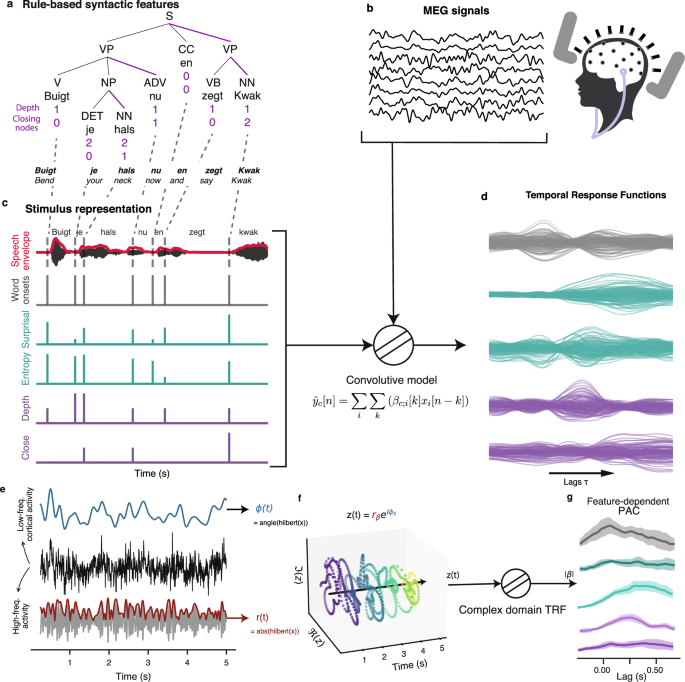
- Select a language for the TTS:
- UK English Female
- UK English Male
- US English Female
- US English Male
- Australian Female
- Australian Male
- Language selected: (auto detect) - EN
Play all audios:
ABSTRACT Humans excel at extracting structurally-determined meaning from speech despite inherent physical variability. This study explores the brain’s ability to predict and understand
spoken language robustly. It investigates the relationship between structural and statistical language knowledge in brain dynamics, focusing on phase and amplitude modulation. Using
syntactic features from constituent hierarchies and surface statistics from a transformer model as predictors of forward encoding models, we reconstructed cross-frequency neural dynamics
from MEG data during audiobook listening. Our findings challenge a strict separation of linguistic structure and statistics in the brain, with both aiding neural signal reconstruction.
Syntactic features have a more temporally spread impact, and both word entropy and the number of closing syntactic constituents are linked to the phase-amplitude coupling of neural dynamics,
implying a role in temporal prediction and cortical oscillation alignment during speech processing. Our results indicate that structured and statistical information jointly shape neural
dynamics during spoken language comprehension and suggest an integration process via a cross-frequency coupling mechanism. SIMILAR CONTENT BEING VIEWED BY OTHERS SPEECH PROSODY ENHANCES THE
NEURAL PROCESSING OF SYNTAX Article Open access 20 June 2024 SEQUENCES OF INTONATION UNITS FORM A ~ 1 HZ RHYTHM Article Open access 28 September 2020 EVIDENCE OF A PREDICTIVE CODING
HIERARCHY IN THE HUMAN BRAIN LISTENING TO SPEECH Article Open access 02 March 2023 INTRODUCTION Humans comprehend language despite high variability in the physicality of speech acoustics,
which can stem from noisy environments or from variations in speakers and their accents. Such robustness in perception may stem from the projection of stored linguistic knowledge via the
anticipatory mechanisms of predictive processing. Predictive processing is a widespread neurocomputational principle, or more generally, a framework in which predictions play an active role
in the processing of upcoming information streams in the brain1. The incoming information may be sensory or solely endogenous neuronal activity from other brain regions, and the output of
such processes may be perceptual, motor, or cognitive2,3,4,5. Although this framework originates from the field of perception6, evidence of such processing during language comprehension is
also abundant7,8,9,10,11,12. Although language comprehension is remarkably adaptable to variation in speech acoustics, the inherent unpredictability of novel messages-each encoding the
intended meaning from the speaker-poses a challenge. This unpredictability is not absolute but relates to the relevance and specificity of each message, which often contains sequences and
structures that are nevertheless anticipated based on knowledge from previous linguistic experience. There are different levels of granularity, each benefiting from prediction from other
representations, such as phonemes predicted from preceding items and spectro-temporal characteristics of the sound segment13,14. Furthermore, an essential feature of human language is its
display of nested syntactic structures over which meanings are computed15,16. Traditionally, language’s inherent unboundedness and generative aspect have often been seen as being in putative
opposition to distributional and statistical accounts of language processing (e.g., the traditional view on the _competence hypothesis_17; or grammar-free account of comprehension18,19). In
contrast to this dichotomy, in the present study, we synthesise these positions and present a framework wherein the syntactic structure and statistical cues are jointly processed during
comprehension20,21,22. We build upon the work of Brennan et al.23 who demonstrated the sensitivity of the BOLD signal to both structure and surprisal but focused on the localisation of such
effect. Moreover, their study, while also investigating naturalistic speech comprehension, could not analyse the temporal dynamics or high frequency activity due to the poor temporal
resolution of the BOLD signal. In the present study, we can leverage the use of magnetoencephalography (MEG) to investigate phase and amplitude dynamics of band-limited cortical activity in
response to variation in both statistical cues and syntactic features. To operationalise syntactic processing, we constructed a set of word-level features which describe critical
characteristics of the underlying constituency parse or tree structure. Those syntactic features are derived from hierarchical trees operating at the sentence level and are de-lexicalised.
They represent aspects of syntactic trees (viz., number of brackets, depth in the tree) which by themselves can elicit a response reflecting tracking of syntactic structures as in Ding et
al. and Frank and Yang24,25. Similar syntactic metrics have been used to study the effect of syntactic operations such as unification (the “merge" operation in the minimalist
program15,26) or integration of an item into a larger structure and the depth, a proxy for ongoing complexity, of the syntactic tree at a given word27,28. These metrics align with
foundational linguistic theories and have been shown to elicit predictable neural responses16,23,29,30. Since those features are derived from the constituency parse, we refer to them as
rule-based features, in contrast to surface statistical features, which are estimated from sequences of words with no information beyond the sequence itself25,31. However, this does not
necessarily imply that such statistics will not carry any information about hierarchical structures or about syntax, a topic that is currently under debate32,33,34. What is indisputable,
however, is that such statistical metrics are built from a sequence of words and optimised to learn a probability distribution conditioned on the sequence context of preceding words. To
learn this distribution, words are presented to a system, such as a recurrent neural network, and the system’s task is to predict the next word based on the series of preceding words
encountered so far (this memory is embedded in the model’s architecture). Non-linear models, like Long Short-Term Memory network or Transformers, can manipulate information such that they
could potentially encode structured information within their memory (hidden states, latent representations). From an information-theoretic perspective, surface statistics can give an
estimate of the self-information (viz., surprisal) and uncertainty (viz., entropy) measured at the word level while conditioning on the observed context of previous words. Importantly we
note that these models, despite their increasingly complex architecture, do not have rule-based knowledge (structurally-)embedded in their learning algorithm and solely make predictions from
an estimate of the conditional probability distribution over the vocabulary that is updated through their inner (recurrent) dynamics. However, we note that recent models, such as larger
GPTx, appear to perform better on a series of syntactic paradigms32,33 compared to older architectures that were trained jointly with grammatical rules and structural information along with
the word sequence (such as RNNG, Grammatical recurrent neural network; but see refs. 28,35,36). Finally, we note that such statistical cues have been shown to modulate cortical activity
during language comprehension27,37,38,39. In light of this apparent dichotomy, and in the context of the debate in cognitive science regarding the role of statistical information in language
processing17,31,40,41, we then ask to what extent do their individual contributions explain neuroimaging data, is the whole better than the sum of its parts? We hypothesise that they
jointly contribute to explaining variance in the MEG data while presenting overlapping spatio-temporal sources. Moreover, the dynamics might disentangle them further as predictions and
statistical inference seem to be a widespread phenomenon in cortical computation, while the organisation of linguistic units into nested hierarchical structures, at least at first blush, may
be related to hierarchical processing in other domains in some ways, but not others42,43,44. We thus further hypothesise that brain responses to statistical and structural features are
operated synchronously, with potential distinct time scales, and orchestrated through cross-frequency coupling. Differentiation arises as information flows from one system of prediction to,
potentially, another, which would compute different combinatorial aspects of structure building and semantic processing. To tap into the orchestration of spatio-temporal dynamics through
cross-frequency coupling, we measured brain activity with magnetoencephalography (MEG), allowing for a time-resolved recording of neural activity. The temporal resolution of MEG data is fine
enough to measure power modulation in a wide range of (high) frequencies together with the phase of a slower frequency range. The role of cortical oscillations in neural computation is
still unclear; nonetheless, an increasing number of studies have now attributed some functional role to different frequency bands, not only for low-level perceptual or sensory processing but
also in relation to speech processing. Delta and theta-band activity (1-4 Hz and 4–8 Hz respectively) play an important role in the neural tracking of the acoustic envelope9,45. It has been
hypothesised that low-frequency cortical activity rhythmically modulates neuronal excitability to match the rhythm and landmarks of the acoustic stream, perhaps reflecting speech
segmentation mechanism46,47,48 but also facilitating the processing of syntactic information via synchronisation to lower level acoustic cues2,16,49,50. Moreover, studies have demonstrated
how low-frequency neural signals couple their phase with power of higher frequency broadband power (phase-amplitude coupling, PAC thereafter) and such coupling has an impact on behavioural
response51,52. It has also been proposed that the ongoing phase of neural signals may be modulated by the predictability of words, thus affecting the temporal prediction of syllable and word
onset48,53. The phase of neural signals is a candidate for carrying information both about statistical and structural features53,54,55,56. However, it is still widely debated whether
measured oscillatory activity in response to speech stimulus truly comes from an oscillatory mechanism or is a by-product of measuring a response to a (pseudo-)rhythmic stimulus, that is, a
series of evoked responses. The interpretation of the role, cause and effect, of low-frequency oscillations remains unclear. While some studies claim that there is evidence that neural
tracking to speech envelope is solely due to evoked response convolved with acoustic edges47,57, other studies find evidence consistent with an entrained endogenous oscillator which shows
sustained activity and phase-related behavioural modulation8,10. We adopt a more agnostic view, focusing on measured phenomena, where we investigate how linguistic features influence neural
activity across different frequency bands, by analysing phase consistency, power modulation and PAC. While we prefer to refer to low- and high-frequency activity specifically, we may use the
term _cortical oscillations_ to refer to band-limited power elevation observed in the MEG power spectra without making any claim about the underlying mechanism. Our hypothesis draws on the
intersection of syntactic processing and predictive coding theories, positing that the brain’s response to language is not just reactive but anticipatory, integrating both structural and
statistical cues in real-time. We thus propose to link properties of syntactic structures, jointly with information-theoretic metrics to MEG data. Brennan & Pylkkänen58 and Nelson et
al.27 have used a similar approach to link syntactic features to electrophysiological data. However, the former studies focused on the localisation of MEG activity to study word-evoked
responses, while the latter analysed high gamma activity recorded from intracranial electrodes. In another study, Brennan & Hale20 used information theoretic metrics built from
context-free grammar parsers and delexicalised n-grams, which do not capture semantic information (thus their surprisal metric greatly differs from ours). Finally, in Brennan et al.
