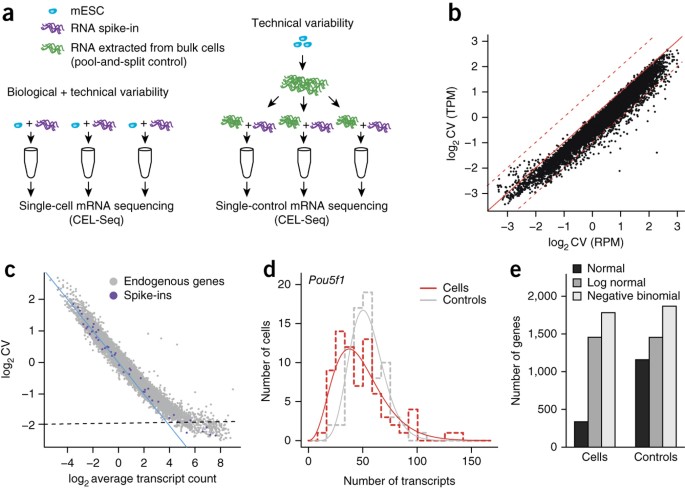
- Select a language for the TTS:
- UK English Female
- UK English Male
- US English Female
- US English Male
- Australian Female
- Australian Male
- Language selected: (auto detect) - EN
Play all audios:
ABSTRACT Single-cell transcriptomics has recently emerged as a powerful technology to explore gene expression heterogeneity among single cells. Here we identify two major sources of
technical variability: sampling noise and global cell-to-cell variation in sequencing efficiency. We propose noise models to correct for this, which we validate using single-molecule FISH.
We demonstrate that gene expression variability in mouse embryonic stem cells depends on the culture condition. Access through your institution Buy or subscribe This is a preview of
subscription content, access via your institution ACCESS OPTIONS Access through your institution Subscribe to this journal Receive 12 print issues and online access $259.00 per year only
$21.58 per issue Learn more Buy this article * Purchase on SpringerLink * Instant access to full article PDF Buy now Prices may be subject to local taxes which are calculated during checkout
ADDITIONAL ACCESS OPTIONS: * Log in * Learn about institutional subscriptions * Read our FAQs * Contact customer support SIMILAR CONTENT BEING VIEWED BY OTHERS SCPOWER ACCELERATES AND
OPTIMIZES THE DESIGN OF MULTI-SAMPLE SINGLE CELL TRANSCRIPTOMIC STUDIES Article Open access 16 November 2021 MARKERMAP: NONLINEAR MARKER SELECTION FOR SINGLE-CELL STUDIES Article Open access
14 February 2024 SEPARATING MEASUREMENT AND EXPRESSION MODELS CLARIFIES CONFUSION IN SINGLE-CELL RNA SEQUENCING ANALYSIS Article 24 May 2021 ACCESSION CODES PRIMARY ACCESSIONS GENE
EXPRESSION OMNIBUS * GSE54695 REFERENCES * Munsky, B., Neuert, G. & van Oudenaarden, A. _Science_ 336, 183–187 (2012). Article CAS Google Scholar * Eldar, A. & Elowitz, M.B.
_Nature_ 467, 167–173 (2010). Article CAS Google Scholar * Hashimshony, T., Wagner, F., Sher, N. & Yanai, I. _Cell Rep._ 2, 666–673 (2012). Article CAS Google Scholar * Sasagawa,
Y. et al. _Genome Biol._ 14, R31 (2013). Article Google Scholar * Tang, F. et al. _Nat. Methods_ 6, 377–382 (2009). Article CAS Google Scholar * Ramsköld, D. et al. _Nat. Biotechnol._
30, 777–782 (2012). Article Google Scholar * Islam, S. et al. _Genome Res._ 21, 1160–1167 (2011). Article CAS Google Scholar * Picelli, S. et al. _Nat. Methods_ 10, 1096–1098 (2013).
Article CAS Google Scholar * Shapiro, E., Biezuner, T. & Linnarsson, S. _Nat. Rev. Genet._ 14, 618–630 (2013). Article CAS Google Scholar * Kivioja, T. et al. _Nat. Methods_ 9,
72–74 (2012). Article CAS Google Scholar * Shiroguchi, K., Jia, T.Z., Sims, P.A. & Xie, X.S. _Proc. Natl. Acad. Sci. USA_ 109, 1347–1352 (2012). Article CAS Google Scholar * Hug,
H. & Schuler, R. _J. Theor. Biol._ 221, 615–624 (2003). Article CAS Google Scholar * Shalek, A.K. et al. _Nature_ 498, 236–240 (2013). Article CAS Google Scholar * Islam, S. et al.
