in vivo where five of eight mice achieved cure following a single cycle of combination therapy (Fig. 5). We have observed that the DAWT models
used in this study, PEDS_0023_T1 and T2, are sensitive to nuclear export inhibition and have aberrant TP53 function. To discern if nuclear mutant _TP53_ accumulation is driving this response
in DAWT, we attempted to delete _TP53_ using CRISPR-Cas9 similar to PEDS1012T and PEDS_0022T. We observed that these models were unable to tolerate deletion of _TP53_, suggesting that these
cells rely on mutant p53 for their proliferation. Together these observations suggest that PEDS_0023_T may have another mechanism through which nuclear export inhibition functions. Further
studies with additional models of different p53 mutants are needed to fully characterize the molecular mechanisms driving KPT-330 activity in DAWT. Finally, there are limitations to our
studies. First, these studies utilized short-term patient-derived cell lines. Some features found in patient tumors are likely lost or enriched in the cultured environment, particularly at
later passages. Future studies to dissect out the triphasic biology at single cell resolution are needed. In addition, limited expansion of these cell lines prevents high throughput studies
across other cell lines included in this study. The use of immortalization techniques may alter our assessment of the biology of WT so identifying growth factors or cytokines to support in
vitro growth is needed. Another limitation of this study is the genetic heterogeneity identified within the tumor and normal samples used in this study. We have transcriptionally confirmed
our samples and respective cell lines represent Wilms tumor or normal kidney tissue; however this does not elucidate the mechanisms by which mosaicism and genetic heterogeneity may drive
disease pathogenesis in Wilms tumor. Further studies will be needed to assess these features of Wilms tumor in in vitro and in vivo settings. Taken together, we have identified that KPT-330
acts in part by inhibiting TRIP13 in Favorable Histology Wilms Tumor. In combination with a topoisomerase II inhibitor, nuclear export inhibitors could be studied in patients with high-risk
FHWT. METHODS DEVELOPMENT OF PATIENT-DERIVED WILMS TUMOR CELL LINES Tumor cells were isolated from tumor and if available, adjacent normal kidneys tissues of patients with a pathological
diagnosis of Wilms Tumor. Samples were obtained with informed consent under IRB approved protocols from patients at either DanaFarber/Boston Children’s Cancer and Blood Disorders Center or
Aflac Cancer and Blood Disorders Center at the Children’s Healthcare of Atlanta and Emory University School of Medicine. Tumor tissue or adjacent normal tissue was finely minced into 1–2 mm3
pieces and cell lines were established using F-media (Supplementary Table 1)22. Cells were detached for passaging using TrypLE (Gibco, 12605036). Samples were then plated in six-well
plates. Cells were serially passaged after reaching confluency of 70–80%. For PDX cell lines, a tumor fragment (<5 mm in diameter) was implanted subcutaneously into NSG mice and once
passaged twice, a tumor fragment was then minced and fragmented on a six-well dish to develop a PDX cell line. In general, Wilms Tumor cells were grown for up to 30 passages and normal
kidney cells were grown for approximately 12–15 passages before senescing (Supplementary Table 2). Cell lines were tested for mycoplasma contamination using the MycoAlert Mycoplasma
Detection Kit (Lonza, LT07-118) and were negative. All ethical regulations relevant to human research participants were followed. LOW COVERAGE WHOLE GENOME SEQUENCING (WGS) AND COPY NUMBER
ANALYSES DNA from tumor tissue, normal tissue if available or blood, and cell pellets from our patient-derived cell lines was extracted (NEB, T3010S). Genomic libraries were prepared
(Illumina) by Novogene Inc. and sequenced at 1× on Novoseq 6000 (Illumina). Fastq or BAM files were mapped and aligned using Illumina Dragen v3.7.5 to GrCh38 on Amazon Web Services. Copy
number alterations (amplification, gain, deletion) were assessed with ichorCNA64 and TITAN65. Loss of heterozygosity was assessed using TITAN. WHOLE EXOME SEQUENCING WES was performed using
DNA as described above. For five patients, WES was performed at the Broad Institute Genomics Platform using HiSeq 2000 (Illumina). WES libraries were based on an Illumina Customized Exon
(ICE) array. For three patients, WES was performed at Novogene Inc using Novoseq 6000 (Illumina). Libraries were based on an Agilent array. Sample coverage was >100× for tumor and >50×
for normal. Fastq or BAM files were mapped and aligned using Illumina Dragen v3.7.5 to GrCh3866 on Amazon Web Services. Variant call files (vcf) were subsequently processed with OpenCRAVAT
v2.4.2 using cancer hotspots, cancer gene landscape, chasm plus, civic, cosmic, mutpanning and NDEX NCI to filter for pathogenic variants. RNA SEQUENCING RNA was extracted from samples
collected from tumor, adjacent normal kidney and cell lines (Qiagen RNeasy or NEB Monarch Total RNA). For mechanistic studies, RNA was collected in biological replicates from cells treated
with shRNAs or compounds as listed in the figures. Libraries were prepared using Illumina TruSeq. Samples were run with at least 50 million paired-end reads using Novoseq 6000 (Illumina).