(2016)23, a link is made between hierarchical syntactic features (node count) and surprisal from Markov models (n-grams, lexicalised and unlexicalised). While they elegantly show how
different parsing strategies affect the prediction of neural activity, the statistics of word predictions are overlooked. Modern autoregressive language models produce a more precise
estimate of conditional probabilities, from which we extract surprisal and also entropy, thus allowing for a better account of predictive mechanisms. To our knowledge, the current literature
has not explored the interplay of such features across frequency bands with MEG. Finally, we investigate the relation of word level computation at different timescales, following the work
from Donhauser & Baillet59. Indeed, they observed distinct roles for theta and delta rhythms during the prediction of phoneme sequences. The authors intepreted effects in theta as a
read-out of the sensory sampling mechanism bound to maximize the expected information gain, and their delta effect as encoding non-redundant, i.e. novel, information deviating from
internally generated predictions, which results in an update of the internal model60. Top-down predictions, but also updates, have been already linked to beta power modulation61. All in all,
this mechanism, if supported via delta-phase, is bound to endogenously generated predictions and thus to the internal model of the listener. Thus, in line with Donhauser & Baillet59, we
expect a stronger delta-beta coupling for non-redundant information, which is approximated by the _surprisal_ feature of the statistical features. In the present study, we ask whether
syntactic and statistical word-level features provide complementary cues to the processing of speech input by investigating how putative cortical oscillations and broadband activity are
distinctively modulated by linguistic features. The aim is to discuss how different frequency bands orchestrate the processing of linguistic units. Those units are favoured differently,
whether from predictions on sequential statistics, a potentially domain-general feat, or mirror integration into larger linguistic compounds, thus reflecting language-specialised
computations. Specifically, we examine how syntactic and statistical features influence phase consistency, power, and phase-amplitude coupling. The Results section will detail our findings
on how the brain responds to these linguistic cues, highlighting the separate and combined effects of structural and statistical information on neural processing. Through our analyses, we
aim to shed light on the mechanisms underlying predictive coding in language comprehension, offering insights into the neural basis of language processing. RESULTS In order to measure
time-resolved cross-frequency coupling in relationship to different linguistic features we first analyse both the presence of word-related phase and power modulation as well as how speech
representations perform in predicting MEG signals. First, we extracted linguistic features reflecting both syntactic complexity and statistical properties of speech from the presented
stimuli. So called rule-based features are derived from constituency tree, while statistical features are generated using the probability distribution of next word predictions from a large
language model. These features include syntactic depth and the number of closing brackets to represent structural properties of syntax29,58, and word surprisal and entropy for statistics of
word-level predictions27,38. We construct Temporal Response Function (TRF) models to predict MEG signals based on these linguistic features, enabling a precise examination of how each
feature influences neural responses over time. The analysis pipeline (detailed in the Methods section) involves aligning the MEG data with our linguistic features, then using ridge
regression to estimate the TRF coefficients, and finally evaluating the models’ performance through correlation analysis between predicted and observed neural activities. In most cases,
model comparison is carried out between the true model and a null model for which values (but not timing) of a given feature (or of a feature set) are shuffled. Through this method, we aim
to reveal the mechanisms by which the brain integrates and processes linguistic information at different levels. The general analysis pipeline, along with stimulus representations and
analysis methods, are presented in the diagram of Fig. 1. We measured power spectral density (PSD) of the MEG data in order to assess the quality of the data and the presence of neural
oscillations. As seen in Fig. 2a, b, the MEG data presents neural oscillations in the alpha and beta bands. We found a marginally significant difference in power between the French and Dutch
listening conditions in the beta band (FDR corrected for multiple comparison across all frequencies, corrected _p_-value = 0.06, one sampled _t_-test, dof = 24). We then computed the
cerebro-acoustic coherence by taking the magnitude coherence squared between the sensor-level MEG signals and the sound envelope of the stimuli, see Fig. 2c. This reveals coherent phase
alignment between sound amplitude and MEG signals in the delta and theta bands. Importantly, while those reflect processing of speech-like sounds they do not directly reflect comprehension
as it occurs for both French and Dutch listening conditions. Besides, it is actually significantly greater for the French condition in the delta and theta-band (_p_ = 0.01, cluster-based
permutation test using one sample _t_-test as statistic). Finally, we computed a time-frequency representation for every word-epochs to analyse phase consistency and power modulation at
higher frequencies. We extracted power modulation and inter-word phase clustering from the complex Fourier coefficients. As seen in Fig. 2d, e, we observe power modulation in the beta band,
and inter-word phase clustering in the delta and theta band. PHASE CONSISTENCY AND POWER MODULATION AT WORD ONSETS Recent studies have demonstrated the important role of low-frequency
cortical activity during speech processing. Whether it is a form of neural entrainment to the acoustic envelope or a series of evoked responses to acoustic edges is debated, but results come
together in that they show a stronger correlation between speech spectro-temporal features and MEG or EEG signals in both the delta and theta band7,45,54. Etard & Reichenbach62, for
instance, concluded from their study the existence of a dissociable account for each frequency band with respect to the clarity and intelligibility of the speech signal. Modelling work from
Hyafil et al.48 reinforces the idea of a phase alignment within the theta band to promote segmentation of syllabic sequences. Altogether, we hypothesised change in phase consistency in delta
and theta range after word onset, potentially coupled with modulation of beta oscillatory activity and with gamma broadband activity (which has already been suggested51,52,56). To further
validate this choice of frequency bands of interest, we computed word-triggered phase consistency and power modulation. Fig. 2 presents the long-term power spectral density across all
stories (panel a) as well as power modulation and inter-trial phase consistency happening after word onsets (panels d,e). We found significant power modulation in the beta bands and
significant inter-word phase consistency in the delta and theta bands (cluster-based permutation using one sample _t_-tests as cluster statistics after baseline removal, tested against a
null hypothesis of zero-valued population mean). One challenging aspect in computing the inter-trial phase consistency (ITPC, or inter-event phase clustering and in the case of the present
study: inter-word phase clustering) is that it relies on the clustering of phase, evidently, across trials (defined by word onset timings). Hence, it depends on trial-based experimental
design. We propose an innovative approach to circumvent trial design and leverage the use of naturalistic stimuli by adapting the forward linear model to compute an equivalent of ITPC (and
PAC, shown later on) for continuous M/EEG recordings. We could indeed reproduce those results of word-related modulation by computing a TRF model with word onset only on band limited data
(in Fig. 3a, b). Again a cluster-based permutation test revealed a significant difference against baseline (taken as the average signal for negative lags up to -50 ms). We further
investigated the peak times of the evoked activity contributing to the phase clustering in the low-frequency cortical activity (inset topographies in Fig. 3a). This suggests a
fronto-temporal wave propagating from posterior to anterior sensors in the delta-band and in the opposite direction for the theta-band, such low-frequency travelling waves have been recently
proposed as a mechanism for temporal binding and integration63 as well as for encoding structural information56. These preliminary results indicate the presence of modulation in the
canonical frequency bands, thus validating our choice of frequency bands for further analysis. Note that given the report of theta-gamma coupling in the literature for speech
processing46,48,55, we are adding the gamma band to subsequent analysis. We then proceeded to investigate the influence of linguistic features on the phase and power modulation of the MEG
signal within the following bands: delta (0.5–4 Hz), theta (4–8 Hz), beta (15–25 Hz), gamma (30–80 Hz). We computed the TRF models for each linguistic feature set and frequency band, and
evaluated the reconstruction accuracy of the models. The results are presented in Fig. 3. JOINT CONTRIBUTIONS OF RULE-BASED AND STATISTICAL FEATURES We now assess how brain signals vary in
response to specific aspect of linguistic stimuli. Namely, we built two rule-based features, both derived from syntactic constituency trees: _close_ designates the number of phrasal
constituents a given word is closing (thus counting closing brackets at each word), while _depth_ stands as a proxy of ongoing syntactic complexity simply by measuring how deep in the
hierarchy a given word is. The two other features, _surprisal_ and _entropy_, are derived from a neural language model from which we can compute the probability distribution of next word
prediction based on the preceding context. We first analysed the spatio-temporal dynamics of low-frequency activity in response to all features using a convolutional model which consists in
estimating so-called temporal response functions (TRFs). We assessed the reliability of those features in representing the MEG data by computing the reconstruction accuracy at each sensor
(or sources) for several models (as described in Table 1). As a baseline, we used a model with only acoustic and word onset regressors. Every model is then matched in number of regressors by
swapping the feature of interest with a null feature, which follows the same statistics. Each null model for a given feature set consists of a TRF computed by using a shuffled version of
the stimulus features (the shuffling is strict, as we keep word onset intact and shift values of linguistic features by several words). For instance, to compute the relative increase in
reconstruction accuracy of Rule-based features (as seen in Fig. 3d), we alter the values of the _close_ and _depth_ features while keeping their original timings. By comparing the score to
baseline null models, we normalise for the increasing number of features as each null model contains the exact same number of regressors. As seen in Fig. 3d, each set of features generates a
significant increase in reconstruction accuracy compared to null models. A linear mixed-effects (LMM) model analysis, including subject variability as a random intercept with a random slope
per frequency band, revealed that the feature set had a significant overall effect on the relative score (likelihood ratio test against LMM without feature set factor, _χ_2(17) = 231.65,
_p_ < 0.001) and that frequency band does not significantly influence score outcomes (_χ_2(18) = 15.53, _p_ = 0.63), suggesting that the pattern seen for each model score might be similar
across frequency band. Importantly the full model, which contains both statistical and syntactic features, shows a significantly higher reconstruction accuracy than both other models. We
also compared the reconstruction accuracy of the joint model, comprising all features, and a model where we combined TRF coefficients from the two independently trained “statistical"
and “rule-based" models (called additive model in Fig. 3). The rule-based model presents a marginally higher reconstruction score compared to the statistical-only model in the delta
band (paired _t_-test on means, _p_ < 0.005, correcting for multiple comparison with FDR (Benjamin/Hochberg)). No significant differences were observed in other frequency bands. Across
every frequency bands, the joint model performed better than either specific models (all tests in Fig. 3d are controlled for multiple comparison with FDR (Benjamin/Hochberg) correction on
_p_-values). TIME-RESOLVED CONTRIBUTIONS OF SYNTAX AND STATISTICAL FEATURES We further investigated the time-resolved contribution of each feature set to the overall score. We computed the
score for each feature set for a series of time windows, by evaluating a TRF model _per_ window of analysis. Therefore, there is a distinct TRF model trained for small non-overlapping
segments of 100ms each, spanning from -400–900 ms around word onsets. This is shown in Fig. 3c for each frequency band, where the stacked plots represent the relative increase (in
percentage) of the model of interest over an acoustic model of reference. The base model uses only speech envelope as a feature, while other features are mismatched between stimulus and MEG.
