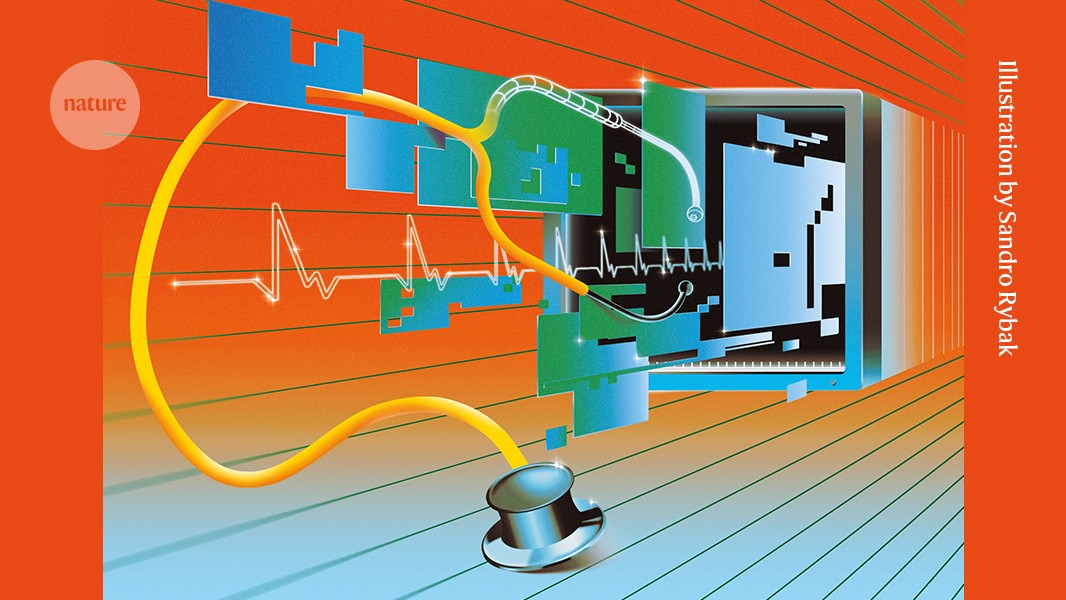
- Select a language for the TTS:
- UK English Female
- UK English Male
- US English Female
- US English Male
- Australian Female
- Australian Male
- Language selected: (auto detect) - EN
Play all audios:
When Devin Singh was a paediatric resident, he attended to a young child who had gone into cardiac arrest in the emergency department after a prolonged wait to see a doctor. “I remember
doing CPR on this patient and feeling that kiddo slip away,” he says. Devastated by the child’s death, Singh remembers wondering whether a shorter waiting time could have prevented it. The
incident convinced him to combine his paediatric expertise with his other speciality — computer science — to see whether artificial intelligence (AI) might help to cut waiting times. Using
emergency-department triage data from the Hospital for Sick Children (SickKids) in Toronto, Canada, where Singh currently works, he and his colleagues built a collection of AI models that
provide potential diagnoses and indicate which tests will probably be required. “If we can predict, for example, that a patient has a high likelihood of appendicitis and needs an abdominal
ultrasound, we can automate ordering that test almost instantly after a patient arrives, rather than having them wait 6–10 hours to see a doctor,” he says. A study using retrospective data
from more than 77,000 emergency-department visits to SickKids suggested that these models would expedite care for 22.3% of visits, speeding up results by nearly 3 hours for each person
requiring medical tests1. The success of an AI algorithm in a study such as this, however, is only the first step in verifying whether such an intervention would help people in real life.
Ex-Meta scientists debut gigantic AI protein design model Properly testing AI systems for use in a medical setting is a complex multiphase process. But relatively few developers are
publishing the results of such analyses. Only 65 randomized controlled trials of AI interventions were published between 2020 and 2022, a review shows2. Meanwhile, regulators such as the US
Food and Drug Administration (FDA) have approved hundreds of AI-powered medical devices for use in hospitals and clinics. “Health-care organizations are seeing many approved devices that
don’t have clinical validation,” says David Ouyang, a cardiologist at Cedars-Sinai Medical Center in Los Angeles, California. Some hospitals opt to test such equipment themselves. And
although researchers know what an ideal clinical trial for an AI-based intervention should look like3, in practice, testing these technologies is challenging. Implementation depends on how
well health-care professionals interact with the algorithms: a perfectly good tool will fail if humans ignore its suggestions. AI programs can be particularly sensitive to differences
between the populations whose data they were trained on and the ones they’re aiming to help. Moreover, it’s not yet clear how best to inform patients and their families about these
technologies and ask for their consent to use their data for testing the devices. Some hospitals and health-care systems are experimenting with ways to use and evaluate AI systems in
medicine. And as more AI tools and companies are entering the market, groups are coming together to seek consensus on what kinds of assessment work best and provide the most rigour. WHO IS
TESTING MEDICAL AI SYSTEMS? AI-based medical applications, such as the one being built by Singh, are generally considered medical devices by drug regulators, including the US FDA and the UK
Medicines and Healthcare products Regulatory Agency. As such, the criteria for reviewing and authorizing them for use are often less rigorous than are those for drugs. Only a small
proportion of devices — those that might pose a high risk to patients — require clinical-trial data for approval. Many think that the bar is too low. When Gary Weissman, a critical-care
physician at the University of Pennsylvania in Philadelphia, reviewed the FDA-approved AI devices in his field, he found that, of the ten he identified, only three cited published data in
their authorizations. Just four mentioned a safety assessment and none included a bias evaluation, which analyses whether the tool’s outcomes are fair across different patient groups4.