Fastq or BAM files were mapped and aligned using Illumina Dragen v3.7.5 to GrCh3866 and GenCode3667 on Amazon Web Services. Samples were then quantified using salmon through Illumina Dragen
v3.7.5. Gene count files were converted to a counts matrix using tximport68. TARGET clustering analysis in Fig. 2a utilized ComBat-seq69 to correct for batch effects between studies. The
counts matrix was used as input into DESeq2 to evaluate differential gene expression70. Log2 fold change values were used as input into GSEA42 to measure gene set enrichment. Normalized read
counts matrices were used as input into UMAP28 to visualize clustering between samples. UMAP analysis was performed on the 1000 most highly variable genes in Wilms tumor as previously
identified71. Versions used: R v4.2.2; R Studio 2022.07.1 Build 554; tximport v1.26.1, sva (ComBat-seq) v3.46.0, DESeq2 v1.38.3, umap v0.2.10.0, ggplot v3.4.2, Hmisc v4.6-0 (R packages);
fgsea_1.24.0. LOSS OF FUNCTION SCREENS—METHODOLOGY AND ANALYSES RNAi and CRISPR-Cas9 screens were performed as previously described20,21,22. Specifically, we transduced into noted cell lines
the DCT v1.0 libraries: shRNA (CP1050) and sgRNA (CP0026) libraries from the Broad Institute Genetic Perturbation Platform (GPP) (http://www.broadinstitute.org/rnai/public/). In parallel,
PEDS_0023_T, PEDS_0023_T2, and PEDS_0002T cells were transduced with Cas9 expression vector pLX311_Cas972. These stable cells were subsequently transduced using the DCT v2.0 libraries and at
a multiplicity of infection (MOI) between 0.3 and 0.632. Screens were performed with a goal representation rate of >500 cells per shRNA or sgRNA. Cells were passaged every 5–7 days until
days 25–29 following transduction. Genomic DNA was extracted from an early time point and at the end time point. Samples were sequenced as previously described, deconvoluted and analyzed
using MAGeK33. CELL VIABILITY ASSAYS Cells were plated in a concentration of 2500 cells/well in a 96-well plate (Corning, 3903, or Greiner Bio-One, 655098) and were incubated overnight in
F-media. After 24 h, F-media was aspirated and F-media with the indicated drug concentration was added. Cells were treated between the range of 0.007 μM to 50 μM of KPT-330 (Selleck, S7252)
and doxorubicin (Selleck, S1208) for 72 h. Cell viability was measured using CellTiter-Glo (Promega, G7573). Luminescence measured with BioTek Synergy MX with BioTek Gen5 v1.11.5 or Perkin
Elmer 2103 with EnVision Manager 1.14.3049.1193. Cell viability was normalized to the luminescence of the control sample, with luminescence values of zero serving as 0% viability and the
average luminescence value of the technical replicates of the control sample serving as 100% viability. IC50s of KPT-330 and doxorubicin were determined in each cell line by fitting the dose
response curve using Graphpad Prism v9.3.0. Each experiment was repeated in at least two biological replicates. _XPO1_ AND _TRIP13_ SHRNA EXPERIMENTS shRNA sequences were designed using the
Broad Institute GPP portal (http://www.broadinstitute.org/rnai/public/). Oligos were obtained from Integrated DNA Technologies (IDT). Oligos were annealed and ligated with pLKO.5 as
previously described. Constructs were transfected using TransIT-LT1 (Mirus Bio LLC, Madison, WI, USA) and HEK 293T cells. Cells were then transduced with lentivirus to achieve appropriate
knockdown without viral toxicity as previously described21. Following selection with puromycin (Invivogen), cell proliferation was assayed by CellTiter-Glo at 10 days. Experiments were
repeated a minimum of three times in technical triplicates. shRNA sequences can be found in Supplementary Table 4. CELL CYCLE ANALYSIS WITH FLOW CYTOMETRY Cells were grown to 70–80%
confluency and were treated or transduced as indicated in the figures. After treatment, cells were harvested and washed with cold PBS before being fixed in 70% ethanol for two hours on ice.
Cells were stained with PI/RNase solution (Invitrogen, F10797) for fifteen minutes before being analyzed via flow cytometry. Data was collected with Beckman Coulter CytExpert v2.3. Data was
analyzed in FlowJo v10.8.1. First, debris was removed via gating under FSC-A/SSC-A, singlets were gated with FSC-A/FSC-H, and then cell cycle phases were determined using the Watson
(Pragmatic) model (Supplementary Fig. 7). _TP53_ CRISPR-CAS9 DELETION PEDS1012T and PEDS_0022T cells were transduced with Cas9 expression vector pLX311_Cas9. These cells were subsequently
transduced with sgTP53-1 and sgLacZ in the pXPR003 backbone. Transduced cells were then cultured in the indicated antibiotics for selection and suppression of gene expression was confirmed
by immunoblotting. QRT-PCR Total RNA was extracted using the NEB Monarch RNA extraction kit. One microgram of RNA and oligo primers were used for cDNA synthesis in a total reaction volume of
20 µL High-Capacity cDNA Reverse Transcription Kit (ThermoFisher Scientific, 4368814). qRT-PCR reactions were prepared using SYBR-Green PCR Master Mix (ThermoFisher Scientific, 4367659) and
run on a BioRad CFX96 qPCR System/BioRad CFX Manager v3.1 with a minimum of technical duplicates. Relative mRNA levels were calculated using the 2-ΔΔCt method73. Results shown are
representative of at least two biological replicates. Primer sequences can be found in Supplementary Table 4. IMMUNOBLOTS Cells were grown to 70–80% confluency and were treated or transduced
as indicated in the figures. Thereafter, cells were lysed using 1× RIPA (Cell Signaling Technologies, 9806) with protease inhibitors (coMplete, Roche, 42484600) and phosphatase inhibitors
(PhosSTOP, Roche, 04906837001). For experiments using nuclear and cytoplasmic fractions, fractions were extracted using NE-PER (ThermoFisher Scientific, 78835). Using 10% or 4–12% SDS-PAGE
gels, gels were transferred onto PVDF (Millipore, IPFL00010) or nitrocellulose membranes (ThermoFisher Scientific, IB23001). Blots were then visualized using Odyssey Classic v3.0.30 (Licor,
Lincoln, NE). Antibodies used in this study include: XPO1 (Santa Cruz; sc-5595), β-Actin (C-4) (Santa Cruz; sc-47778), β-Actin (Cell Signaling; 8457), p53 (Santa Cruz; sc-126), TRIP13
(Abcam; ab128171), α-Tubulin (Santa Cruz, sc-5286), α-Tubulin (Cell Signaling; 2144), Lamin A/C (Cell Signaling; 4777 or 2032), p21 (Cell Signaling; 29475), CCND1 (Santa Cruz; sc-8396).