Results show that syntactic features mostly contribute to the score throughout a large time window extending at earlier and later time lags. For both feature sets, we see significant
reconstruction beyond and above acoustic and word-onset only models (Fig. 3c shows uncorrected significant segments with a coloured bar above time regions (paired _t_-tests, dof = 25). We
then adjusted _p_-values of our statistical tests using a conservatory Bonferroni correction, therefore dividing our critical _p_-value by the number of lags and feature set, to control for
potential increase in type I errors. Corrected significant time segments are shown with a marker on the corresponding time-course). For this time-resolved analysis, we used causal filters in
order to avoid any spurious artefacts due to filtering which could alter the score of earlier lags (note that for the rest of the analysis we chose to keep anti-causal FIR linear filter in
order to not alter the phase of signals). Despite this, we observe significant improvement at negative lags in the delta-band. In a supplementary analysis (Supplementary Fig. 2) we compared
the score for each individual feature without grouping them. This showed that _entropy_ and _close_ contribute to the reconstruction accuracy at negative lags. This is not anti-causal as the
entropy at a given word depends only on the previous words heard. In other words, it is possible to observe a neural response time-locked to the current word onset if we assume that the
timing of the coming words is anticipated. For _close_ feature, the same reasoning does not hold, however it can be argued that, in many cases, words closing constituent phrases are also
likely to be more anticipated. This can also be an evidence of anticipatory mechanism for syntactic processing of ongoing structure building. The auditory system have been shown to be
sensitive to long-term duration prior, learned from lifelong exposure over language-dependent syntactic cues64. Finally, the improvement in reconstruction accuracy for syntactic features at
later time lags is likely due to the integration of words into larger syntactic units. This is supported by the fact that TRF time course for closing bracket count feature present the
largest coefficients at later time-lags. This feature corresponds to the number of syntactic units that are closed at a given word, which is a proxy for the integration of words into larger
syntactic units. PHASE AMPLITUDE COUPLING It has been suggested that the phase of low-frequency activity may modulate power of higher-frequency band limited activity or oscillations in order
to align excitability of neural population with relevant segment in speech48,61 or in order to temporally bind and encode structural information56. The question remains whether this
coupling between low- and high-frequency neural activity is also modulated by the predictability of words, thus affecting the temporal prediction of syllable and word onset53. We decided to
investigate the presence of phase-amplitude coupling (PAC) in the MEG data, and in particular to disentangle the effect that distinct linguistic features may have on PAC. First, we examined
the presence of PAC across the entire signal, regardless of the timing of words. That is, we used equation (5) but instead of averaging across trials, we took the average across time. We
computed PAC modulation index for each label in source space following the parcellation from Destrieux et al.65 to limit computational time and memory (74 labels per hemisphere). We found
evidence of PAC between the phase of delta-band activity and the power in the beta and gamma range, as well as between the phase in the theta range and gamma power (Fig. 4). PAC estimates
were compared to surrogate data in order to compute a normalised metrics by z-scoring the resulting modulation index using the mean and standard deviation from the surrogate data
computation. Those standardised metrics were then contrasted with the French condition for statistical testing. We found significant PAC when averaging over labels with the strongest mean
PAC values (paired _t_-tests, dof = 24, realised per phase and amplitude frequency bins, fdr-corrected to control for multiple comparisons). We observed greater PAC over fronto-temporal
network for both delta-beta and theta-gamma PAC (Fig. 4, bottom panels). Furthermore, we are introducing a new method to disentangle the effect of different stimulus features to PAC. As
such, we are investigating PAC relative to word onsets. The simulations described in Fig. 6 show that we can recover the effect of an individual feature affecting phase-amplitude coupling
using a linear forward model based on the complex analytical signal formed with the power of high-frequency activity and the phase of low-frequency activity \({r}_{high}(t){e}^{i{\phi
}_{low}(t)}\). This novel approach relies on a linear forward model akin to temporal response function computation where instead of the band-passed MEG data we used the normalised analytical
signal (see bottom panels of Fig. 1). By doing so, we effectively look at how the phase of the complex analytical signal clusters at a given lag. The advantage of this method is that it
takes into account every predictor we feed into the forward model and thus allows us to establish which features weigh in the most (see details in Methods section, simulations of this
technique are presented in Fig. 6, and further discussion can be found in the supplementary materials). We computed the PAC for each feature set and compared it to a null model where only
the feature of interest is being shuffled. First, the likelihood ratio test comparing LMMs with and without the categorical variables for feature sets and frequency bands indicated a
significant overall effect (_χ_2(7) = 378.63, _p_ < 0.001), suggesting that these factors contribute to variations in the relative score. Pairwise analysis revealed greater score
reconstruction for the joint model for delta-beta while the Rule-based model presents stronger score in theta-gamma (see Fig. 5a and c, paired _t_-tests, dof = 25, _p_-values adjusted with
FDR correction, to compare score increment w.r.t Base model between each model: for the delta-beta PAC: between Statistical and Rule-Based model, _p_ = 7.5_e_−4; between All and Rule-based
model _p_ = 2.7_e_−9; for theta-gamma: _p_ = 0.014 and _p_ = 0.48). To investigate the effect of PAC from a specific feature, we computed null models where only the feature of interest is
being shuffled, one at a time. Then we used a permutation cluster-based analysis to compare the coefficient of the PAC for a given feature and its corresponding null model. Fig. 5 (panels b
and d) show the resulting phase-amplitude coupling coefficients for individual features (supplementary Fig. 3 shows all features, including controls, such as PAC from acoustic envelope). We
summarize the PAC coefficient by computing the global field power (mean squared value) across sensors. A cluster-based permutation test was run against baseline mean values (t-statistics as
test statistic, with primary threshold of _p_ < 0.05, randomly permuting condition labels 1000 times, significance threshold for clusters set at 0.05). All features significantly
modulated cross-frequency coupling in the delta to beta bands. While only precision entropy and closing bracket counts showed a significant modulation of gamma power through theta phase.
Higher PAC coefficients were found in the superior temporal gyri bilaterally. In the left hemisphere, we found clusters in the inferior frontal gyrus, the anterior temporal lobe (for _close_
only) and the superior temporal gyrus. The left inferior frontal gyrus and the left anterior temporal lobe are two regions that have been previously associated with syntactic processing and
semantic composition27,58,66. DISCUSSION A growing number of studies demonstrated how to measure the sensitivity of the brain to naturalistic speech for word-level features. It remains
difficult to control for confounding aspects, such as in Ding et al.24, where it has been argued that the chunking observed around syntactic phrases might be elicited simply by word-level
occurrence statistics or by the repetition of part-of-speech tags25. We built a word-level representation of naturalistic speech encompassing both syntactic, rule-based, features and
statistical, data-driven, features. We showed that both feature sets could be recovered from MEG signals and that they could be used to decode the comprehension state of the subject.
Finally, we showed how phase-amplitude modulation jointly occurs for both feature sets, suggesting that they are both processed and orchestrated in parallel. We found overlapping brain
regions for both feature sets, in particular in the left inferior frontal gyrus, and in the anterior temporal lobe, two brain regions that have been previously associated with syntactic
processing and semantic composition26,27,66. Using naturalistic stimuli, we dissociated, on the one hand feature set computed solely based on statistics of word sequences as computed via GPT
model and, on the other hand a set of rule-based abstract features built directly from constituency tree structures. Temporal response functions obtained from those feature sets could each
explain variance in MEG signals beyond chance and gave enhanced representations of the signal that allowed for decoding of comprehension state on unseen subject data. Nelson et al.27 showed
that broad-band gamma activity was more sensitive to syntactic surprisal, dissociating statistical features from syntax. Although, they trained statistical models purely on sequences of
part-of-speech tags, which conveniently remove the lexico-semantic properties of the context. In the present study, we cannot control for the syntactic information contained in the
statistical features, but we verified that the sensitivity to phrasal boundaries is at least minimal in those features. In particular, the language model is trained to predict the next word
given the previous ones, and thus may learn an approximation of syntactic distributions across words as much as that approximation facilitates next word prediction. Therefore, the
statistical features are not fully independent of syntax. However, syntactic features are computed from annotations of the constituency-parse tree structure of the sentences, and thus do not
contain any information about the statistics of word sequences. And we assume that they are solely driven by the syntactic structure of the sentences. The result of the joint model
explaining over and beyond any of the individual feature sets suggests that the brain is sensitive to both aspects of language in an inseparable manner. This synergy is observed in regions
where both types of information are encoded with equal strength. We found that both feature sets consistently drive an increase relative to our base model (envelope and word onset) across
frequency bands (see Fig. 3). Only in the delta band we observed a significant improvement of Rule-based feature over Statistical ones. Syntactic processing has been previously shown to
elicit a stronger phase-locked neural activity within the delta-band in response to speech24,49,67,68. However, as both feature sets contribute to a significant increase, we infer that
statistical information aids the inference of categorical information. The idea that both prediction errors and representational states, which in the present study may be related to
statistical and syntactic features respectively, are processed hierarchically and in parallel at different levels has been recently the converging result across a number of studies using
naturalistic stimuli for speech11,14,69 This effect can be observed at other levels of granularity during speech processing, for instance, in the processing of the spectro-temporal acoustic
features into categorical phonemes, and from phonemes themselves into lexical representations - in this sense, integration from phonemes to words, is modulated by statistical cues from
top-down predictions5,13,59. In particular, our findings relate to Giraud & Arnal5 who showed that slow cortical activity is related to the processing of the predictability of the next
word in a sentence. Our results extend this finding by showing that low-frequency phase information modulates gamma power in a way that is related to the predictability of the next word in a
sentence, namely when the uncertainty of those predictions is high. Different aspects of syntax have been previously linked to brain activity ranging from syntactic violation and the P600
event-related potential component70 (for a review, see Kuperberg, 200771), to phrase structure tagging24 and syntactic surprisal27. In particular, the _merge_ operation26,27,72 has the
privilege of reflecting a crucial aspect of syntax, namely its recursive and combinatorial power. The merge operation is fundamental for composition and syntactic unification. It is also
crucial in endowing language with its recursivity, allowing it to generate an unbounded set of utterances from a finite vocabulary set. Researchers have focused on pinpointing a specific
location for such computation26. However, it is unclear whether such computation, in the brain and in complementary fashion to computational-level claims in formal theories, occurs in
isolation from semantic processing and more generally, from the dynamical update and integration of cues at different levels of the speech and language processing hierarchy21. We used the
closing bracket count as a proxy for the binding operation, similar to other studies23,27. We found that spatio-temporal responses to both syntactic features are generally left-lateralised,
but not exclusively so; moreover, we found distributed activity in the left anterior temporal lobe and in parietal regions and inferior frontal gyrus. In the time domain, we found a
significant effect of closing nodes in both delta and theta bands. Other studies have attributed neural tracking to speech in the theta-band to the active chunking process of word units73,