“What’s concerning is these devices really can and do influence care at the bedside,” he says. “A patient’s life can hinge on those decisions.” The dearth of data leaves hospitals and
health-care systems in a difficult position when deciding whether to use these technologies. In some cases, financial incentives come into play. In the United States, for example,
health-insurance programmes already reimburse hospitals for the use of certain medical AI devices5, making them economically appealing. These institutions might also be inclined to adopt AI
tools that promise cost savings, even if they don’t necessarily improve patient care. Those incentives could discourage AI companies from investing in clinical trials, says Ouyang. “For many
commercial enterprises, you can imagine they’re putting more effort in making sure their AI tool is reimbursable and has a good financial outcome, because they see that that drives
adoption,” he says. An AI revolution is brewing in medicine. What will it look like? The situation might be different depending on the market. In the United Kingdom, for example, nationwide
government-sponsored health programmes might set a higher evidence bar before medical centres can acquire a given product, says Xiaoxuan Liu, a clinical researcher who studies responsible
innovation in AI at the University of Birmingham, UK. “Then, the incentive is there for companies to do clinical trials,” says Liu. Once hospitals purchase an AI product, they are not
required to perform further tests and can use it immediately as they would any other software. Some institutions, however, recognize that regulatory approval does not guarantee that the
device is truly beneficial. So they choose to test it themselves. Many of these efforts are currently performed and funded by academic medical centres, Ouyang says. Alexander Vlaar, the head
of intensive-care medicine at Amsterdam University Medical Center, and Denise Veelo, an anaesthesiologist at the same institution, started one such endeavour in 2017. Their goal was to test
an algorithm that aims to predict the occurrence of low blood pressure during surgery. This condition, known as intraoperative hypotension, can lead to life-threatening complications, such
as myocardial injury, heart attack and acute renal failure, and even death. The algorithm was developed by Edwards Lifesciences, a company in Irvine, California, and uses arterial waveform
data — the red line with peaks and troughs seen on monitors in an emergency department or intensive-care unit. It can predict hypotension minutes before it happens, enabling early
intervention. Vlaar, Veelo and their colleagues conducted a randomized clinical trial to test the tool on 60 patients undergoing non-cardiac surgery. Individuals who had the device running
during their surgery experienced a median time of 8 minutes of hypotension compared with nearly 33 minutes for those in the control group6. The team ran a second clinical trial, which
confirmed that the device, combined with a clear treatment protocol, also works in more-complex settings, including during cardiac surgery and in the intensive-care unit. The results have
not yet been published. The success wasn’t simply because of the precision of the algorithm. How the anaesthesiologists respond to an alert matters. So, the researchers made sure to prepare
physicians carefully: “We had a diagnostic flowchart with steps to take when you get an alarm,” says Veelo. The same algorithm failed to show a benefit in a clinical trial performed by
another institution7. In that case, “there was no compliance by the bedside physicians for doing something when the alarm went off”, says Vlaar. THE HUMANS IN THE LOOP A perfectly good
algorithm might fail because of variability in human behaviour, both by health-care professionals and by people receiving treatments. When Mayo Clinic in Rochester, Minnesota, tested an
algorithm developed in-house to detect a heart condition called low ejection fraction, the centre’s human–computer interaction researcher, Barbara Barry, was in charge of bridging the gap
between developers and the primary-care providers using the technology. AI tools are designing entirely new proteins that could transform medicine The tool was designed to flag individuals
who might be at high risk of the condition, which can be a sign of heart failure and is treatable, but often goes undiagnosed. A clinical trial showed that the algorithm did increase
diagnosis8. However, in conversations with providers, Barry found that they wanted further guidance on how to talk to the patients about the algorithm’s findings. This led to the
recommendation that the application, if widely implemented, should include bullet points with important information to communicate to the patient so that the health-care provider doesn’t
have to consider how to have that conversation each time. “This is one example of how we move from a pragmatic trial to implementation strategies,” Barry says. Another issue that can limit
the success of certain medical AI devices is ‘alert fatigue’ — when clinicians are exposed to a high number of AI-generated warnings, they might become desensitized to them. This should be
considered during the testing process, says David Rushlow, chair of the family medicine department at Mayo Clinic. “We’re already getting alerted many times a day on conditions that our
patients may be at risk for. And that’s actually a very difficult task for a busy front-line clinician,” he says. “I think many of these tools will be able to help us. But, if they are not
introduced accurately, the default will be to just continue to do things the same way, because we don’t have the bandwidth to learn something new,” Rushlow notes. CONSIDERING BIAS Another
challenge in testing medical AI is that clinical-trial results are hard to generalize to different population groups. “It’s simply a known fact that AI algorithms are very fragile when they
are used on data that is different from the data that it was trained on,” Liu says. Results can be extrapolated safely only if the clinical-trial participants are representative of the
population the tool will be used in, she notes. ENJOYING OUR LATEST CONTENT? LOGIN OR CREATE AN ACCOUNT TO CONTINUE * Access the most recent journalism from Nature's award-winning team
* Explore the latest features & opinion covering groundbreaking research Access through your institution or Sign in or create an account Continue with Google Continue with ORCiD