Results shown are representative of at least two biological replicates. SYNERGY EXPERIMENTS WT cells were plated in 96-well plates at a concentration of 2500 cells/well. WT cells were
treated with either doxorubicin (62.5–500 nM), vincristine (62.5–500 nM), or actinomycin (62.5– 500 nM) in combination with KPT-330 (1.25–10 μM). Each plate included a 4-dose dilution of
each drug alone or in combination and included DMSO controls. Following a 3-day incubation, we performed a cell viability assay using CellTiter-Glo. Relative cell viabilities were then
calculated following the procedure listed previously in cell viability assays and analyzed with SynergyFinder74 using two alternate drug–drug interaction models: zero interaction potency
(ZIP)75 and Bliss independence76. Experiments were performed in biological replicates or triplicates. Raw data for calculations can be found in Supplementary Data 8. IN VIVO EXPERIMENTS
Female NSG (NOD-scid IL2Rgamma null) mice, 6 weeks old were purchased from The Jackson Laboratory (Bar Harbor, ME). Animals were acclimated for at least 5 days before initiation of the
study. The study was conducted at Dana-Farber Cancer Institute (DFCI) with the approval of the Institutional Animal Care and Use Committee in an AAALAC accredited vivarium. NSG mice were
implanted with CCLF_PEDS_0041_T2 tumor fragments from previously expanded tumors, subcutaneously in the hind-flank. Tumors were allowed to establish to 86– 163 mm3 (average 114.3 mm3) in
size before randomization using Studylog software (San Francisco, CA) into various treatment groups with 8 mice/group as follows: vehicle control (0.6% Pluronic F-68 + 0.6% Plasdone PVP;
oral gavage on MWF × 4 weeks), selinexor (purchased from Selleck Chemicals LLC, 15 mg/kg oral gavage on MWF × 4 weeks), doxorubicin (obtained from DFCI pharmacy, 5 mg/kg intravenous
injection on days 2 and 9) and the combination of selinexor and doxorubicin. No statistical methods were used to pre-determine sample sizes, but our sample sizes are similar to those
reported in previous publications21,22,77. Once the treatment was completed, tumors were monitored at least once a week. For single agent and combination agent efficacy studies, mice were
dosed for 21 or 28 days and monitored daily. Drugs were then withdrawn, and tumors were monitored twice weekly until study termination on day 150. Tumor volumes were calculated using the
following formula: (mm3) = length × width × width × 0.5. Mice were immediately euthanized if the tumor volume exceeded 2000 mm3 or if the tumors became necrotic or ulcerated. The compounds
were well tolerated with less than 8% body weight loss. Data collection and analysis were not performed blind to the conditions of the experiments. GraphPad Prism v9.3.0 was used to
calculate significance in the Kaplan–Meier curves. We have complied with all relevant ethical regulations for animal use. STATISTICS AND REPRODUCIBILITY Comparisons between two groups were
statistically evaluated by the student’s paired or unpaired _t_ test, where applicable. Significance for Kaplan–Meier curves was performed using the log-rank (Mantel–Cox) test. Statistical
significance for tumor volume between groups was performed using a one-way ANOVA test. Differences were considered significant at _P_ < 0.05. Statistical analysis of RNA sequencing data
was performed using DESeq2. All genes that had an FDR < 0.05 and a log2FoldChange > |1| were considered significant. At least two independent experiments with at least two technical
replicates were performed to support statistically analyzed findings. REPORTING SUMMARY Further information on research design is available in the Nature Portfolio Reporting Summary linked
to this article. DATA AVAILABILITY Sequence data have been deposited at the European Genome-phenome Archive (EGA), which is hosted by the EBI and the CRG, under accession number
EGAS00001007389. This study does not use custom code or mathematical algorithms. The uncropped immunoblotting images were exhibited in Supplementary Fig. 8. The source data of the graph
figures are exhibited in Supplementary Data 8. Plasmids herein can be found at https://www.addgene.org/Andrew_Hong/. All other data is available from the corresponding author upon reasonable
request. REFERENCES * Nakata, K. et al. Incidence of childhood renal tumours: an international population-based study. _Int. J. Cancer_ 147, 3313–3327 (2020). Article CAS PubMed PubMed
Central Google Scholar * Smith, M. A., Altekruse, S. F., Adamson, P. C., Reaman, G. H. & Seibel, N. L. Declining childhood and adolescent cancer mortality. _Cancer_ 120, 2497–2506
(2014). Article PubMed Google Scholar * Spreafico, F. et al. Wilms tumour. _Nat. Rev. Dis. Primers_ 7, 75 (2021). Article PubMed Google Scholar * Axt, J. et al. Race disparities in
Wilms tumor incidence and biology. _J. Surg. Res._ 170, 112–119 (2011). Article PubMed PubMed Central Google Scholar * Siegel, R. L., Miller, K. D., Fuchs, H. E. & Jemal, A. Cancer
statistics, 2021. _CA Cancer J. Clin._ 71, 7–33 (2021). Article PubMed Google Scholar * Daw, N. C. et al. Activity of vincristine and irinotecan in diffuse anaplastic Wilms tumor and