perhaps aided by predictions5,59. In that respect, our data suggest that syntactic processing may also play a role in aligning with linguistic units and in providing a basis for tracking of
such linguistic units. Syntactic and predictive processing do not need to be fully disjoint in the brain. Each feature can jointly contribute to the prediction of upcoming input while at the
same time help in integrating context with currently processed units. Locally, in a given neural circuit, we propose that binding of neural representations can be made through
phase-amplitude coupling. This mechanism has been proposed to be a general mechanism for neural binding74,75, and has been argued to be involved in the processing of linguistic
features5,56,59,61,67. In particular, we found that the coupling between theta phase and gamma amplitude was stronger, specifically for entropy and for the integration of nested trees. This
may suggest that the two feature sets are processed in parallel. These features reflect how neural dynamics anticipate precision-weighted predictions and integrate a word to the current
phrase respectively. We see evidence of phase alignment and power modulation from the word-evoked activity by measuring phase constituency and induced power across all canonical frequency
band of M/EEG. Importantly, the phase constituency may reflect mere evoked and time-locked activity, and not only oscillatory mechanisms. Therefore, we do not take those results as evidence
for the presence of oscillatory response for low-frequency signals. Beta band power might, however, be reflecting oscillatory power change. Interestingly we see a typical decrease in beta
power at the word-level. But once we disentangle the effect of specific features, we find for instance, a positive effect of syntactic depth, a broad proxy for syntactic complexity, which is
in line with the structure and sentence-building effect leading to increased beta power observed72,76,77. More recently, an analysis on the dependency parse of sentences on this very same
dataset also provided with evidence for the role of beta for maintenance and/or prediction during dependency resolution. Zioga et al.30 showed that the beta power was modulated
proportionally with the number of dependencies to be resolved. This would result in a consistent power increase while complex sentences are being processed. We also find a positive effect of
depth in the delta band, which is in line with the syntactic processing account of delta tracking16,24,49,54,67,68. Our results support the dual timescale for predictive speech processing
as proposed by Donhauser & Baillet59. The observed theta/gamma coupling occurs for highly expected information gain (high entropy leading to stronger coupling) and for words which must
integrate into a larger number of constituents. This is in line with the idea that theta is a sensory sampling mechanism tuned to maximize expected information gain59. A word that closes
several nested constituents will generally be well predicted syntactically (for its part-of-speech tag is constrained by context) but highly anticipated semantically (anticipated, as in
expecting high-information content although not actually known or predicted), as it plays a crucial role in the sentence (and even more possibly in word-final languages). As such, an
externally driven, weakly entrained, oscillator could synchronize the integration of a word into a larger syntactic structure while aligning through phase synchrony and nested theta-gamma
oscillations the excitability of neural assemblies engaged (as in48 though at word level here). This is synchronized both with the input and the internally generated temporal predictions
further as the expected information gain is high. The computational model proposed by Ten Oever & Martin53 also supports such framework where the predictability of a word will generate a
corresponding phase advance or lag. Although in our study, it is precisely the expected information gain that is driving the phase synchrony. On the other hand, delta/beta coupling occurs
for words that are less predictable, i.e., with a high surprisal value. This is in line with the idea that delta is encoding non-redundant information as in Donhauser & Baillet59. In
other words, novel information deviating from internally generated predictions generates an update of the internal model. Top-down predictions, but also updates, have been already linked to
beta power modulation61,76. All in all, this mechanism, if supported via delta-phase, is bound to endogenously generated predictions and thus to the internal model of the listener21. Given
the synchronous increase in coupling for syntactic features (depth and close) we suggest that this internal model update is aligned with the slower rhythm of (predicted) phrasal
boundaries60. We find these results to align with the idea of multiplexed and alternating predictive and integrative mechanisms between, respectively, top-down and bottom-up information as
seen in Fontolan et al.78 or in the syllabic-level speech inference model from Hovsepyan et al.61. If based purely on data showing tracking to sound envelope, we must note that delta band
does not reflect purely linguistic processes. First we see on the present data that coherence with sound envelope is stronger in delta for uncomprehended language, and that power alone does
not carry sensible difference between our listening condition (Fig. 2a, b). Moreover, low-frequency tracking of envelope is sensitive to acoustic changes too, rather than linguistic
information, for instance it becomes larger when pauses are inserted in the stimulus79. Here however, we note the role of delta phase and its coupling with higher frequency band, in
particular beta, for the processing of linguistic features. We observed strong dependency of beta modulation, coupled through the phase of delta due to word surprisal. This is in line with
the idea that beta carries out top-down predictions56,61 and internal model updates while being modulated by the phase of endogenously generated delta rhythms30,77,80. Given the importance
of theta- and delta-band speech tracking for comprehension38,45,62,81, we propose that the coupling between delta and higher frequency bands is a general mechanism for the processing of
linguistic features, possibly leveraging the temporal regularities of lower-level cues to align with internally generated predictions and representations. Previous studies have highlighted
the role of beta oscillations for predictive coding61,82. We use surprisal as a proxy for information prediction, which may or may not take some syntactic and semantic features into account.
It certainly does not build upon lower levels such as spectro-temporal acoustic information, as this would imply having trained a statistical language model from raw audio data. In the
classical view of predictive processing though, bottom up prediction errors are matched against top-down predictions. One caveat in language is that, beyond the sensory representations, the
hierarchy of representation is not well-defined in the brain. Structural and semantic information may not need to always sit within a strict hierarchy (except for example when function
application or domain specification for semantic functions is specified by syntactic structure - which may be quite often), and the brain may nonetheless leverage both sources of information
to work in parallel. Thus, it is unclear what becomes top-down or bottom-up information at the sentence level, when considering only word-level features. Moreover, we must account for the
temporality of speech, where contextual information, from previous words, also helps to refine predictions. In this study, surprisal and precision (entropy) encompass such information from
the word sequence, while syntactic features are more likely stemming from higher levels sources (i.e., not purely lexically-driven) if we consider the possibility that sentence level
representations serve as top-down predictions for upcoming word-level processing. Indeed, the information added by incoming words within nested syntactic structures comes about by taking in
account the embedding into a larger constituent, and thus reflects linguistic knowledge and processing over the entire sentence, if not discourse. As such, on a predictive account, the
information from lower levels, such as phoneme sequence and fine-grained acoustic information, together with their relation to word recognition, must also be accounted for to fully capture
the predictive context of speech processing. A comprehensive account of a predictive coding theory of speech processing must therefore incorporate an ongoing model of language processing
together with perceptual processing of incoming sensory input. In conclusion, we investigated the contribution of rule-based syntactic features together with the sequential predictability of
words to cortical signals. Each set of features resulted together in a more accurate representation of MEG signals, suggesting an overlap in how the brain encodes and processes those
different features. By computing a forward model, we could extract the neural response to those features from naturalistic listening conditions, thus leveraging the need to manipulate the
stimulus to exhibit responses to phrase structures. Syntactic operations, such as _merge_, operationalised here with the closing bracket count, together with the depth in constituency tree
structures, showed a temporally broader response around word onset as compared to the sequence-derived features of surprisal and entropy. Across several frequency bands we observed distinct
networks with some overlapping regions were both feature sets improved reconstruction accuracies. In particular, we found that the statistical features were more sensitive to theta band
activity, while the syntactic features were more sensitive to delta band activity. Phase-amplitude coupling analysis revealed that the two feature sets are processed in parallel, and are
used to predict the next segment (lexicalised items in the current study) as it is integrated with the current context. We found that the coupling between theta phase and gamma amplitude was
stronger, specifically for entropy and for the integration of nested trees. This suggests that the two feature sets are jointly used to update predictions and integrate the linguistic
content to the current phrase. Given the role of theta band in speech tracking59,62,73 we suggest that such rhythms are tightly bound to the acoustic signal and reflect thus temporal
predictions aligned with high expected information53. More generally, all features presented significant cross-frequency coupling between delta and higher frequency bands. But we observed a
larger delta-beta coupling for surprisal, potentially in line with the idea that beta carries out top-down predictions and internal model updates while being modulated by the phase of
endogenously generated delta rhythms. Given the importance of theta- and delta-band speech tracking for comprehension38,45,62,81, we propose that the coupling between delta and higher
frequency bands forms a core cortical computational mechanism for the processing of linguistic features, as speech becomes language, and leverages the temporal regularities of lower-level
cues to align with internally generated predictions and representations. METHODS The study was approved by the ethical commission for human research in Arnhem and Nijmegen (CMO2014/288). A
total of 25 participants (18 women, between 18 and 58 years old) completed the experiment. Informed consent was obtained from all individual participants. All participants were right-handed
native Dutch speakers with no reported fluency in French despite incidental exposure. Participants self-reported their (in)ability to understand a sentence in French. Participants were given
monetary reimbursement for their participation. EXPERIMENTAL DESIGN Participants were asked to listen and pay attention to several audio stories while we simultaneously recorded their MEG.
The stimulus consisted of Dutch short stories (from Hans Christian Andersen and the Brothers Grimm) and French stories (from Grimm, E. A. Poe and Hans C. Andersen) available online on the
Gutenberg project, totalling 49 min and 21 min, respectively. All stories were divided into short story parts lasting between 5 and 7 min (leaving a total of 9 Dutch story parts and 4 French
ones). Each story part was presented without interruption, while participants fixed a cross in the centre of the presentation screen. Participants were prompted on-screen with five
multiple-choice questions between each part to assess their attention and comprehension. Stimuli were presented using the Psychtoolbox library on Matlab83. MEG data were acquired at 1200 Hz
using a CTF 275-channel whole-head system (VSM MedTech, Coquitlam, Canada) in a magnetically shielded room. The MEG system was equipped with 275 first-order axial gradiometers with a
baseline of 5 cm. The head position was measured before and after the experiment using five head position indicators (HPI) coils. The HPI coils were activated every 200 ms during the
experiment to monitor head movements. The head position was corrected for each story part using custom-built Matlab code to display how the head aligns with the initial recorded position;
participants were asked to adjust their head position to fit head markers if the movement was too large. Participants could self-pace the start of a trial after answering the behavioural
comprehension question, allowing them to pause between stories blocks. Each participant also had a structural MRI scan (T1-weighted) using a 3T MAGNETOM Skyra scanner (Siemens Healthcare,
Erlangen, Germany). The MRI scan was used to reconstruct the cortical surface of each participant using FreeSurfer software (Martinos Center for Biomedical Imaging, Charlestown, MA, USA).