_Nat. Methods_ 11, 163–166 (2014). Article CAS Google Scholar * Jaitin, D.A. et al. _Science_ 343, 776–779 (2014). Article CAS Google Scholar * Brennecke, P. et al. _Nat. Methods_ 10,
1093–1095 (2013). Article CAS Google Scholar * Ying, Q.-L. et al. _Nature_ 453, 519–523 (2008). Article CAS Google Scholar * The External RNA Controls Consortium. _Nat. Methods_ 2,
731–734 (2005). * Raj, A., Peskin, C.S., Tranchina, D., Vargas, D.Y. & Tyagi, S. _PLoS Biol._ 4, e309 (2006). Article Google Scholar * Raj, A., van den Bogaard, P., Rifkin, S.A., van
Oudenaarden, A. & Tyagi, S. _Nat. Methods_ 5, 877–879 (2008). Article CAS Google Scholar * Li, H. & Durbin, R. _Bioinformatics_ 26, 589–595 (2010). Article Google Scholar *
Meyer, L.R. et al. _Nucleic Acids Res._ 41, D64–D69 (2013). Article CAS Google Scholar * Anders, S. & Huber, W. _Genome Biol._ 11, R106 (2010). Article CAS Google Scholar *
Robinson, M.D., McCarthy, D.J. & Smyth, G.K. _Bioinformatics_ 26, 139–140 (2010). Article CAS Google Scholar * Byrd, R.H., Lu, P., Nocedal, J. & Zhu, C. _SIAM J. Sci. Comput._ 16,
1190–1208 (1995). Article Google Scholar Download references ACKNOWLEDGEMENTS This work was supported by a European Research Council Advanced grant (ERC-AdG 294325-GeneNoiseControl) and a
Nederlandse Organisatie voor Wetenschappelijk Onderzoek (NWO) Vici award. AUTHOR INFORMATION Author notes * Dominic Grün and Lennart Kester: These authors contributed equally to this work.
AUTHORS AND AFFILIATIONS * Hubrecht Institute-KNAW (Royal Netherlands Academy of Arts and Sciences), Utrecht, The Netherlands Dominic Grün, Lennart Kester & Alexander van Oudenaarden *
University Medical Center Utrecht, Cancer Genomics Netherlands, Utrecht, The Netherlands Dominic Grün, Lennart Kester & Alexander van Oudenaarden Authors * Dominic Grün View author
publications You can also search for this author inPubMed Google Scholar * Lennart Kester View author publications You can also search for this author inPubMed Google Scholar * Alexander van
Oudenaarden View author publications You can also search for this author inPubMed Google Scholar CONTRIBUTIONS D.G., L.K. and A.v.O. conceived the methods. D.G. developed the noise models,
performed all computations and wrote the manuscript. L.K. performed all experiments and corrected the manuscript. A.v.O. guided experiments, data analysis and writing of the manuscript, and
corrected the manuscript. CORRESPONDING AUTHOR Correspondence to Alexander van Oudenaarden. ETHICS DECLARATIONS COMPETING INTERESTS The authors declare no competing financial interests.
SUPPLEMENTARY INFORMATION SUPPLEMENTARY TEXT AND FIGURES Supplementary Figures 1–15, Supplementary Table 1 and Supplementary Notes 1–4. (PDF 11686 kb) SUPPLEMENTARY TABLE 2 GO terms enriched
among genes with increased expression variability in serum versus 2i culture condition. Enriched biological processes and enriched molecular functions are given as separate lists. Only
significantly enriched GO-terms (_P_ < 0.05) were included. The lists indicate the GO-term ID, the hypergeometric P-value, the odds ratio, the expected number of genes associated with
each GO-term, the observed number of genes for each GO-term, the size of the GO-term (total number of genes associated) and a short description. For the inference of over-represented GO
terms, the set of differentially variable genes was compared to the universe of all genes expressed in the two conditions. The GOstats package was used to compute GO enrichment in R. (XLSX
82 kb) SUPPLEMENTARY TABLE 3 Probe set composition of smFISH probes used. Each column represents a probe set for the gene specified in the column header. All probes were labeled on the
3' end with TMR, Alexa594 or Cy5. (XLSX 56 kb) RIGHTS AND PERMISSIONS Reprints and permissions ABOUT THIS ARTICLE CITE THIS ARTICLE Grün, D., Kester, L. & van Oudenaarden, A.
Validation of noise models for single-cell transcriptomics. _Nat Methods_ 11, 637–640 (2014). https://doi.org/10.1038/nmeth.2930 Download citation * Received: 16 October 2013 * Accepted: 25
March 2014 * Published: 20 April 2014 * Issue Date: June 2014 * DOI: https://doi.org/10.1038/nmeth.2930 SHARE THIS ARTICLE Anyone you share the following link with will be able to read this
content: Get shareable link Sorry, a shareable link is not currently available for this article. Copy to clipboard Provided by the Springer Nature SharedIt content-sharing initiative