therapy outcomes of stage II to IV disease: results of the Children’s Oncology Group AREN0321 Study. _J. Clin. Oncol._ 38, 1558–1568 (2020). Article CAS PubMed PubMed Central Google
Scholar * Fernandez, C. V. et al. Outcome and prognostic factors in stage III favorable-histology Wilms tumor: a report from the Children’s Oncology Group Study AREN0532. _J. Clin. Oncol._
36, 254–261 (2018). Article CAS PubMed Google Scholar * Jain, J., Sutton, K. S. & Hong, A. L. Progress update in pediatric renal tumors. _Curr. Oncol. Rep._ 23, 33 (2021). Article
PubMed Google Scholar * Garvin, A. J., Re, G. G., Tarnowski, B. I., Hazen-Martin, D. J. & Sens, D. A. The G401 cell line, utilized for studies of chromosomal changes in Wilms’ tumor,
is derived from a rhabdoid tumor of the kidney. _Am. J. Pathol._ 142, 375–380 (1993). CAS PubMed PubMed Central Google Scholar * Pritchard-Jones, K. & Perotti, D. WARNING: G-401 and
SK-NEP-1 cell lines are not Wilms tumor cell lines. _Pediatr. Blood Cancer_ 66, e27741 (2019). Article PubMed Google Scholar * Smith, M. A. et al. SK-NEP-1 and Rh1 are Ewing family tumor
lines. _Pediatr. Blood Cancer_ 50, 703–706 (2008). Article PubMed Google Scholar * Stroup, E. et al. WT-CLS1 is a rhabdoid tumor cell line and can be inhibited by miR-16. _Cancer Rep._ 2,
e1110 (2018). Article Google Scholar * Brandt, A. et al. Establishment of a conditionally immortalized Wilms tumor cell line with a homozygous WT1 deletion within a heterozygous 11p13
deletion and UPD limited to 11p15. _PLoS ONE_ 11, e0155561 (2016). Article PubMed PubMed Central Google Scholar * Royer-Pokora, B. et al. Wilms tumor cells with WT1 mutations have
characteristic features of mesenchymal stem cells and express molecular markers of paraxial mesoderm. _Hum. Mol. Genet._ 19, 1651–1668 (2010). Article CAS PubMed Google Scholar *
Royer-Pokora, B. et al. Comprehensive biology and genetics compendium of Wilms tumor cell lines with different WT1 mutations. _Cancers_ 13, 60 (2020). Article PubMed PubMed Central Google
Scholar * Wegert, J. et al. Characterization of primary Wilms tumor cultures as an in vitro model. _Genes Chromosomes Cancer_ 51, 92–104 (2012). Article CAS PubMed Google Scholar *
Calandrini, C. et al. An organoid biobank for childhood kidney cancers that captures disease and tissue heterogeneity. _Nat. Commun._ 11, 1310 (2020). Article CAS PubMed PubMed Central
Google Scholar * Murphy, A. J. et al. Forty-five patient-derived xenografts capture the clinical and biological heterogeneity of Wilms tumor. _Nat. Commun._ 10, 5806 (2019). Article CAS
PubMed PubMed Central Google Scholar * Ooms, A. H. A. G., Calandrini, C., de Krijger, R. R. & Drost, J. Organoid models of childhood kidney tumours. _Nat. Rev. Urol._ 17, 311–313
(2020). Article CAS PubMed Google Scholar * Dharia, N. V. et al. A first-generation pediatric cancer dependency map. _Nat. Genet._ 53, 529–538 (2021). Article CAS PubMed PubMed
Central Google Scholar * Hong, A. L. et al. Integrated genetic and pharmacologic interrogation of rare cancers. _Nat. Commun._ 7, 11987 (2016). Article CAS PubMed PubMed Central Google
Scholar * Hong, A. L. et al. Renal medullary carcinomas depend upon SMARCB1 loss and are sensitive to proteasome inhibition. _eLife_ 8, e44161 (2019). Article PubMed PubMed Central
Google Scholar * Chagtai, T. et al. Gain of 1q as a prognostic biomarker in Wilms tumors (WTs) treated with preoperative chemotherapy in the International Society of Paediatric Oncology
(SIOP) WT 2001 trial: a SIOP Renal Tumours Biology Consortium Study. _J. Clin. Oncol._ 34, 3195–3203 (2016). Article CAS PubMed PubMed Central Google Scholar * Gratias, E. J. et al.
Association of chromosome 1q gain with inferior survival in favorable-histology Wilms tumor: a report from the Children’s Oncology Group. _J. Clin. Oncol._ 34, 3189–3194 (2016). Article
PubMed PubMed Central Google Scholar * Gadd, S. et al. A Children’s Oncology Group and TARGET initiative exploring the genetic landscape of Wilms tumor. _Nat. Genet._ 49, 1487–1494
(2017). Article CAS PubMed PubMed Central Google Scholar * Gadd, S. et al. Genetic changes associated with relapse in favorable histology Wilms tumor: a Children’s Oncology Group
AREN03B2 study. _Cell Rep. Med._ 3, 100644 (2022). Article CAS PubMed PubMed Central Google Scholar * Cresswell, G. D. et al. Intra-tumor genetic heterogeneity in Wilms tumor: clonal
evolution and clinical implications. _EBioMedicine_ 9, 120–129 (2016). Article PubMed PubMed Central Google Scholar * McInnes, L. et al. UMAP: uniform manifold approximation and
projection for dimension reduction. _J. Open Source Softw._ 3, 861 (2018). * Murphy, A. J. et al. SIX2 and CITED1, markers of nephronic progenitor self-renewal, remain active in primitive
elements of Wilms’ tumor. _J. Pediatr. Surg._ 47, 1239–1249 (2012). Article PubMed PubMed Central Google Scholar * Walz, A. L. et al. Recurrent DGCR8, DROSHA, and SIX homeodomain
mutations in favorable histology Wilms tumors. _Cancer Cell_ 27, 286–297 (2015). Article CAS PubMed PubMed Central Google Scholar * Wegert, J. et al. Mutations in the SIX1/2 pathway and
the DROSHA/DGCR8 miRNA microprocessor complex underlie high-risk blastemal type Wilms tumors. _Cancer Cell_ 27, 298–311 (2015). Article CAS PubMed Google Scholar * Harris, I. S. et al.