The cortical surface was used to project the MEG sensor data onto the cortical surface using a linearly constrained minimum variance beamformer84. Finally, we measured the head shape of each
participant using a Polhemus Isotrak system (Polhemus Inc., Colchester, VT, USA) to co-register the MEG and MRI data. MEG PREPROCESSING The original MEG data were recorded at 1200 Hz. We
first resampled the data at 200 Hz after applying and anti-aliasing low-pass filter to the data. Noisy channels and flat channels were marked as bad for interpolation and to be discarded in
subsequent analysis (computation of covariance or ICA algorithm). We removed blink artefacts by matching ICA component time courses to measured EOG and similarly removed heartbeat artefacts.
Note that the ICA decomposition was run on data filtered between 1 and 40 Hz, we then kept the ICA spatial filters for subsequent processing while discarding the band-passed data used to
compute them. Finally, we applied a notch filter at 50 Hz to remove line noise. SOURCE RECONSTRUCTION We then used all data to compute a data covariance matrix, and used it to compute a
noise-normalized linearly constrained minimum variance (LCMV) beamformer84. The LCMV beamformer was created using a 7 mm grid with 3 mm spacing, using functions from the MNE-python
library85. TIME-FREQUENCY ANALYSIS was performed on the MEG data using the MNE software package85. We first epoched the data around word onset, with no baseline applied. We then used the
Morlet wavelet transform to compute the time-frequency decomposition of the MEG data. The Morlet wavelet transform was computed with varying number of cycles from 2 to 7 cycles. We used a
logarithmically spaced grid of frequencies between 3 and 80 Hz, with 32 steps. The time-frequency decomposition was computed for each epoch. We extracted the average power by taking the
absolute of the complex Fourier coefficients and the inter-words phase constituency by summing the complex Fourier coefficients after normalising them by their absolute value. STIMULUS
REPRESENTATION Most of the analyses performed relied on linear models. We computed forward encoding models which map stimulus features to MEG data. Such models are also called temporal
response functions (TRF)86. This approach gives us a way to assess the importance of each feature in the stimulus in explaining the MEG data. The obtained model coefficients are also
directly interpretable in terms of modulated neural activity, as opposed to filters learned from backward models87. In this section, we will first present how each stimulus features were
defined and computed, and then how the TRFs were estimated from the data. In light of the current literature, we focused on rule-based syntactic features on the one hand, following cortical
tracking of hierarchies suggested by Ding et al.24, and on statistical features reflecting predictive processing of sequences38. The syntactic features were computed using the Stanford
parser88. The statistical features were computed using the GPT2 language model89. Importantly, we do not assume that language processing is supported by information-theoretic metrics in the
hard sense. That is, we are not proposing a theory of language understanding from mere surface statistics. However, following the hypothesis given by theories on predictive processing in
various cognitive domains and notably for perception, we assume that the brain can extract information-theoretic features from the stimulus, regardless of the specifics of its
representational format (e.g., functionally semantic or syntactic), and that these features are actively used to predict internal representations. Those information-theoretic features are
thus a proxy for the underlying domain-general cognitive process of predictive inference, notably for predictive processing and Bayesian inference, where surprisal becomes a proxy for
prediction error59,90 and entropy for uncertainty3. Both of these are critical concepts in predictive processing. These quantities are involved in most predictive mechanisms4,90, where cues
are extracted at different representational levels to predict or infer upcoming linguistic information. On the other hand, rule-based features are derived from specific instantiations of
parsing mechanisms (such as constituency trees in context-free grammar), which already support a particular theory of syntax derived from linguistics rather than neuroscience. Again, we are
not suggesting by the use of those particular features that the brain is precisely implementing such a parsing strategy to compute syntactic representations. Nevertheless, the known
sensitivity to such structures24 motivates the use of a metric that captures the general shape of syntactic trees throughout naturalistic sentences. We decided to create a set of features
that tracks the complexity of such structures and also captures the integrative mechanisms at play when words or phrases need to be integrated into a larger syntactic unit. We note that
although the left-corner parsing strategy has been previously suggested as a better model for cognitive processing of syntax22,58,91, this was done on English data and potentially differs in
head-final or mixed word order languages16,92. Finally, it may be that for the method applied here, the fine-tuning of parsing strategy does not matter as much as the mere presence of
brackets, indicating constituent structures16. * Rule-based (or Syntactic) features: To build our syntactic features, we first ran a tree parser on every sentence from our stimuli. * 1.
Depth: Syntactic depth is a proxy for syntactic complexity. A word highly embedded within nested structures, which is to say, a word deep in the tree structure hierarchy, will carry a higher
value for this feature. This may reflect several cognitive processes among which the maintenance in working memory of syntactic structure or the general cognitive load for processing
complex sentences. * 2. Close: This refers to the number of subtrees being closed at a given word. Some words do not close any subtree, and some will close several at once. This feature
encompasses the variability accounting for integrative mechanisms such as “merge"26. When words or phrases need to be grouped into a larger syntactic unit this feature is incremented.
It is also referred to as _bottom-up_ count of syntactic structures16,23,92. This is in contrast to the top-down count, which enumerates opening nodes. Giglio et al.92 found bottom-up
parsing to better represent structure-building during comprehension rather than production, which we decided to use here. * Statistical features: Extracting the information-theoretic values
requires an estimate of the probability distribution of each word in the utterance conditioned on the previous word (over the entire vocabulary). This was quantified using GPT2, a
state-of-the-art language model, which is trained specifically to causally predict word from sequences of textual data. * 1. Surprisal: The negative natural logarithm of the probability of a
given word item conditioned on the sequence of preceding words; \(-\log (P({w}_{i}| {w}_{i-1},{w}_{i-2},\ldots,{w}_{1}))\). * 2. Entropy: It quantifies the amount of uncertainty, at a given
word, in predicting the next word. Mathematically, this is the expected surprisal, which we can also interpret as the expected information gain. It is computed as \(-{\sum
}_{{{{\rm{words}}}}}P({w}_{i}| {w}_{i-1},\cdots )\log (P({w}_{i}| {w}_{i-1},\cdots ))\) On top of those features of interest, we used two other regressors to control for the low-level
acoustic and prosodic effects on neural signal as well as any unexplained variance at the word-level. This was achieved by using the acoustic envelope along with a “word onset"
features. The former was computed from the audio waveform by half-rectifying it and then applying a low-pass filter (type I FIR filter, _f__c_ = 20 Hz, transition bandwidth of 10 Hz,
attenuation in stop band of 60dB, ripples relative to peak = 1_e_−3) and raising the signal to the power of \(\frac{1}{3}\) to mimic the non-linear compression from early auditory processing
stages. The latter comprised of a comb-like feature with constant values of “ones" aligned with every word onsets. A table of the features used in the analysis is presented in Table 1.
The correlation is not excessively high and should not, presumably, lead to collinearity issues in the subsequent analysis. To verify we computed the Variance Inflation Factor (VIF) for
each feature. All VIFs were below 2, which is below the threshold of 5, indicating that there is no collinearity issue in our data93. TEMPORAL RESPONSE FUNCTIONS With the speech
representation time-aligned to the magneto-encephalographic recordings, we then compute optimal filters that map the stimulus to the MEG signals. This is known as forward modelling, or
encoding, and in particular the present method boils down to extracting _temporal response functions_ of the above features. This method assumes a convolutional linear model mapping from
stimulus to MEG data. Such an approach has been successfully applied to recovering brain responses to sound envelope7,62, allowing to further decode auditory attention, and also in
estimating brain responses to ongoing linguistic features38,94. This has been applied with different neuroimaging data such as fMRI, ECoG, and M/EEG. We model the MEG response signal at
sensor _i_ (or source estimate location) \({\{{y}_{i}\}}_{t}\) as a convolution between a kernel _β_ (to be estimated) and the stimulus representation signal \({\{{x}_{j}\}}_{t}\) for the
_j_th feature. Activity from the data that is not captured by this model is supposed to be Gaussian noise. $${y}_{i}({t}_{n})={\sum}_{j=1}^{{N}_{feat}}({\beta
}_{ij}*{x}_{j})({t}_{n})={\sum}_{j=1}^{{N}_{feat}}{\sum}_{\tau=1}^{{\tau }_{\max }}{\beta }_{ij}(\tau ){x}_{j}({t}_{n}-\tau )+{\epsilon }_{in}$$ (1)
$$\hat{{y}_{i}}({t}_{n})={\sum}_{j=1}^{{N}_{feat}}{\sum}_{\tau=1}^{{\tau }_{\max }}\hat{{\beta }_{ij}}(\tau ){x}_{j}({t}_{n}-\tau )$$ (2) Note that the hat symbol, \(\hat{.}\), correspond to
the estimated or reconstructed values. It is easy to rewrite equation (2) in a vectorized form as \(\hat{{{{\bf{Y}}}}}=\hat{{{{\mathbf{\beta }}}}}{{{\bf{X}}}}\) where the temporal dimension
is expressed as column vectors and each channel data (vector) are concatenated along the row dimension of the matrix \(\hat{{{{\bf{Y}}}}}\). In this formulation, X, called the design matrix
in the context of linear models, contains all the lagged time series of every feature in its columns and time samples along its rows: \({{{\bf{X}}}}\in {{\mathbb{R}}}^{({N}_{feat}\cdot
{\tau }_{max})\times {N}_{samples}}\). The matrix \(\hat{{{{\bf{Y}}}}}\) contains the reconstructed MEG data, with the same number of rows as X and as many columns as MEG sensors or source
estimates: \(\hat{{{{\bf{Y}}}}}\in {{\mathbb{R}}}^{{N}_{samples}\times {N}_{sensors}}\). Finally, \(\hat{{{{\mathbf{\beta }}}}}\) is the matrix of estimated TRFs, with a row for each lag for
each feature and as many columns as MEG sensors or sources: \(\hat{{{{\boldsymbol{\beta }}}}}\in {{\mathbb{R}}}^{({N}_{feat}\cdot {\tau }_{\max })\times {N}_{sensors}}\). Written in its
vectorized form, we can easily see how the closed-form formula to estimate \(\hat{\beta }\) arises: $${{{\bf{Y}}}}={{{\bf{X}}}}{{{\boldsymbol{\beta }}}}\\
{{{{\bf{X}}}}}^{{{{\bf{T}}}}}{{{\bf{Y}}}}={{{{\bf{X}}}}}^{{{{\bf{T}}}}}{{{\bf{X}}}}{{{\boldsymbol{\beta }}}}\\ \hat{{{{\mathbf{\beta
}}}}}={({{{{\bf{X}}}}}^{{{{\bf{T}}}}}{{{\bf{X}}}})}^{-1}{{{{\bf{X}}}}}^{{{{\bf{T}}}}}{{{\bf{Y}}}}$$ (3) However, when the inversion of XTX is unstable, which easily happens with continuous
predictors, a regularised autocorrelation matrix is used instead (via Thikonov regularisation): $${{{\bf{w}}}}={({{{{\bf{X}}}}}^{T}{{{\bf{X}}}}+\lambda