Deubiquitinases maintain protein homeostasis and survival of cancer cells upon glutathione depletion. _Cell Metab._ 29, 1166–1181.e6 (2019). Article CAS PubMed PubMed Central Google
Scholar * Li, W. et al. MAGeCK enables robust identification of essential genes from genome-scale CRISPR/Cas9 knockout screens. _Genome Biol._ 15, 554 (2014). Article PubMed PubMed
Central Google Scholar * Kasamon, Y. L. et al. FDA approval summary: selinexor for relapsed or refractory diffuse large B-cell lymphoma. _Oncologist_ 26, 879–886 (2021). Article CAS
PubMed PubMed Central Google Scholar * Coutinho, D. F. et al. Validation of a non-oncogene encoded vulnerability to exportin 1 inhibition in pediatric renal tumors. _Med_ 3, 774–791.e7
(2022). Article CAS PubMed Google Scholar * Bjork, I., Peralez, J., Haussler, D., Spunt, S. L. & Vaske, O. M. Data sharing for clinical utility. _Cold Spring Harb. Mol. Case Stud._
5, a004689 (2019). Article CAS PubMed PubMed Central Google Scholar * Azmi, A. S., Uddin, M. H. & Mohammad, R. M. The nuclear export protein XPO1—from biology to targeted therapy.
_Nat. Rev. Clin. Oncol._ 18, 152–169 (2021). Article CAS PubMed Google Scholar * Razak, A. R. A. et al. First-in-class, first-in-human phase I study of selinexor, a selective inhibitor
of nuclear export, in patients with advanced solid tumors. _J. Clin. Oncol._ 34, 4142–4150 (2016). Article PubMed PubMed Central Google Scholar * Tai, Y. T. et al. CRM1 inhibition
induces tumor cell cytotoxicity and impairs osteoclastogenesis in multiple myeloma: molecular mechanisms and therapeutic implications. _Leukemia_ 28, 155–165 (2014). Article CAS PubMed
Google Scholar * Breit, M. N. et al. Biologic activity of the novel orally bioavailable selective inhibitor of nuclear export (SINE) KPT-335 against canine melanoma cell lines. _BMC Vet.
Res._ 10, 160 (2014). Article PubMed PubMed Central Google Scholar * Cui, Y.-r et al. KPT330 improves Cas9 precision genome- and base-editing by selectively regulating mRNA nuclear
export. _Commun. Biol._ 5, 237 (2022). Article CAS PubMed PubMed Central Google Scholar * Subramanian, A. et al. Gene set enrichment analysis: a knowledge-based approach for
interpreting genome-wide expression profiles. _Proc. Natl. Acad. Sci. USA_ 102, 15545–15550 (2005). Article CAS PubMed PubMed Central Google Scholar * Gravina, G. L. et al. KPT-330, a
potent and selective exportin-1 (XPO-1) inhibitor, shows antitumor effects modulating the expression of cyclin D1 and survivin [corrected] in prostate cancer models. _BMC Cancer_ 15, 941
(2015). Article PubMed PubMed Central Google Scholar * Etchin, J. et al. KPT-330 inhibitor of CRM1 (XPO1)-mediated nuclear export has selective anti-leukaemic activity in preclinical
models of T-cell acute lymphoblastic leukaemia and acute myeloid leukaemia. _Br. J. Haematol._ 161, 117–127 (2013). Article CAS PubMed PubMed Central Google Scholar * Zhang, Q. et al.
The oncogenic role of TRIP13 in regulating proliferation, invasion, and cell cycle checkpoint in NSCLC cells. _Int. J. Clin. Exp. Pathol._ 12, 3357–3366 (2019). CAS PubMed PubMed Central
Google Scholar * Pressly, J. D., Hama, T., Brien, S. O., Regner, K. R. & Park, F. TRIP13-deficient tubular epithelial cells are susceptible to apoptosis following acute kidney injury.
_Sci. Rep._ 7, 43196 (2017). Article PubMed PubMed Central Google Scholar * Yost, S. et al. Biallelic TRIP13 mutations predispose to Wilms tumor and chromosome missegregation. _Nat.
Genet._ 49, 1148–1151 (2017). Article CAS PubMed PubMed Central Google Scholar * Buehler, E., Chen, Y. C. & Martin, S. C911: a bench-level control for sequence specific siRNA
off-target effects. _PLoS ONE_ 7, e51942 (2012). Article CAS PubMed PubMed Central Google Scholar * Zhang, G. et al. TRIP13 promotes the cell proliferation, migration and invasion of
glioblastoma through the FBXW7/c-MYC axis. _Br. J. Cancer_ 121, 1069–1078 (2019). Article CAS PubMed PubMed Central Google Scholar * Sheng, N. et al. TRIP13 promotes tumor growth and is
associated with poor prognosis in colorectal cancer. _Cell Death Dis._ 9, 402 (2018). Article PubMed PubMed Central Google Scholar * Yu, D.-C. et al. TRIP13 knockdown inhibits the
proliferation, migration, invasion, and promotes apoptosis by suppressing PI3K/AKT signaling pathway in U2OS cells. _Mol. Biol. Rep._ 49, 3055–3064 (2022). Article CAS PubMed Google
Scholar * Lu, R. et al. Upregulation of TRIP13 promotes the malignant progression of lung cancer via the EMT pathway. _Oncol. Rep._ 46, 1–10 (2021). * Yao, J. et al. Silencing TRIP13
inhibits cell growth and metastasis of hepatocellular carcinoma by activating of TGF-β1/smad3. _Cancer Cell Int._ 18, 208 (2018). Article CAS PubMed PubMed Central Google Scholar *
Lara, M. F. et al. Gene profiling approaches help to define the specific functions of retinoblastoma family in epidermis. _Mol. Carcinog._ 47, 209–221 (2008). Article CAS PubMed Google
Scholar * Fu, S. et al. MYCT1-TV, a novel MYCT1 transcript, is regulated by c-Myc and may participate in laryngeal carcinogenesis. _PLoS ONE_ 6, e25648 (2011). Article CAS PubMed PubMed
Central Google Scholar * Nagamachi, A. et al. Haploinsufficiency of SAMD9L, an endosome fusion facilitator, causes myeloid malignancies in mice mimicking human diseases with monosomy 7.
_Cancer Cell_ 24, 305–317 (2013). Article CAS PubMed Google Scholar * Thanh Nha Uyen, L. et al. PCDH17 functions as a common tumor suppressor gene in acute leukemia and its
transcriptional downregulation is mediated primarily by aberrant histone acetylation, not DNA methylation. _Int. J. Hematol._ 111, 451–462 (2020). Article CAS PubMed Google Scholar *
Bond, J. et al. ASPM is a major determinant of cerebral cortical size. _Nat. Genet._ 32, 316–320 (2002). Article CAS PubMed Google Scholar * Li, H. et al. CDCA7 facilitates tumor
progression by directly regulating CCNA2 expression in esophageal squamous cell carcinoma. _Front. Oncol._ 11, 734655 (2021). Article CAS PubMed PubMed Central Google Scholar * Breslow,
N. E. et al. Doxorubicin for favorable histology, stage II-III Wilms tumor: results from the National Wilms Tumor Studies. _Cancer_ 101, 1072–1080 (2004). Article CAS PubMed Google
Scholar * Attiyeh, E. F. et al. Pharmacodynamic and genomic markers associated with response to the XPO1/CRM1 inhibitor selinexor (KPT-330): a report from the pediatric preclinical testing
program. _Pediatr. Blood Cancer_ 63, 276–286 (2016). Article CAS PubMed Google Scholar * Sun, H. et al. CRM1 inhibition promotes cytotoxicity in Ewing sarcoma cells by repressing
EWS-FLI1-dependent IGF-1 signaling. _Cancer Res._ 76, 2687–2697 (2016). Article CAS PubMed Google Scholar * Hama, T. et al. DNA damage is overcome by TRIP13 overexpression during
cisplatin nephrotoxicity. _JCI Insight_ 6, e139092 (2021). Article PubMed PubMed Central Google Scholar * Adalsteinsson, V. A. et al. Scalable whole-exome sequencing of cell-free DNA
reveals high concordance with metastatic tumors. _Nat. Commun._ 8, 1324 (2017). Article PubMed PubMed Central Google Scholar * Ha, G. et al. TITAN: inference of copy number architectures
in clonal cell populations from tumor whole-genome sequence data. _Genome Res._ 24, 1881–1893 (2014). Article CAS PubMed PubMed Central Google Scholar * Schneider, V. A. et al.
Evaluation of GRCh38 and de novo haploid genome assemblies demonstrates the enduring quality of the reference assembly. _Genome Res._ 27, 849–864 (2017). * Harrow, J. et al. GENCODE: the
reference human genome annotation for The ENCODE Project. _Genome Res._ 22, 1760–1774 (2012). Article CAS PubMed PubMed Central Google Scholar * Soneson, C., Love, M. I. & Robinson,
M. D. Differential analyses for RNA-seq: transcript-level estimates improve gene-level inferences. _F1000Res_ 4, 1521 (2015). Article PubMed Google Scholar * Zhang, Y., Parmigiani, G.
& Johnson, W. E. ComBat-seq: batch effect adjustment for RNA-seq count data. _NAR Genom. Bioinform._ 2, lqaa078 (2020). Article PubMed PubMed Central Google Scholar * Love, M. I.,
Huber, W. & Anders, S. Moderated estimation of fold change and dispersion for RNA-seq data with DESeq2. _Genome Biol._ 15, 550 (2014). Article PubMed PubMed Central Google Scholar *
Trink, A. et al. Geometry of gene expression space of Wilms’ tumors from human patients. _Neoplasia_ 20, 871–881 (2018). Article CAS PubMed PubMed Central Google Scholar * Giacomelli,
A. O. et al. Mutational processes shape the landscape of TP53 mutations in human cancer. _Nat. Genet._ 50, 1381–1387 (2018). Article CAS PubMed PubMed Central Google Scholar * Livak, K.
J. & Schmittgen, T. D. Analysis of relative gene expression data using real-time quantitative PCR and the 2(-Delta Delta C(T)) method. _Methods_ 25, 402–408 (2001). Article CAS PubMed
Google Scholar * Ianevski, A., He, L., Aittokallio, T. & Tang, J. SynergyFinder: a web application for analyzing drug combination dose-response matrix data. _Bioinformatics_ 33,
2413–2415 (2017). Article PubMed PubMed Central Google Scholar * Yadav, B., Wennerberg, K., Aittokallio, T. & Tang, J. Searching for drug synergy in complex dose-response landscapes
using an interaction potency model. _Comput. Struct. Biotechnol. J._ 13, 504–513 (2015). Article CAS PubMed PubMed Central Google Scholar * BLISS, C. I. The toxicity of poisons applied
jointly1. _Annal. Appl. Biol._ 26, 585–615 (1939). Article CAS Google Scholar * Bandopadhayay, P. et al. Neuronal differentiation and cell-cycle programs mediate response to
BET-bromodomain inhibition in MYC-driven medulloblastoma. _Nat. Commun._ 10, 2400 (2019). Article PubMed PubMed Central Google Scholar Download references ACKNOWLEDGEMENTS We thank the
patients and their families for their participation. We thank the Hong, Spangle, and Hahn labs for their thoughtful comments and suggestions. Tissue samples were provided by the Children’s
Healthcare of Atlanta Pediatric Bio-Repository or Dana-Farber/Boston Children’s Center for Cancer and Blood Disorders. Other investigators may have received specimens from the same subjects.