{{{\bf{I}}}})}^{-1}{{{{\bf{X}}}}}^{T}{{{\bf{y}}}}$$ (4) where _λ_ is the regularisation parameter, and I is the identity matrix. Wherever used, the regularisation parameter was set to _λ_ =
< _λ__k_ > _k_, the arithmetic mean over the eigenvalues of XTX. All the TRF estimation and scoring was carried out using custom-built Python code. This allowed to optimise the
computation of the TRF: in particular, we aggregate the computation of correlation matrices across stories and leverage on the use of singular value decomposition to compute the
pseudo-inverse of the design matrix. MODEL EVALUATION We used Pearson’s correlation coefficient to assess the quality of the model fit. We computed the correlation between the predicted and
the observed MEG data for each model and each sensor or source location. We then averaged the correlation across sensors to obtain a single value for each feature set. In order to avoid
overfitting, we used a leave-one-story-out cross-validation scheme. We trained the model on all but one story and then tested the model on the left-out story. We repeated this procedure for
each story and then averaged the correlation across stories. Moreover, we used mismatched feature values to compute a null distribution of scores and coefficients (see Table 1 for a
description of each model used). We then compared the observed correlation to the null distribution to assess the significance of the model fit of a given feature set. Those mismatched
features were generated by keeping the same onset timings but shuffling the feature values across words. By doing so, we are keeping the same temporal structure and statistics within the
feature set but destroying any potential relationship between the feature and the MEG data. As an example, when we compute the score of a given feature set, e.g. Statistical features, we
compare the observed score to the distribution of scores obtained by fitting the model with mismatched statistical features, while keeping other features intact. This method also has the
advantage to control for the number of features in the model thus correcting the bias in the score, in particular, due to extra features when those are not explaining variance at a given
sensor. INTER-WORD PHASE COHERENCE AND PHASE-AMPLITUDE COUPLING GLOBAL PAC A first analysis for estimating phase amplitude coupling consisted in scanning across several frequencies using a
time-frequency representation of the MEG data. We used the Morlet wavelet transform to compute the time-frequency decomposition of the MEG data. The Morlet wavelet transform was computed
using seven cycles between 1 and 80 Hz. We extracted the average power by taking the absolute of the complex Fourier coefficients and the phase by normalising the complex wavelet output by
its absolute value. We used the python package Tensorpac (version 0.6.5)95 to extract this global standardised PAC coefficient. The library’s PAC computation also dealt with the shuffling of
amplitude segments to compute the surrogate data. TRF-BASED PAC One challenging aspect in computing the inter-trial phase consistency (ITPC, or inter-event phase clustering and in the case
of the present study: inter-word phase clustering) is that it relies on the clustering of phase, evidently, across trials (defined by word onset timings). Hence, it depends on trial-based
experimental design. We propose an innovative approach to circumvent trial design and leverage the use of naturalistic stimuli by adapting the forward linear model to compute an equivalent
of ITPC and PAC for continuous M/EEG recordings. Our approach consists in using the complex analytical signal to compute a complex-valued TRF which will jointly contain phase and amplitude
information. Computing the TRF is equivalent to finding the kernel of the convolution, which triggers the recorded response and thus captures and summarises the phase concentration from a
continuous recording. In essence, the TRF computed from a comb-like time-series as features is analogous to ERP analysis. We can extend this analogy and consider this method as a way to
compute ITPC and PAC from continuous recordings. The classic, trial-based, ITPC is computed as: $$ITP{C}_{i}(t)=\frac{1}{{N}_{trials}}\left| {\sum}_{trials}{e}^{{\phi }_{i;\delta
}(t)}\right|$$ While the PAC can be computed as74: $$PA{C}_{\delta \to \beta }(t)=\frac{1}{{N}_{trials}}\left| {\sum}_{trials}{r}_{\beta }(t){e}^{{\phi }_{i;\delta }(t)}\right|$$ (5)
Therefore, we can use the continuous signals \({e}^{{\phi }_{i;\delta }(t)}\) and \({r}_{\beta }(t){e}^{{\phi }_{i;\delta }(t)}\) to compute ITPC and PAC respectively, with respect to
different features. Using only word onset (a feature that is one at word onset and zero otherwise) is equivalent to the trial-based computation of those quantities. However, this extended
framework allows us to incorporate other exogenous variables and thus analyse their respective contributions to ITPC and PAC. Forte et al.96 have successfully applied such a modelling
approach on complex signals to recover phase and amplitude response in brainstem recordings. In order to investigate whether this method reliably recovers features that specifically
contribute to some phase-amplitude coupling, we simulated the effect and recovered the dynamics of coupling from features, beyond the event-related mean vector length. Those simulations are
presented in Fig. 6. We simulated three different scenarios: (A) a simple phase-amplitude coupling where the phase of low-frequency signal modulates the amplitude of a faster signal
regardless to event timing. Then we simulated phase-amplitude coupling occurring in a time-locked manner after some events. Finally, a third simulation was performed where phase-amplitude
coupling occurs in a time-locked manner and the amplitude is modulated differently depending on the values of an external feature signal. We then computed the TRF for each of those
simulations and compared them to the mean vector length computed across trials. As seen in Fig. 6, we can recover the effect of an individual feature affecting phase-amplitude coupling from
the linear forward model. STATISTICS Power spectral densities, coherence, ITPC and power modulation comparisons (in Fig. 2) were carried out using cluster-based permutation tests97
implemented in MNE Python85. We used 1024 permutations and a threshold of _p_ < 0.05 to determine significant clusters. Reconstruction scores and kernel coefficients learned through
linear regression (TRFs) were tested for significance against a null model of temporal response functions which contains control regressor nonetheless (Base model). We constructed an
empirical estimate of the null distribution represented by a model where syntactic and predictive features were unrelated to the stimulus. This is realised by shifting the values of each
feature while keeping the word-onset timings intact. Therefore, each reconstruction score can be compared to the corresponding null model which matches the number of features. To assess the
overall effect of model structure (Rule-based, Statistsical or Joint) and frequency band, we ran a linear mixed model (LMM) analysis with the relative score difference with respect to the
Base model as a dependent variable and a random intercept per subject (and random slope per frequency band where applicable). This was done using statsmodels library (version 0.14.1) with
the formula: score ~ feats + fband + (1 + fband ∣ subject). We then determine the significance of fixed-effects with a likelihood ratio test with a nested model which only account for
intercepts. Finally, post-hoc analysis on the relative score differences is done using one sampled _t_-tests, comparing their value against zero. The resulting _p_-values were corrected for
multiple comparisons using the Benjamini-Hochberg, controlling for the false discovery rate (FDR), implemented by MNE Python’s fdr_correction method85. Global PAC in Fig. 4 are computed by
standardising the computed modulation index with one obtained from surrogate data (by shuffling amplitude segments). We then compared thos standardised coefficient between Dutch and French
condition using paired _t_-tests, correcting for multiple comparisons with FDR correction after averaging across labels with the strongest PAC within each frequency band. For TRF
time-courses (PAC coefficients, in Fig. 5b, d bottom row), we used a cluster-based permutation tests to assess significance (against baseline mean value) and extract spatio-temporal
clusters97 using MNE python’s _permutation_cluster_1samp_test_ with 1024 permutations and using the default threshold value except when indicated in the main text. In the case of the
comparison of reconstruction scores in the source space (Fig. 3e), we used a paired _t_-test to compare the scores across feature sets in a pairwise manner. The resulting _p_-values were
corrected for multiple comparisons with FDR correction and used to display the significance of the comparison in source space. REPORTING SUMMARY Further information on research design is
available in the Nature Portfolio Reporting Summary linked to this article. DATA AVAILABILITY The raw MEG data generated in this study have been deposited in the Radboud University
Repository database and are openly available with the identifier https://doi.org/10.34973/a65x-p00998. Processed MEG data and Source data underlying the figures in this paper are available
in Figshare with the identifier https://doi.org/10.6084/m9.figshare.24236512. CODE AVAILABILITY The code supporting the findings of this study is available on the GitHub repository at
https://github.com/Hugo-W/feature-PAC99. All the analysis was carried out using Python 3.12 using custom code, heavily based on usage of the MNE-Python library (version 1.6.1)85. Figures are
done using Matplotlib (version 3.8.3) and Seaborn (version 0.13.2). REFERENCES * Keller, G. B. & Mrsic-Flogel, T. D. Predictive processing: a canonical cortical computation. _Neuron_
100, 424–435 (2018). Article PubMed PubMed Central CAS Google Scholar * Bastiaansen, M. & Hagoort, P. Oscillatory neuronal dynamics during language comprehension. _Prog. Brain Res._
159, 179–196, 0079-6123. (2006). Google Scholar * Friston, K. The free-energy principle: a unified brain theory? _Nat. Rev. Neurosci._ 11, 127–138 (2010). * Arnal, L. H. & Giraud,
A.-L. Cortical oscillations and sensory predictions. _Trends Cogn. Sci._ 16, 390–398, 13646613 (2012). Article Google Scholar * Giraud, A.-L. & Arnal, L. H. Hierarchical predictive
information is channeled by asymmetric oscillatory activity. _Neuron_ 100, 1022–1024 (2018). Article PubMed Google Scholar * Rao, R. P. N. & Ballard, D. H. Predictive coding in the
visual cortex: a functional interpretation of some extra-classical receptive-field effects. _Nat. Neurosci._ 2, 79–87 (1999). Article PubMed Google Scholar * Keitel, A., Ince, R. A. A.,
Gross, J. & Kayser, C. Auditory cortical delta-entrainment interacts with oscillatory power in multiple fronto-parietal networks. _NeuroImage_ 147, 32–42 (2016). PubMed Google Scholar
* Zoefel, B., ten Oever, S. & Sack, A. T. The involvement of endogenous neural oscillations in the processing of rhythmic input: More than a regular repetition of evoked neural
responses. _Front. Neurosci._ 12, 1–13 (2018). Article Google Scholar * Di Liberto, G. M. et al. Low-frequency cortical entrainment to speech reflects phoneme-level processing. _Curr.
Biol._ 25, 2457–2465 (2015). Article PubMed Google Scholar * Doelling, K. B., Assaneo, M. F., Bevilacqua, D., Pesaran, B. & Poeppel, D. An oscillator model better predicts cortical
entrainment to music. _Proc. Natil Acad. Sci_. _USA_ 116, 10113–10121 (2019). * Heilbron, M., Armeni, K., Schoffelen, J.-M., Hagoort, P. & De Lange, F. P. A hierarchy of linguistic
predictions during natural language comprehension. _Proc. Natl Acad. Sci. USA_ 119, e2201968119 (2022). Article PubMed PubMed Central CAS Google Scholar * Nieuwland, M. S. et al.