Research reported in this publication was supported in part by the Pediatrics/Winship Flow Cytometry Core of Winship Cancer Institute of Emory University, Children’s Healthcare of Atlanta
and NIH/NCI under award number P30CA138292, by the Bioinformatics and Systems Biology Shared and the Biostatistics Shared Resource of Winship Cancer Institute of Emory University and NIH/NCI
under award number P30CA138292. The content is solely the responsibility of the authors and does not necessarily represent the official views of the National Institutes of Health. Research
reported in this publication was supported by the following: NIGMS T32GM008490 (G.W.C.), NCI F31CA278008 (G.W.C.), DOD HT9425-23-0609 (Y.S.), NIGMS T32GM007739 (W.J.K.), NCI K08 CA2555569-01
(A.J.M.), NIH/NCI P30CA138292 (M.R.), NCI R35 CA231958 (D.M.W.), NIH R35 CA210030 (K.S.), NCI U01 CA176058 (W.C.H.), DOD W81XWH1910281 (A.L.H.), ACS MRSG-18-202-01 (A.L.H.). CureSearch for
Children’s Cancer YIA (A.L.H.), Rally for Childhood Cancer Investigator Grants 21IN12 and 22IC37 (A.L.H.). AUTHOR INFORMATION Author notes * David M. Weinstock Present address: Merck &
Co., Rahway, NJ, USA * These authors contributed equally: Karuna Mittal, Garrett W. Cooper, Benjamin P. Lee. AUTHORS AND AFFILIATIONS * Department of Pediatrics, Emory University School of
Medicine, Atlanta, GA, USA Karuna Mittal, Garrett W. Cooper, Benjamin P. Lee, Yongdong Su, Katie T. Skinner, Jenny Shim, Hunter C. Jonus, Taylor Lawrence, Manoj Bhasin, Kelly C. Goldsmith
& Andrew L. Hong * Aflac Cancer and Blood Disorders Center, Children’s Healthcare of Atlanta, Atlanta, GA, USA Karuna Mittal, Garrett W. Cooper, Benjamin P. Lee, Yongdong Su, Katie T.
Skinner, Jenny Shim, Hunter C. Jonus, Taylor Lawrence, Manoj Bhasin, Kelly C. Goldsmith & Andrew L. Hong * Winship Cancer Institute, Emory University, Atlanta, GA, USA Jenny Shim, Bhakti
Dwivedi, Manali Rupji, Manoj Bhasin, Jennifer M. Spangle, Kelly C. Goldsmith & Andrew L. Hong * Department of Medical Oncology, Dana-Farber Cancer Institute, Boston, MA, USA Won Jun
Kim, Mihir Doshi, Diego Almanza, Bryan D. Kynnap, Amanda L. Christie, David M. Weinstock & William C. Hahn * Broad Institute of MIT and Harvard, Cambridge, MA, USA Won Jun Kim, Mihir
Doshi, Diego Almanza, Bryan D. Kynnap, Xiaoping Yang, Glenn S. Cowley, Paula Keskula, Brian D. Crompton, Yuen-Yi Tseng, Jesse S. Boehm, Keith L. Ligon, David E. Root, David M. Weinstock,
Miguel N. Rivera, Kimberly Stegmaier & William C. Hahn * Experimental Therapeutics Core and Belfer Center for Applied Cancer Science, Dana-Farber Cancer Institute, Boston, MA, USA
Brittaney A. Leeper & Prafulla C. Gokhale * Department of Surgery, St. Jude Children’s Research Hospital, Memphis, TN, USA Christopher L. Morton & Andrew J. Murphy * Department of
Pediatric Oncology, Dana-Farber Cancer Institute, Boston, MA, USA Stephanie Meyer, Catherine M. Clinton, Brian D. Crompton, David M. Weinstock, Elizabeth A. Mullen & Kimberly Stegmaier *
Department of Pathology, Brigham and Women’s Hospital, Boston, MA, USA Keith L. Ligon * Department of Oncologic Pathology, Dana-Farber Cancer Institute, Boston, MA, USA Keith L. Ligon *
Department of Radiation Oncology, Emory University School of Medicine, Atlanta, GA, USA Jennifer M. Spangle * Department of Pathology, Massachusetts General Hospital, Boston, MA, USA Miguel
N. Rivera Authors * Karuna Mittal View author publications You can also search for this author inPubMed Google Scholar * Garrett W. Cooper View author publications You can also search for
this author inPubMed Google Scholar * Benjamin P. Lee View author publications You can also search for this author inPubMed Google Scholar * Yongdong Su View author publications You can also
search for this author inPubMed Google Scholar * Katie T. Skinner View author publications You can also search for this author inPubMed Google Scholar * Jenny Shim View author publications
You can also search for this author inPubMed Google Scholar * Hunter C. Jonus View author publications You can also search for this author inPubMed Google Scholar * Won Jun Kim View author
publications You can also search for this author inPubMed Google Scholar * Mihir Doshi View author publications You can also search for this author inPubMed Google Scholar * Diego Almanza
View author publications You can also search for this author inPubMed Google Scholar * Bryan D. Kynnap View author publications You can also search for this author inPubMed Google Scholar *
Amanda L. Christie View author publications You can also search for this author inPubMed Google Scholar * Xiaoping Yang View author publications You can also search for this author inPubMed
Google Scholar * Glenn S. Cowley View author publications You can also search for this author inPubMed Google Scholar * Brittaney A. Leeper View author publications You can also search for
this author inPubMed Google Scholar * Christopher L. Morton View author publications You can also search for this author inPubMed Google Scholar * Bhakti Dwivedi View author publications You
can also search for this author inPubMed Google Scholar * Taylor Lawrence View author publications You can also search for this author inPubMed Google Scholar * Manali Rupji View author
publications You can also search for this author inPubMed Google Scholar * Paula Keskula View author publications You can also search for this author inPubMed Google Scholar * Stephanie
Meyer View author publications You can also search for this author inPubMed Google Scholar * Catherine M. Clinton View author publications You can also search for this author inPubMed Google
Scholar * Manoj Bhasin View author publications You can also search for this author inPubMed Google Scholar * Brian D. Crompton View author publications You can also search for this author
inPubMed Google Scholar * Yuen-Yi Tseng View author publications You can also search for this author inPubMed Google Scholar * Jesse S. Boehm View author publications You can also search for
this author inPubMed Google Scholar * Keith L. Ligon View author publications You can also search for this author inPubMed Google Scholar * David E. Root View author publications You can
also search for this author inPubMed Google Scholar * Andrew J. Murphy View author publications You can also search for this author inPubMed Google Scholar * David M. Weinstock View author
publications You can also search for this author inPubMed Google Scholar * Prafulla C. Gokhale View author publications You can also search for this author inPubMed Google Scholar * Jennifer
M. Spangle View author publications You can also search for this author inPubMed Google Scholar * Miguel N. Rivera View author publications You can also search for this author inPubMed
Google Scholar * Elizabeth A. Mullen View author publications You can also search for this author inPubMed Google Scholar * Kimberly Stegmaier View author publications You can also search
for this author inPubMed Google Scholar * Kelly C. Goldsmith View author publications You can also search for this author inPubMed Google Scholar * William C. Hahn View author publications
You can also search for this author inPubMed Google Scholar * Andrew L. Hong View author publications You can also search for this author inPubMed Google Scholar CONTRIBUTIONS K.M., W.C.H.
and A.L.H. in the design of the study. K.M., G.W.C., B.P.L., W.C.H. and A.L.H. wrote the manuscript. K.M., B.P.L., G.W.C., Y.S., K.S., B.D., M.R., M.B. and A.L.H performed analyses of the
sequencing data. K.M., B.P.L., Y.S., W.J.K., M.D., B.D.K., P.K., Y.Y.T. J.B. and A.L.H. generated the cancer cell lines. J.S., H.C.J., T.L. and K.C.G. oversaw the Aflac Solid Tumor
Biorepository. S.M., C.C. and B.C. oversaw the DF/BCH Solid Tumor Biorepository. W.J.K., M.D., D.A., B.D.K., X.Y., G.C., D.R. and A.L.H. performed and/or analyzed the functional genomic
screens. A.L.C., B.A.L., J.S., H.C.J., C.M., A.J.M., K.C.G., K.L., D.M.W. and P.C.G. generated the PDXs and performed in vivo studies. M.B., B.C., Y.Y.T., J.B., K.L., D.R., A.J.M., D.M.W.,
P.C.G., J.M.S., M.R., E.A.M., K.S., K.C.G., W.C.H. and A.L.H. supervised the studies. All authors discussed the results and implications and edited the manuscript. All authors also read and
approved the final manuscript. CORRESPONDING AUTHORS Correspondence to William C. Hahn or Andrew L. Hong. ETHICS DECLARATIONS COMPETING INTERESTS The authors declare the following competing
financial interests. K.M. is currently employed at PreludeDx. A.L.C. is currently an employee of AstraZeneca. G.C. is currently an employee of Johnson & Johnson. D.M.W. is currently an
employee of Merck and has research support from Daiichi Sankyo, Abcuro, Verastem, Secura, and is on the Advisory Board or has equity in Ajax, Travera, AstraZeneca, Bantam. K.S. receives
grant funding from Novartis and KronosBio, consults for and has stock options in Auron Therapeutics and has served as an advisor for KronosBio and AstraZeneca. D.E.R. receives research
funding from members of the Functional Genomics Consortium (Abbvie, BMS, Jannsen, Merck, Vir), and is a director of Addgene, Inc. W.C.H. is a consultant for ThermoFisher, Solasta Ventures,
MPM Capital, KSQ Therapeutics, Tyra Biosciences, Jubilant Therapeutics, RAPPTA Therapeutics, Function Oncology, Frontier Medicines, Hexagon Biosciences, Serinus Biosciences, Kestral
Therapeutics and Calyx. The remaining authors declare no competing interests. PEER REVIEW PEER REVIEW INFORMATION _Communications Biology_ thanks Harold Lovvorn III, Holger Scholz, and the
other, anonymous, reviewer(s) for their contribution to the peer review of this work. Primary Handling Editor: Manuel Breuer. A peer review file is available. ADDITIONAL INFORMATION
PUBLISHER’S NOTE Springer Nature remains neutral with regard to jurisdictional claims in published maps and institutional affiliations. SUPPLEMENTARY INFORMATION PEER REVIEW FILE
SUPPLEMENTARY INFORMATION DESCRIPTION OF ADDITIONAL SUPPLEMENTARY FILES SUPPLEMENTARY DATA 1-7 SUPPLEMENTARY DATA 8 REPORTING SUMMARY RIGHTS AND PERMISSIONS OPEN ACCESS This article is
licensed under a Creative Commons Attribution 4.0 International License, which permits use, sharing, adaptation, distribution and reproduction in any medium or format, as long as you give
appropriate credit to the original author(s) and the source, provide a link to the Creative Commons licence, and indicate if changes were made. The images or other third party material in
this article are included in the article’s Creative Commons licence, unless indicated otherwise in a credit line to the material. If material is not included in the article’s Creative
Commons licence and your intended use is not permitted by statutory regulation or exceeds the permitted use, you will need to obtain permission directly from the copyright holder. To view a
copy of this licence, visit http://creativecommons.org/licenses/by/4.0/. Reprints and permissions ABOUT THIS ARTICLE CITE THIS ARTICLE Mittal, K., Cooper, G.W., Lee, B.P. _et al._ Targeting
TRIP13 in favorable histology Wilms tumor with nuclear export inhibitors synergizes with doxorubicin. _Commun Biol_ 7, 426 (2024). https://doi.org/10.1038/s42003-024-06140-6 Download
citation * Received: 17 March 2022 * Accepted: 03 April 2024 * Published: 08 April 2024 * DOI: https://doi.org/10.1038/s42003-024-06140-6 SHARE THIS ARTICLE Anyone you share the following
link with will be able to read this content: Get shareable link Sorry, a shareable link is not currently available for this article. Copy to clipboard Provided by the Springer Nature
SharedIt content-sharing initiative