Large-scale replication study reveals a limit on probabilistic prediction in language comprehension. _ELife_ 7, e33468 (2018). Article PubMed PubMed Central Google Scholar * Tezcan, F.,
Weissbart, H. & Martin, A. E. A tradeoff between acoustic and linguistic feature encoding in spoken language comprehension. _eLife_ 12, e82386 (2023). * Gwilliams, L., Marantz, A.,
Poeppel, D. & King, J.-R. Top-down information shapes lexical processing when listening to continuous speech. _Lang. Cogn. Neurosci._ 0, 1–14 (2023). Google Scholar * Hauser, M. D.,
Chomsky, N. & Fitch, W. T. The faculty of language: what is it, who has it, and how did it evolve? _Sci. (N. Y., N. Y.)_ 298, 1569–79 (2002). Article Google Scholar * Coopmans, C. W.,
De Hoop, H., Hagoort, P. & Martin, A. E. Effects of structure and meaning on cortical tracking of linguistic units in naturalistic speech. _Neurobiol. Lang._ 3, 386–412 (2022). Article
Google Scholar * Chomsky, N. _Aspects of the Theory of Syntax,_ Vol. 296 (MIT Press, 2014). * Sanford, A. J. & Sturt, P. Depth of processing in language comprehension: not noticing the
evidence. _Trends Cogn. Sci._ 6, 382–386 (2002). Article PubMed Google Scholar * Frank, S. L. & Bod, R. Insensitivity of the human sentence-processing system to hierarchical
tsructure. _Psychol. Sci._ 22, 21586764 (2011). Article Google Scholar * Brennan, J. R. & Hale, J. T. Hierarchical structure guides rapid linguistic predictions during naturalistic
listening. _PLoS ONE_ 14, e020774 (2019). * Martin, A. E. A compositional neural architecture for language. _J. Cogn. Neurosci._ 32, 1407–1427 (2020). Article PubMed Google Scholar * Oh,
B.-D., Clark, C. & Schuler, W. Comparison of structural parsers and neural language models as surprisal estimators. _Front. Artif. Intell._ 5, 2624–8212 (2022). Article Google Scholar
* Brennan, J. R., Stabler, E. P., Van Wagenen, S. E., Luh, W.-M. & Hale, J. T. Abstract linguistic structure correlates with temporal activity during naturalistic comprehension. _Brain
Lang._ 157-158, 81–94 (2016). Article PubMed PubMed Central Google Scholar * Ding, N., Melloni, L., Zhang, H., Tian, X. & Poeppel, D. Cortical tracking of hierarchical linguistic
structures in connected speech. _Nat. Neurosci._ 19, 158–164 (2016). Article PubMed CAS Google Scholar * Frank, S. L. & Yang, J. Lexical representation explains cortical entrainment
during speech comprehension. _PLoS ONE_ 13, e0197304 (2018). * Zaccarella, E., Schell, M. & Friederici, A. D. Reviewing the functional basis of the syntactic Merge mechanism for
language: a coordinate-based activation likelihood estimation meta-analysis. 80, 646–656 (2017). * Nelson, M. J. et al. Neurophysiological dynamics of phrase-structure building during
sentence processing. _Proc. Natl Acad. Sci. USA_ 114, E3669–E3678 (2017). * Hale, J. T. et al. Neurocomputational models of language processing. _Annu. Rev. Ling._ 8, 427–446 (2022). Article
Google Scholar * Lopopolo, A., van den Bosch, A., Petersson, K.-M. & Willems, R. M. Distinguishing dyntactic operations in the brain: dependency and phrase-structure parsing.
_Neurobiol. Lang._ 2, 152–175 (2021). Article Google Scholar * Zioga, I., Weissbart, H., Lewis, A. G., Haegens, S. & Martin, A. E. Naturalistic spoken language comprehension is
supported by alpha and beta oscillations. _J. Neurosci._ 43, 3718–3732 (2023). PubMed PubMed Central CAS Google Scholar * Elman, J. Finding structure in time. _Cogn. Sci._ 14, 179–211
(1990). Article Google Scholar * McCoy, R. T., Smolensky, P., Linzen, T., Gao, J. & Celikyilmaz, A. How much do language models copy from their training data? evaluating linguistic
novelty in text generation using RAVEN. _Trans. Assoc. Comput. Linguist._ 11, 652–670 (2023). Article Google Scholar * Mahowald, K. et al. Dissociating language and thought in large
language models: a cognitive perspective. _arXiv_ https://arxiv.org/abs/2301.06627 (2023). * Van Schijndel, M. & Linzen, T. Single-stage prediction models do not explain the magnitude of
syntactic disambiguation difficulty. _Cogn. Sci._ 45, e12988 (2021). Article PubMed Google Scholar * Antonello, R. & Huth, A. Predictive coding or just feature discovery? an
alternative account of why language models fit brain data. _Neurobiol. Lang._ 5, 64–79 (2022). * Jain, S., Vo, V. A., Wehbe, L. & Huth, A. G. Computational language modeling and the
promise of in silico experimentation. _Neurobiol. Lang._ 5, 80–106 (2023). * Hale, J. The information conveyed by words in sentences. _J. Psycholinguist. Res._ 32, 101–123 (2003). Article
PubMed Google Scholar * Weissbart, H., Kandylaki, K. D. & Reichenbach, T. Cortical tracking of surprisal during continuous speech comprehension. _J. Cogn. Neurosci._ 32, 155–166
(2020). Article PubMed Google Scholar * Karimi, H., Weber, P. & Zinn, J. Information entropy facilitates (not impedes) lexical processing during language comprehension. _Psychon.
Bull. Rev_. https://doi.org/10.3758/s13423-024-02463-x (2024). * Saffran, J. R., Aslin, R. N. & Newport, E. L. Statistical learning by 8 month-old infants. _Science_ 274, 1926–1928
(1996). Article ADS PubMed CAS Google Scholar * MacDonald, M. C., Pearlmutter, N. J. & Seidenberg, M. S. The lexical nature of syntactic ambiguity resolution. _Psychol. Rev._ 101,
676 (1994). Article PubMed CAS Google Scholar * Boorman, E. D., Sweigart, S. C. & Park, S. A. Cognitive maps and novel inferences: a flexibility hierarchy. _Curr. Opin. Behav. Sci._
38, 141–149 (2021). Article Google Scholar * Coopmans, C. W., De Hoop, H., Kaushik, K., Hagoort, P. & Martin, A. E. Hierarchy in language interpretation: evidence from behavioural
experiments and computational modelling. _Lang. Cogn. Neurosci._ 37, 420–439 (2022). Article Google Scholar * Coopmans, C. W., Kaushik, K. & Martin, A. E. Hierarchical structure in
language and action: a formal comparison. _Psychol. Rev._ 130, 935–952 (2023). * Peelle, J. E. & Davis, M. H. Neural oscillations carry speech rhythm through to comprehension. _Front.
Psychol._ 3, 320 (2012). Article PubMed PubMed Central Google Scholar * Giraud, A.-L. & Poeppel, D. Cortical oscillations and speech processing: emerging computational principles and
operations. _Nat. Neurosci._ 15, 511–517 (2012). Article PubMed PubMed Central CAS Google Scholar * Oganian, Y. et al. Phase alignment of low-frequency neural activity to the amplitude
envelope of speech reflects evoked responses to acoustic edges, not oscillatory entrainment. _J. Neurosci._ 43, 3909–3921 (2023). Article PubMed PubMed Central CAS Google Scholar *
Hyafil, A., Fontolan, L., Kabdebon, C., Gutkin, B. & Giraud, A.-L. Speech encoding by coupled cortical theta and gamma oscillations. _eLife_ 4, e06213 (2015). * Kaufeld, G. et al.
Linguistic structure and meaning organize neural oscillations into a content-specific hierarchy. _J. Neurosci._ 40, 9467–9475 (2020). Article PubMed PubMed Central CAS Google Scholar *
Kösem, A., van Wassenhove, V. & van Wassenhove, V. Distinct contributions of low- and high-frequency neural oscillations to speech comprehension. _Lang., Cogn. Neurosci._ 32, 536–544
(2017). Article Google Scholar * Lakatos, P., Karmos, G., Mehta, A. D., Ulbert, I. & Schroeder, C. E. Entrainment of neuronal oscillations as a mechanism of attentional selection.
_Science_ 320, 110–3 (2008). Article ADS PubMed CAS Google Scholar * Arnal, L. H., Doelling, K. B. & Poeppel, D. Delta-beta coupled oscillations underlie temporal prediction
accuracy. _Cereb. Cortex_ 25, 3077 (2015). Article PubMed Google Scholar * Ten Oever, S. & Martin, A. E. An oscillating computational model can track pseudo-rhythmic speech by using
linguistic predictions. _Elife_ 10, e68066 (2021). Article PubMed PubMed Central Google Scholar * Brennan, J. R., & Martin, A. E. Phase synchronization varies systematically with
linguistic structure composition. _Philos. Trans. R. Soc. B_ 375, 20190305 (2020). Article Google Scholar * Lisman, J. E. & Jensen, O. The theta-gamma neural code. _Neuron_ 77,
1002–1016 (2013). Article PubMed PubMed Central CAS Google Scholar * Murphy, E. ROSE: A neurocomputational architecture for syntax. _J. Neurolinguist._ 70, 101180 (2024). Article
Google Scholar * Zou, J. et al. _θ_-Band cortical tracking of the speech envelope shows the linear phase property. _eNeuro_ 8, ENEURO.0058-21.2021 (2021). * Brennan, J. R. & Pylkkänen,
L. MEG evidence for incremental sentence composition in the anterior temporal lobe. _Cogn. Sci._ 41, 1515–1531 (2017). Article PubMed Google Scholar * Donhauser, P. W. & Baillet, S.
Two distinct neural timescales for predictive speech processing. _Neuron_ 105, 385–393.e9 (2020). Article PubMed Google Scholar * Meyer, L., Sun, Y. & Martin, A. E. Synchronous, but
not entrained: exogenous and endogenous cortical rhythms of speech and language processing. _Lang., Cogn. Neurosci._ 35, 1089–1099 (2020). Article Google Scholar * Hovsepyan, S.,
Olasagasti, I. & Giraud, A.-L. Rhythmic modulation of prediction errors: a top-down gating role for the beta-range in speech processing. _PLOS Comput. Biol._ 19, 1–29 (2023). Article
Google Scholar * Etard, O. & Reichenbach, T. Neural speech tracking in the theta and in the delta frequency band differentially encode clarity and comprehension of speech in noise. _J.
Neurosci. Off. J. Soc. Neurosci._ 39, 5750–5759 (2019). Article CAS Google Scholar * Alamia, A. & VanRullen, R. A traveling waves perspective on temporal binding. _J. Cogn. Neurosci._
36, 721–729 (2024). Article PubMed Google Scholar * Morucci, P., Nara, S., Lizarazu, M., Martin, C. & Molinaro, N. Language experience shapes predictive coding of rhythmic sound
sequences. _eLife_ https://doi.org/10.1101/2023.04.28.538247 (2023). * Destrieux, C., Fischl, B., Dale, A. & Halgren, E. Automatic parcellation of human cortical gyri and sulci using
standard anatomical nomenclature. _NeuroImage_ 53, 1–15 (2010). Article PubMed Google Scholar * Pylkkänen, L. & Brennan, J. R. in _The Cognitive Neurosciences_ (eds. Gazzaniga, M.,
Mangun, G. & Poeppel, D.) 1152 (2019). * Bai, F., Meyer, A. S. & Martin, A. E. Neural dynamics differentially encode phrases and sentences during spoken language comprehension. _PLoS
Biol._ 20, e3001713 (2022). Article PubMed PubMed Central CAS Google Scholar * Slaats, S., Weissbart, H., Schoffelen, J.-M., Meyer, A. S. & Martin, A. E. Delta-band neural
responses to individual words are modulated by sentence processing. _J. Neurosci._ (2023). * Brodbeck, C. et al. Parallel processing in speech perception with local and global
representations of linguistic context. _eLife_ 11, e72056 (2022). * Molinaro, N., Barber, H. A. & Carreiras, M. Grammatical agreement processing in reading: ERP findings and future
directions. _Cortex_ 47, 908–930 (2011). Article PubMed Google Scholar * Kuperberg, G. R. Neural mechanisms of language comprehension: challenges to syntax. _Brain Res._ 1146, 23–49
(2007). Article PubMed CAS Google Scholar * Bastiaansen, M., Magyari, L. & Hagoort, P. Syntactic unification operations are reflected in oscillatory dynamics during on-line sentence
comprehension. en. _J. Cogn. Neurosci._ 22, 1333–1347 (2010). Article PubMed Google Scholar * Teng, X., Tian, X., Doelling, K. & Poeppel, D. Theta band oscillations reflect more than
entrainment: behavioral and neural evidence demonstrates an active chunking process. _Eur. J. Neurosci._ 48, 2770–2782 (2018). Article PubMed Google Scholar * Canolty, R. T. et al. High
gamma power is phase-locked to theta oscillations in human neocortex. _Science_ 313, 1626–1628 (2006). Article ADS PubMed PubMed Central CAS Google Scholar * Von der Malsburg, C. The
what and why of binding: the modeler’s perspective. _Neuron_ 24, 95–104 (1999). Article PubMed Google Scholar * Lewis, A. G., Schoffelen, J.-M., Hoffmann, C., Bastiaansen, M. &
Schriefers, H. Discourse-level semantic coherence influences beta oscillatory dynamics and the N400 during sentence comprehension. _Lang. Cogn. Neurosci._ 32, 601–617 (2017). Article Google
Scholar * Lewis, A. G., Wang, L. & Bastiaansen, M. Fast oscillatory dynamics during language comprehension: unification versus maintenance and prediction? _Brain Lang._ 148, 51–63
(2015). Article PubMed Google Scholar * Fontolan, L., Morillon, B., Liegeois-Chauvel, C. & Giraud, A.-L. The contribution of frequency-specific activity to hierarchical information
processing in the human auditory cortex. _Nat. Commun._ 5, 4694 (2014). Article ADS PubMed CAS Google Scholar * Deoisres, S., Lu, Y., Vanheusden, F. J., Bell, S. L. & Simpson, D. M.
Continuous speech with pauses inserted between words increases cortical tracking of speech envelope. _PLoS ONE_ 18, 1–21 (2023). Article Google Scholar * Spitzer, B. & Haegens, S.
Beyond the status quo: a role for beta oscillations in endogenous content (re) activation. _Eneuro_ 4, ENEURO.0170-17.2017 (2017). * Ding, N. & Pan, X. et al. Attention is required for
knowledge-based sequential grouping: insights from the integration of syllables into words. _J. Neurosci._ 38, 1178–1188 (2018). Article PubMed PubMed Central CAS Google Scholar *
Lewis, A. G., Schoffelen, J.-M., Schriefers, H. & Bastiaansen, M. A predictive coding perspective on beta oscillations during sentence-level language comprehension. _Front. Hum.
Neurosci_. https://doi.org/10.3389/fnhum.2016.00085 (2016). * Kleiner, M., Brainard, D. & Pelli, D. What’s new in psychtoolbox-3? _Perception_ 36, 1–16 (2007). * Van Veen, B., Van
Drongelen, W., Yuchtman, M. & Suzuki, A. Localization of brain electrical activity via linearly constrained minimum variance spatial filtering. _IEEE Trans. Biomed. Eng._ 44, 867–880
(1997). Article PubMed Google Scholar * Gramfort, A. et al. MEG and EEG data analysis with MNE-python. _Front. Neurosci._ 7, 1–13 (2013). Article Google Scholar * Crosse, M. J., Di
Liberto, G. M., Bednar, A. & Lalor, E. C. The multivariate temporal response function (mTRF) toolbox: a MATLAB toolbox for relating neural signals to continuous stimuli. _Front. Hum.
Neurosci._ 10, 604 (2016). * Haufe, S. et al. On the interpretation of weight vectors of linear models in multivariate neuroimaging. _NeuroImage_ 87, 96–110 (2014). Article PubMed Google
Scholar * Manning, C. D. et al. The Stanford CoreNLP natural language processing toolkit. in _Proc. 52nd Annual Meeting of the Association for Computational Linguistics: System
Demonstrations._ 55–60 (ACL, 2014). * Radford, A. et al. Language models are unsupervised multitask learners. _OpenAI Blog_ 1, 9 (2019). Google Scholar * Koelsch, S., Vuust, P. &
Friston, K. Predictive processes and the peculiar case of music. _Trends Cogn. Sci._ 23, 63–77 (2019). * Rasmussen, N. E. & Schuler, W. Left-corner parsing with distributed associative
memory produces surprisal and locality effects. _Cogn. Sci._ 42, 1009–1042 (2018). Article PubMed Google Scholar * Giglio, L., Ostarek, M., Sharoh, D. & Hagoort, P. Diverging neural
dynamics for syntactic structure building in naturalistic speaking and listening. _Proc. Natl Acad. Sci. USA_ 121, e2310766121 (2024). Article PubMed PubMed Central CAS Google Scholar *
Zuur, A. F., Ieno, E. N. & Elphick, C. S. A protocol for data exploration to avoid common statistical problems. _Methods Ecol. Evol._ 1, 3–14 (2010). Article Google Scholar *
Broderick, M. P., Anderson, A. J., Di Liberto, G. M., Crosse, M. J. & Lalor, E. C. Electrophysiological correlates of semantic dissimilarity reflect the comprehension of natural,
narrative speech. _Curr. Biol._ 28, 803–809.e3 (2018). Article PubMed Google Scholar * Combrisson Etienne amd Nest, T. toolbox for tensor-based phase-amplitude coupling measurement in
electrophysiological brain signals. _PLoS Comput. Biol._ 16, e1008302 (2020). Article Google Scholar * Forte, A. E., Etard, O. & Reichenbach, T. The human auditory brainstem response
to running speech reveals a subcortical mechanism for selective attention. _eLife_ 6, e27203 (Oct. 2017). * Oostenveld, R., Fries, P., Maris, E. & Schoffelen, J.-M. Cluster-based
permutation tests in neuroscience: theory, examples, and pitfalls. _NeuroImage_ 56, 908–916 (2011). Google Scholar * Martin, A. E. _Constructing Sentence-Level Meaning: An MEG Study of
Naturalistic Language Comprehension_ (Radboud University, 2023). * Weissbart, H. The structure and statistics of language jointly shape cross-frequency neural dynamics during spoken language
comprehension version v0.1.0. _Zenodo_ https://doi.org/10.5281/zenodo.12667684 (2024). Download references ACKNOWLEDGEMENTS A.E.M. was supported by an Independent Max Planck Research Group
and a Lise Meitner Research Group “Language and Computation in Neural Systems”, by NWO Vidi grant 016.Vidi.188.029 to AEM, and by Big Question 5 (to Prof. dr. Roshan Cools & Dr. Andrea
E. Martin) of the Language in Interaction Consortium funded by NWO Gravitation Grant 024.001.006 to Prof. dr. Peter Hagoort. Hugo Weissbart was supported by NWO Vidi grant 016.Vidi.188.029
to AEM. We thank the members of the Language and Computation in Neural Systems Group for assistance and support in the form of team data collection and discussion of our results. FUNDING
Open Access funding enabled and organized by Projekt DEAL. AUTHOR INFORMATION AUTHORS AND AFFILIATIONS * Donders Centre for Cognitive Neuroimaging, Radboud University, Nijmegen, The
Netherlands Hugo Weissbart & Andrea E. Martin * Max Planck Institute for Psycholinguistics, Nijmegen, The Netherlands Hugo Weissbart & Andrea E. Martin Authors * Hugo Weissbart View
author publications You can also search for this author inPubMed Google Scholar * Andrea E. Martin View author publications You can also search for this author inPubMed Google Scholar
CONTRIBUTIONS H.W. and A.E.M. conceptualized the study. H.W. designed and implemented the experiment, collected and preprocessed the data; developed the code and analysis of the data. H.W.
and A.E.M. wrote, reviewed and edited the manuscript. CORRESPONDING AUTHOR Correspondence to Hugo Weissbart. ETHICS DECLARATIONS COMPETING INTERESTS The authors declare no competing
interests. PEER REVIEW PEER REVIEW INFORMATION _Nature Communications_ thanks Anastasia Klimovich-Gray, Benedikt Zoefel and the other, anonymous, reviewer(s) for their contribution to the
peer review of this work. A peer review file is available. ADDITIONAL INFORMATION PUBLISHER’S NOTE Springer Nature remains neutral with regard to jurisdictional claims in published maps and
institutional affiliations. SUPPLEMENTARY INFORMATION SUPPLEMENTARY INFORMATION PEER REVIEW FILE REPORTING SUMMARY RIGHTS AND PERMISSIONS OPEN ACCESS This article is licensed under a
Creative Commons Attribution 4.0 International License, which permits use, sharing, adaptation, distribution and reproduction in any medium or format, as long as you give appropriate credit
to the original author(s) and the source, provide a link to the Creative Commons licence, and indicate if changes were made. The images or other third party material in this article are
included in the article’s Creative Commons licence, unless indicated otherwise in a credit line to the material. If material is not included in the article’s Creative Commons licence and
your intended use is not permitted by statutory regulation or exceeds the permitted use, you will need to obtain permission directly from the copyright holder. To view a copy of this
licence, visit http://creativecommons.org/licenses/by/4.0/. Reprints and permissions ABOUT THIS ARTICLE CITE THIS ARTICLE Weissbart, H., Martin, A.E. The structure and statistics of language
jointly shape cross-frequency neural dynamics during spoken language comprehension. _Nat Commun_ 15, 8850 (2024). https://doi.org/10.1038/s41467-024-53128-1 Download citation * Received: 18
October 2023 * Accepted: 30 September 2024 * Published: 14 October 2024 * DOI: https://doi.org/10.1038/s41467-024-53128-1 SHARE THIS ARTICLE Anyone you share the following link with will be
able to read this content: Get shareable link Sorry, a shareable link is not currently available for this article. Copy to clipboard Provided by the Springer Nature SharedIt content-sharing
initiative